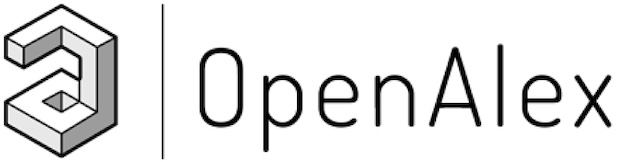
OpenAlex is a bibliographic catalogue of scientific papers, authors and institutions accessible in open access mode, named after the Library of Alexandria. It's citation coverage is excellent and I hope you will find utility in this listing of citing articles!
If you click the article title, you'll navigate to the article, as listed in CrossRef. If you click the Open Access links, you'll navigate to the "best Open Access location". Clicking the citation count will open this listing for that article. Lastly at the bottom of the page, you'll find basic pagination options.
Requested Article:
General Pitfalls of Model-Agnostic Interpretation Methods for Machine Learning Models
Christoph Molnar, Gunnar König, Julia Herbinger, et al.
Lecture notes in computer science (2022), pp. 39-68
Open Access | Times Cited: 93
Christoph Molnar, Gunnar König, Julia Herbinger, et al.
Lecture notes in computer science (2022), pp. 39-68
Open Access | Times Cited: 93
Showing 1-25 of 93 citing articles:
Machine learning in concrete science: applications, challenges, and best practices
Zhanzhao Li, Jinyoung Yoon, Rui Zhang, et al.
npj Computational Materials (2022) Vol. 8, Iss. 1
Open Access | Times Cited: 188
Zhanzhao Li, Jinyoung Yoon, Rui Zhang, et al.
npj Computational Materials (2022) Vol. 8, Iss. 1
Open Access | Times Cited: 188
A Perspective on Explainable Artificial Intelligence Methods: SHAP and LIME
Ahmed Salih, Zahra Raisi‐Estabragh, Ilaria Boscolo Galazzo, et al.
Advanced Intelligent Systems (2024)
Open Access | Times Cited: 58
Ahmed Salih, Zahra Raisi‐Estabragh, Ilaria Boscolo Galazzo, et al.
Advanced Intelligent Systems (2024)
Open Access | Times Cited: 58
On the importance of interpretable machine learning predictions to inform clinical decision making in oncology
Sheng-Chieh Lu, Christine L. Swisher, Caroline Chung, et al.
Frontiers in Oncology (2023) Vol. 13
Open Access | Times Cited: 47
Sheng-Chieh Lu, Christine L. Swisher, Caroline Chung, et al.
Frontiers in Oncology (2023) Vol. 13
Open Access | Times Cited: 47
How Interpretable Machine Learning Can Benefit Process Understanding in the Geosciences
Shijie Jiang, Lily‐belle Sweet, Georgios Blougouras, et al.
Earth s Future (2024) Vol. 12, Iss. 7
Open Access | Times Cited: 27
Shijie Jiang, Lily‐belle Sweet, Georgios Blougouras, et al.
Earth s Future (2024) Vol. 12, Iss. 7
Open Access | Times Cited: 27
Interpretable Machine Learning Techniques in ECG-Based Heart Disease Classification: A Systematic Review
Yehualashet Megersa Ayano, Friedhelm Schwenker, Bisrat Derebssa Dufera, et al.
Diagnostics (2022) Vol. 13, Iss. 1, pp. 111-111
Open Access | Times Cited: 60
Yehualashet Megersa Ayano, Friedhelm Schwenker, Bisrat Derebssa Dufera, et al.
Diagnostics (2022) Vol. 13, Iss. 1, pp. 111-111
Open Access | Times Cited: 60
Beyond prediction: methods for interpreting complex models of soil variation
Alexandre M.J.‐C. Wadoux, Christoph Molnar
Geoderma (2022) Vol. 422, pp. 115953-115953
Open Access | Times Cited: 44
Alexandre M.J.‐C. Wadoux, Christoph Molnar
Geoderma (2022) Vol. 422, pp. 115953-115953
Open Access | Times Cited: 44
Using SHAP Values and Machine Learning to Understand Trends in the Transient Stability Limit
R. I. Hamilton, Panagiotis N. Papadopoulos
IEEE Transactions on Power Systems (2023) Vol. 39, Iss. 1, pp. 1384-1397
Open Access | Times Cited: 38
R. I. Hamilton, Panagiotis N. Papadopoulos
IEEE Transactions on Power Systems (2023) Vol. 39, Iss. 1, pp. 1384-1397
Open Access | Times Cited: 38
Relating the Partial Dependence Plot and Permutation Feature Importance to the Data Generating Process
Christoph Molnar, Timo Freiesleben, Gunnar König, et al.
Communications in computer and information science (2023), pp. 456-479
Open Access | Times Cited: 36
Christoph Molnar, Timo Freiesleben, Gunnar König, et al.
Communications in computer and information science (2023), pp. 456-479
Open Access | Times Cited: 36
Interpretable Dropout Prediction: Towards XAI-Based Personalized Intervention
Marcell Nagy, Roland Molontay
International Journal of Artificial Intelligence in Education (2023) Vol. 34, Iss. 2, pp. 274-300
Open Access | Times Cited: 34
Marcell Nagy, Roland Molontay
International Journal of Artificial Intelligence in Education (2023) Vol. 34, Iss. 2, pp. 274-300
Open Access | Times Cited: 34
Interpretable machine learning for psychological research: Opportunities and pitfalls.
Mirka Henninger, Rudolf Debelak, Yannick Rothacher, et al.
Psychological Methods (2023)
Open Access | Times Cited: 30
Mirka Henninger, Rudolf Debelak, Yannick Rothacher, et al.
Psychological Methods (2023)
Open Access | Times Cited: 30
Machine learning for an explainable cost prediction of medical insurance
Ugochukwu Orji, Elochukwu Ukwandu
Machine Learning with Applications (2023) Vol. 15, pp. 100516-100516
Open Access | Times Cited: 23
Ugochukwu Orji, Elochukwu Ukwandu
Machine Learning with Applications (2023) Vol. 15, pp. 100516-100516
Open Access | Times Cited: 23
Can Fairness be Automated? Guidelines and Opportunities for Fairness-aware AutoML
Hilde Weerts, Florian Pfisterer, Matthias Feurer, et al.
Journal of Artificial Intelligence Research (2024) Vol. 79, pp. 639-677
Open Access | Times Cited: 8
Hilde Weerts, Florian Pfisterer, Matthias Feurer, et al.
Journal of Artificial Intelligence Research (2024) Vol. 79, pp. 639-677
Open Access | Times Cited: 8
Learning and actioning general principles of cancer cell drug sensitivity
Francesco Carli, Pierluigi Di Chiaro, Mariangela Morelli, et al.
Nature Communications (2025) Vol. 16, Iss. 1
Open Access | Times Cited: 1
Francesco Carli, Pierluigi Di Chiaro, Mariangela Morelli, et al.
Nature Communications (2025) Vol. 16, Iss. 1
Open Access | Times Cited: 1
Pretrained transformers applied to clinical studies improve predictions of treatment efficacy and associated biomarkers
Gustavo Arango-Argoty, Elly Kipkogei, Ross Stewart, et al.
Nature Communications (2025) Vol. 16, Iss. 1
Open Access | Times Cited: 1
Gustavo Arango-Argoty, Elly Kipkogei, Ross Stewart, et al.
Nature Communications (2025) Vol. 16, Iss. 1
Open Access | Times Cited: 1
Explainable AI In Education : Current Trends, Challenges, And Opportunities
Ashwin Rachha, Mohammed Seyam
SoutheastCon (2023)
Closed Access | Times Cited: 21
Ashwin Rachha, Mohammed Seyam
SoutheastCon (2023)
Closed Access | Times Cited: 21
A Guide to Feature Importance Methods for Scientific Inference
Fiona Katharina Ewald, Ludwig Bothmann, Marvin N. Wright, et al.
Communications in computer and information science (2024), pp. 440-464
Open Access | Times Cited: 6
Fiona Katharina Ewald, Ludwig Bothmann, Marvin N. Wright, et al.
Communications in computer and information science (2024), pp. 440-464
Open Access | Times Cited: 6
Machine learning methods for prediction of cancer driver genes: a survey paper
Renan Soares de Andrades, Mariana Recamonde‐Mendoza
Briefings in Bioinformatics (2022) Vol. 23, Iss. 3
Open Access | Times Cited: 27
Renan Soares de Andrades, Mariana Recamonde‐Mendoza
Briefings in Bioinformatics (2022) Vol. 23, Iss. 3
Open Access | Times Cited: 27
Explaining a Deep Reinforcement Learning (DRL)-Based Automated Driving Agent in Highway Simulations
Francesco Bellotti, Luca Lazzaroni, Alessio Capello, et al.
IEEE Access (2023) Vol. 11, pp. 28522-28550
Open Access | Times Cited: 13
Francesco Bellotti, Luca Lazzaroni, Alessio Capello, et al.
IEEE Access (2023) Vol. 11, pp. 28522-28550
Open Access | Times Cited: 13
The Blame Problem in Evaluating Local Explanations and How to Tackle It
Amir Hossein Akhavan Rahnama
Communications in computer and information science (2024), pp. 66-86
Closed Access | Times Cited: 5
Amir Hossein Akhavan Rahnama
Communications in computer and information science (2024), pp. 66-86
Closed Access | Times Cited: 5
Bankruptcy prediction using machine learning and Shapley additive explanations
Hoang Hiep Nguyen, Jean‐Laurent Viviani, Sami Ben Jabeur
Review of Quantitative Finance and Accounting (2023)
Closed Access | Times Cited: 11
Hoang Hiep Nguyen, Jean‐Laurent Viviani, Sami Ben Jabeur
Review of Quantitative Finance and Accounting (2023)
Closed Access | Times Cited: 11
Variable Importance in High-Dimensional Settings Requires Grouping
Ahmad Chamma, Bertrand Thirion, Denis A. Engemann
Proceedings of the AAAI Conference on Artificial Intelligence (2024) Vol. 38, Iss. 10, pp. 11195-11203
Open Access | Times Cited: 4
Ahmad Chamma, Bertrand Thirion, Denis A. Engemann
Proceedings of the AAAI Conference on Artificial Intelligence (2024) Vol. 38, Iss. 10, pp. 11195-11203
Open Access | Times Cited: 4
Characterizing climate pathways using feature importance on echo state networks
Katherine Goode, Daniel Ries, Kellie McClernon
Statistical Analysis and Data Mining The ASA Data Science Journal (2024) Vol. 17, Iss. 4
Open Access | Times Cited: 4
Katherine Goode, Daniel Ries, Kellie McClernon
Statistical Analysis and Data Mining The ASA Data Science Journal (2024) Vol. 17, Iss. 4
Open Access | Times Cited: 4
A review of evaluation approaches for explainable AI with applications in cardiology
Ahmed Salih, Ilaria Boscolo Galazzo, Polyxeni Gkontra, et al.
Artificial Intelligence Review (2024) Vol. 57, Iss. 9
Open Access | Times Cited: 4
Ahmed Salih, Ilaria Boscolo Galazzo, Polyxeni Gkontra, et al.
Artificial Intelligence Review (2024) Vol. 57, Iss. 9
Open Access | Times Cited: 4
Beyond the black box with biologically informed neural networks
David Selby, Maximilian Sprang, Jan Ewald, et al.
Nature Reviews Genetics (2025)
Closed Access
David Selby, Maximilian Sprang, Jan Ewald, et al.
Nature Reviews Genetics (2025)
Closed Access
An urgent call for robust statistical methods in reliable feature importance analysis across machine learning
Yoshiyasu Takefuji
Journal of Catalysis (2025), pp. 116098-116098
Closed Access
Yoshiyasu Takefuji
Journal of Catalysis (2025), pp. 116098-116098
Closed Access