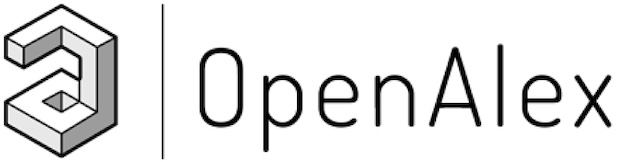
OpenAlex is a bibliographic catalogue of scientific papers, authors and institutions accessible in open access mode, named after the Library of Alexandria. It's citation coverage is excellent and I hope you will find utility in this listing of citing articles!
If you click the article title, you'll navigate to the article, as listed in CrossRef. If you click the Open Access links, you'll navigate to the "best Open Access location". Clicking the citation count will open this listing for that article. Lastly at the bottom of the page, you'll find basic pagination options.
Requested Article:
Predicting suicides after outpatient mental health visits in the Army Study to Assess Risk and Resilience in Servicemembers (Army STARRS)
Ronald C. Kessler, Murray B. Stein, Maria Petukhova, et al.
Molecular Psychiatry (2016) Vol. 22, Iss. 4, pp. 544-551
Open Access | Times Cited: 150
Ronald C. Kessler, Murray B. Stein, Maria Petukhova, et al.
Molecular Psychiatry (2016) Vol. 22, Iss. 4, pp. 544-551
Open Access | Times Cited: 150
Showing 26-50 of 150 citing articles:
Consensus Statement on Ethical & Safety Practices for Conducting Digital Monitoring Studies with People at Risk of Suicide and Related Behaviors
Matthew K. Nock, Evan M. Kleiman, Melissa Abraham, et al.
Psychiatric Research and Clinical Practice (2020) Vol. 3, Iss. 2, pp. 57-66
Open Access | Times Cited: 61
Matthew K. Nock, Evan M. Kleiman, Melissa Abraham, et al.
Psychiatric Research and Clinical Practice (2020) Vol. 3, Iss. 2, pp. 57-66
Open Access | Times Cited: 61
Prospective Validation of an Electronic Health Record–Based, Real-Time Suicide Risk Model
Colin G. Walsh, Kevin B. Johnson, Michael Ripperger, et al.
JAMA Network Open (2021) Vol. 4, Iss. 3, pp. e211428-e211428
Open Access | Times Cited: 55
Colin G. Walsh, Kevin B. Johnson, Michael Ripperger, et al.
JAMA Network Open (2021) Vol. 4, Iss. 3, pp. e211428-e211428
Open Access | Times Cited: 55
Can machine-learning methods really help predict suicide?
Catherine McHugh, Matthew Large
Current Opinion in Psychiatry (2020) Vol. 33, Iss. 4, pp. 369-374
Closed Access | Times Cited: 51
Catherine McHugh, Matthew Large
Current Opinion in Psychiatry (2020) Vol. 33, Iss. 4, pp. 369-374
Closed Access | Times Cited: 51
Applying Machine Learning Approaches to Suicide Prediction Using Healthcare Data: Overview and Future Directions
Edwin D. Boudreaux, Elke A. Rundensteiner, Feifan Liu, et al.
Frontiers in Psychiatry (2021) Vol. 12
Open Access | Times Cited: 45
Edwin D. Boudreaux, Elke A. Rundensteiner, Feifan Liu, et al.
Frontiers in Psychiatry (2021) Vol. 12
Open Access | Times Cited: 45
Integration of Face-to-Face Screening With Real-time Machine Learning to Predict Risk of Suicide Among Adults
Drew Wilimitis, Robert W. Turer, Michael Ripperger, et al.
JAMA Network Open (2022) Vol. 5, Iss. 5, pp. e2212095-e2212095
Open Access | Times Cited: 36
Drew Wilimitis, Robert W. Turer, Michael Ripperger, et al.
JAMA Network Open (2022) Vol. 5, Iss. 5, pp. e2212095-e2212095
Open Access | Times Cited: 36
The performance of machine learning models in predicting suicidal ideation, attempts, and deaths: A meta-analysis and systematic review
Karen Kusuma, Mark Larsen, Juan C. Quiroz, et al.
Journal of Psychiatric Research (2022) Vol. 155, pp. 579-588
Closed Access | Times Cited: 35
Karen Kusuma, Mark Larsen, Juan C. Quiroz, et al.
Journal of Psychiatric Research (2022) Vol. 155, pp. 579-588
Closed Access | Times Cited: 35
Gender Differences in Machine Learning Models of Trauma and Suicidal Ideation in Veterans of the Iraq and Afghanistan Wars
Jaimie L. Gradus, M. King, Isaac R. Galatzer‐Levy, et al.
Journal of Traumatic Stress (2017) Vol. 30, Iss. 4, pp. 362-371
Open Access | Times Cited: 52
Jaimie L. Gradus, M. King, Isaac R. Galatzer‐Levy, et al.
Journal of Traumatic Stress (2017) Vol. 30, Iss. 4, pp. 362-371
Open Access | Times Cited: 52
Suicide Risk Assessment and Prevention: A Systematic Review Focusing on Veterans
Heidi D Nelson, Lauren M. Denneson, Allison Low, et al.
Psychiatric Services (2017) Vol. 68, Iss. 10, pp. 1003-1015
Open Access | Times Cited: 49
Heidi D Nelson, Lauren M. Denneson, Allison Low, et al.
Psychiatric Services (2017) Vol. 68, Iss. 10, pp. 1003-1015
Open Access | Times Cited: 49
Prospective prediction of suicide attempts in community adolescents and young adults, using regression methods and machine learning
Marcel Miché, Erich Studerus, Andrea H. Meyer, et al.
Journal of Affective Disorders (2019) Vol. 265, pp. 570-578
Closed Access | Times Cited: 48
Marcel Miché, Erich Studerus, Andrea H. Meyer, et al.
Journal of Affective Disorders (2019) Vol. 265, pp. 570-578
Closed Access | Times Cited: 48
Predictors of serious suicidal behavior in late-life depression
Katalin Szántó, Hanga Galfalvy, Laura B. Kenneally, et al.
European Neuropsychopharmacology (2020) Vol. 40, pp. 85-98
Open Access | Times Cited: 44
Katalin Szántó, Hanga Galfalvy, Laura B. Kenneally, et al.
European Neuropsychopharmacology (2020) Vol. 40, pp. 85-98
Open Access | Times Cited: 44
Using Administrative Data to Predict Suicide After Psychiatric Hospitalization in the Veterans Health Administration System
Ronald C. Kessler, Mark S. Bauer, Todd M. Bishop, et al.
Frontiers in Psychiatry (2020) Vol. 11
Open Access | Times Cited: 40
Ronald C. Kessler, Mark S. Bauer, Todd M. Bishop, et al.
Frontiers in Psychiatry (2020) Vol. 11
Open Access | Times Cited: 40
Evaluating the Machine Learning Literature: A Primer and User’s Guide for Psychiatrists
Adrienne Grzenda, Nina V. Kraguljac, William M. McDonald, et al.
American Journal of Psychiatry (2021) Vol. 178, Iss. 8, pp. 715-729
Closed Access | Times Cited: 38
Adrienne Grzenda, Nina V. Kraguljac, William M. McDonald, et al.
American Journal of Psychiatry (2021) Vol. 178, Iss. 8, pp. 715-729
Closed Access | Times Cited: 38
Meta-analysis of the strength of exploratory suicide prediction models; from clinicians to computers
Michelle Corke, Katherine Mullin, Helena N. Angel-Scott, et al.
BJPsych Open (2021) Vol. 7, Iss. 1
Open Access | Times Cited: 33
Michelle Corke, Katherine Mullin, Helena N. Angel-Scott, et al.
BJPsych Open (2021) Vol. 7, Iss. 1
Open Access | Times Cited: 33
Reconciling Statistical and Clinicians’ Predictions of Suicide Risk
Gregory E. Simon, Bridget B. Matarazzo, Colin G. Walsh, et al.
Psychiatric Services (2021) Vol. 72, Iss. 5, pp. 555-562
Open Access | Times Cited: 33
Gregory E. Simon, Bridget B. Matarazzo, Colin G. Walsh, et al.
Psychiatric Services (2021) Vol. 72, Iss. 5, pp. 555-562
Open Access | Times Cited: 33
Complex modeling with detailed temporal predictors does not improve health records-based suicide risk prediction
Susan M. Shortreed, Rod Walker, Eric Johnson, et al.
npj Digital Medicine (2023) Vol. 6, Iss. 1
Open Access | Times Cited: 16
Susan M. Shortreed, Rod Walker, Eric Johnson, et al.
npj Digital Medicine (2023) Vol. 6, Iss. 1
Open Access | Times Cited: 16
Using Neural Networks with Routine Health Records to Identify Suicide Risk: Feasibility Study
Marcos DelPozo‐Baños, Ann John, Nicolai Petkov, et al.
JMIR Mental Health (2018) Vol. 5, Iss. 2, pp. e10144-e10144
Open Access | Times Cited: 46
Marcos DelPozo‐Baños, Ann John, Nicolai Petkov, et al.
JMIR Mental Health (2018) Vol. 5, Iss. 2, pp. e10144-e10144
Open Access | Times Cited: 46
Assessing and Minimizing Re-identification Risk in Research Data Derived from Health Care Records
Gregory E. Simon, Susan M. Shortreed, R. Yates Coley, et al.
eGEMs (Generating Evidence & Methods to improve patient outcomes) (2019) Vol. 7, Iss. 1, pp. 6-6
Open Access | Times Cited: 42
Gregory E. Simon, Susan M. Shortreed, R. Yates Coley, et al.
eGEMs (Generating Evidence & Methods to improve patient outcomes) (2019) Vol. 7, Iss. 1, pp. 6-6
Open Access | Times Cited: 42
Risk Factors Associated With Attempted Suicide Among US Army Soldiers Without a History of Mental Health Diagnosis
Robert J. Ursano, Ronald C. Kessler, James A. Naifeh, et al.
JAMA Psychiatry (2018) Vol. 75, Iss. 10, pp. 1022-1022
Open Access | Times Cited: 41
Robert J. Ursano, Ronald C. Kessler, James A. Naifeh, et al.
JAMA Psychiatry (2018) Vol. 75, Iss. 10, pp. 1022-1022
Open Access | Times Cited: 41
Expression of Concern: A gingivitis identification method based on contrast‐limited adaptive histogram equalization, gray‐level co‐occurrence matrix, and extreme learning machine
Wen Li, Yiyang Chen, Weibin Sun, et al.
International Journal of Imaging Systems and Technology (2018) Vol. 29, Iss. 1, pp. 77-82
Closed Access | Times Cited: 40
Wen Li, Yiyang Chen, Weibin Sun, et al.
International Journal of Imaging Systems and Technology (2018) Vol. 29, Iss. 1, pp. 77-82
Closed Access | Times Cited: 40
Predicting suicide attempts among soldiers who deny suicidal ideation in the Army Study to Assess Risk and Resilience in Servicemembers (Army STARRS)
Samantha L. Bernecker, Kelly L. Zuromski, Peter M. Gutierrez, et al.
Behaviour Research and Therapy (2018) Vol. 120, pp. 103350-103350
Open Access | Times Cited: 39
Samantha L. Bernecker, Kelly L. Zuromski, Peter M. Gutierrez, et al.
Behaviour Research and Therapy (2018) Vol. 120, pp. 103350-103350
Open Access | Times Cited: 39
Identifying and Predicting Intentional Self-Harm in Electronic Health Record Clinical Notes: Deep Learning Approach
Jihad S. Obeid, Jennifer Dahne, Sean Christensen, et al.
JMIR Medical Informatics (2020) Vol. 8, Iss. 7, pp. e17784-e17784
Open Access | Times Cited: 39
Jihad S. Obeid, Jennifer Dahne, Sean Christensen, et al.
JMIR Medical Informatics (2020) Vol. 8, Iss. 7, pp. e17784-e17784
Open Access | Times Cited: 39
Why Are Suicide Rates Increasing in the United States? Towards a Multilevel Reimagination of Suicide Prevention
Gonzalo Martínez‐Alés, Daniel Hernández-Calle, Nicole Khauli, et al.
Current topics in behavioral neurosciences (2020), pp. 1-23
Open Access | Times Cited: 38
Gonzalo Martínez‐Alés, Daniel Hernández-Calle, Nicole Khauli, et al.
Current topics in behavioral neurosciences (2020), pp. 1-23
Open Access | Times Cited: 38
AI enabled suicide prediction tools: a qualitative narrative review
Daniel D’Hotman, Erwin Loh
BMJ Health & Care Informatics (2020) Vol. 27, Iss. 3, pp. e100175-e100175
Open Access | Times Cited: 38
Daniel D’Hotman, Erwin Loh
BMJ Health & Care Informatics (2020) Vol. 27, Iss. 3, pp. e100175-e100175
Open Access | Times Cited: 38
The Role of Big Data Analytics in Predicting Suicide
Ronald C. Kessler, Samantha L. Bernecker, Robert M. Bossarte, et al.
Springer eBooks (2019), pp. 77-98
Closed Access | Times Cited: 37
Ronald C. Kessler, Samantha L. Bernecker, Robert M. Bossarte, et al.
Springer eBooks (2019), pp. 77-98
Closed Access | Times Cited: 37
Using machine learning to classify suicide attempt history among youth in medical care settings
Taylor A. Burke, Ross Jacobucci, Brooke A. Ammerman, et al.
Journal of Affective Disorders (2020) Vol. 268, pp. 206-214
Closed Access | Times Cited: 34
Taylor A. Burke, Ross Jacobucci, Brooke A. Ammerman, et al.
Journal of Affective Disorders (2020) Vol. 268, pp. 206-214
Closed Access | Times Cited: 34