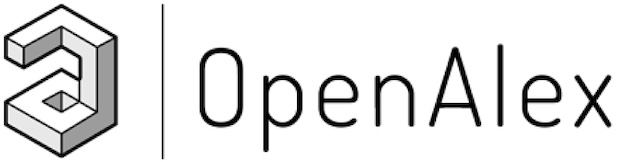
OpenAlex is a bibliographic catalogue of scientific papers, authors and institutions accessible in open access mode, named after the Library of Alexandria. It's citation coverage is excellent and I hope you will find utility in this listing of citing articles!
If you click the article title, you'll navigate to the article, as listed in CrossRef. If you click the Open Access links, you'll navigate to the "best Open Access location". Clicking the citation count will open this listing for that article. Lastly at the bottom of the page, you'll find basic pagination options.
Requested Article:
CoAuthor: Designing a Human-AI Collaborative Writing Dataset for Exploring Language Model Capabilities
Mina Lee, Percy Liang, Qian Yang
CHI Conference on Human Factors in Computing Systems (2022)
Open Access | Times Cited: 225
Mina Lee, Percy Liang, Qian Yang
CHI Conference on Human Factors in Computing Systems (2022)
Open Access | Times Cited: 225
Showing 26-50 of 225 citing articles:
The Promise and Peril of ChatGPT in Higher Education: Opportunities, Challenges, and Design Implications
Hyanghee Park, Daehwan Ahn
(2024), pp. 1-21
Open Access | Times Cited: 23
Hyanghee Park, Daehwan Ahn
(2024), pp. 1-21
Open Access | Times Cited: 23
Teachers, Parents, and Students' perspectives on Integrating Generative AI into Elementary Literacy Education
Ariel Han, Xiaofei Zhou, Zhenyao Cai, et al.
(2024), pp. 1-17
Open Access | Times Cited: 21
Ariel Han, Xiaofei Zhou, Zhenyao Cai, et al.
(2024), pp. 1-17
Open Access | Times Cited: 21
Shaping Human-AI Collaboration: Varied Scaffolding Levels in Co-writing with Language Models
Paramveer S. Dhillon, Somayeh Molaei, Jiaqi Li, et al.
(2024), pp. 1-18
Open Access | Times Cited: 21
Paramveer S. Dhillon, Somayeh Molaei, Jiaqi Li, et al.
(2024), pp. 1-18
Open Access | Times Cited: 21
From explainable to interactive AI: A literature review on current trends in human-AI interaction
Muhammad Raees, Inge Meijerink, Ioanna Lykourentzou, et al.
International Journal of Human-Computer Studies (2024) Vol. 189, pp. 103301-103301
Open Access | Times Cited: 19
Muhammad Raees, Inge Meijerink, Ioanna Lykourentzou, et al.
International Journal of Human-Computer Studies (2024) Vol. 189, pp. 103301-103301
Open Access | Times Cited: 19
DiaryMate: Understanding User Perceptions and Experience in Human-AI Collaboration for Personal Journaling
Taewan Kim, Dong-Hoon Shin, Young‐Ho Kim, et al.
(2024), pp. 1-15
Open Access | Times Cited: 18
Taewan Kim, Dong-Hoon Shin, Young‐Ho Kim, et al.
(2024), pp. 1-15
Open Access | Times Cited: 18
ABScribe: Rapid Exploration & Organization of Multiple Writing Variations in Human-AI Co-Writing Tasks using Large Language Models
Mohi Reza, Nathan Laundry, Ilya Musabirov, et al.
(2024), pp. 1-18
Open Access | Times Cited: 18
Mohi Reza, Nathan Laundry, Ilya Musabirov, et al.
(2024), pp. 1-18
Open Access | Times Cited: 18
Evaluating language models for mathematics through interactions
Katherine M. Collins, Albert Q. Jiang, Simon Frieder, et al.
Proceedings of the National Academy of Sciences (2024) Vol. 121, Iss. 24
Open Access | Times Cited: 18
Katherine M. Collins, Albert Q. Jiang, Simon Frieder, et al.
Proceedings of the National Academy of Sciences (2024) Vol. 121, Iss. 24
Open Access | Times Cited: 18
On the creativity of large language models
Giorgio Franceschelli, Mirco Musolesi
AI & Society (2024)
Open Access | Times Cited: 18
Giorgio Franceschelli, Mirco Musolesi
AI & Society (2024)
Open Access | Times Cited: 18
Modifying AI, Enhancing Essays: How Active Engagement with Generative AI Boosts Writing Quality
Kaixun Yang, Mladen Raković, Zhiping Liang, et al.
(2025), pp. 568-578
Open Access | Times Cited: 2
Kaixun Yang, Mladen Raković, Zhiping Liang, et al.
(2025), pp. 568-578
Open Access | Times Cited: 2
Beyond Text Generation: Supporting Writers with Continuous Automatic Text Summaries
Hai Dang, Karim Benharrak, Florian Lehmann, et al.
(2022), pp. 1-13
Open Access | Times Cited: 56
Hai Dang, Karim Benharrak, Florian Lehmann, et al.
(2022), pp. 1-13
Open Access | Times Cited: 56
VISAR: A Human-AI Argumentative Writing Assistant with Visual Programming and Rapid Draft Prototyping
Zheng Zhang, Jie Gao, Ranjodh Singh Dhaliwal, et al.
(2023), pp. 1-30
Open Access | Times Cited: 43
Zheng Zhang, Jie Gao, Ranjodh Singh Dhaliwal, et al.
(2023), pp. 1-30
Open Access | Times Cited: 43
Designerly Understanding: Information Needs for Model Transparency to Support Design Ideation for AI-Powered User Experience
Q. Vera Liao, Hariharan Subramonyam, Jennifer Wang, et al.
(2023), pp. 1-21
Open Access | Times Cited: 42
Q. Vera Liao, Hariharan Subramonyam, Jennifer Wang, et al.
(2023), pp. 1-21
Open Access | Times Cited: 42
Herding AI Cats: Lessons from Designing a Chatbot by Prompting GPT-3
J.D. Zamfirescu-Pereira, Heather Wei, Amy Xiao, et al.
(2023), pp. 2206-2220
Open Access | Times Cited: 42
J.D. Zamfirescu-Pereira, Heather Wei, Amy Xiao, et al.
(2023), pp. 2206-2220
Open Access | Times Cited: 42
The AI Ghostwriter Effect: When Users do not Perceive Ownership of AI-Generated Text but Self-Declare as Authors
Fiona Draxler, Anna Werner, Florian Lehmann, et al.
ACM Transactions on Computer-Human Interaction (2023) Vol. 31, Iss. 2, pp. 1-40
Open Access | Times Cited: 36
Fiona Draxler, Anna Werner, Florian Lehmann, et al.
ACM Transactions on Computer-Human Interaction (2023) Vol. 31, Iss. 2, pp. 1-40
Open Access | Times Cited: 36
Marked Personas: Using Natural Language Prompts to Measure Stereotypes in Language Models
Myra Cheng, Esin Durmus, Dan Jurafsky
(2023)
Open Access | Times Cited: 33
Myra Cheng, Esin Durmus, Dan Jurafsky
(2023)
Open Access | Times Cited: 33
Generative Image AI Using Design Sketches as input: Opportunities and Challenges
Chengzhi Zhang, Weijie Wang, Paul Pangaro, et al.
Creativity and Cognition (2023)
Open Access | Times Cited: 32
Chengzhi Zhang, Weijie Wang, Paul Pangaro, et al.
Creativity and Cognition (2023)
Open Access | Times Cited: 32
Metaphorian: Leveraging Large Language Models to Support Extended Metaphor Creation for Science Writing
Jeongyeon Kim, Sangho Suh, Lydia B. Chilton, et al.
(2023)
Open Access | Times Cited: 29
Jeongyeon Kim, Sangho Suh, Lydia B. Chilton, et al.
(2023)
Open Access | Times Cited: 29
A systematic review of artificial intelligence technologies used for story writing
Xiaoxuan Fang, Davy Tsz Kit Ng, Jac Ka Lok Leung, et al.
Education and Information Technologies (2023) Vol. 28, Iss. 11, pp. 14361-14397
Closed Access | Times Cited: 28
Xiaoxuan Fang, Davy Tsz Kit Ng, Jac Ka Lok Leung, et al.
Education and Information Technologies (2023) Vol. 28, Iss. 11, pp. 14361-14397
Closed Access | Times Cited: 28
Understanding EFL students’ chatbot-assisted argumentative writing: An activity theory perspective
Kai Guo, Yuanke Li, Yongyan Li, et al.
Education and Information Technologies (2023) Vol. 29, Iss. 1, pp. 1-20
Closed Access | Times Cited: 28
Kai Guo, Yuanke Li, Yongyan Li, et al.
Education and Information Technologies (2023) Vol. 29, Iss. 1, pp. 1-20
Closed Access | Times Cited: 28
Interacting with Next-Phrase Suggestions: How Suggestion Systems Aid and Influence the Cognitive Processes of Writing
Advait Bhat, Saaket Agashe, Parth Oberoi, et al.
(2023)
Open Access | Times Cited: 26
Advait Bhat, Saaket Agashe, Parth Oberoi, et al.
(2023)
Open Access | Times Cited: 26
We’re Afraid Language Models Aren’t Modeling Ambiguity
Alisa Liu, Zhaofeng Wu, Julian Michael, et al.
Proceedings of the 2021 Conference on Empirical Methods in Natural Language Processing (2023), pp. 790-807
Open Access | Times Cited: 25
Alisa Liu, Zhaofeng Wu, Julian Michael, et al.
Proceedings of the 2021 Conference on Empirical Methods in Natural Language Processing (2023), pp. 790-807
Open Access | Times Cited: 25
How AI Processing Delays Foster Creativity: Exploring Research Question Co-Creation with an LLM-based Agent
Yiren Liu, Si Chen, Haocong Cheng, et al.
(2024), pp. 1-25
Open Access | Times Cited: 17
Yiren Liu, Si Chen, Haocong Cheng, et al.
(2024), pp. 1-25
Open Access | Times Cited: 17
Investigating and Designing for Trust in AI-powered Code Generation Tools
Ruotong Wang, Ruijia Cheng, Denae Ford, et al.
2022 ACM Conference on Fairness, Accountability, and Transparency (2024)
Open Access | Times Cited: 17
Ruotong Wang, Ruijia Cheng, Denae Ford, et al.
2022 ACM Conference on Fairness, Accountability, and Transparency (2024)
Open Access | Times Cited: 17
FederatedScope-LLM: A Comprehensive Package for Fine-tuning Large Language Models in Federated Learning
Weirui Kuang, Bingchen Qian, Zitao Li, et al.
Proceedings of the 28th ACM SIGKDD Conference on Knowledge Discovery and Data Mining (2024), pp. 5260-5271
Open Access | Times Cited: 17
Weirui Kuang, Bingchen Qian, Zitao Li, et al.
Proceedings of the 28th ACM SIGKDD Conference on Knowledge Discovery and Data Mining (2024), pp. 5260-5271
Open Access | Times Cited: 17
Measuring the Interpretability and Explainability of Model Decisions of Five Large Language Models
Kaito Fujiwara, Miyu Sasaki, Akira Nakamura, et al.
(2024)
Open Access | Times Cited: 14
Kaito Fujiwara, Miyu Sasaki, Akira Nakamura, et al.
(2024)
Open Access | Times Cited: 14