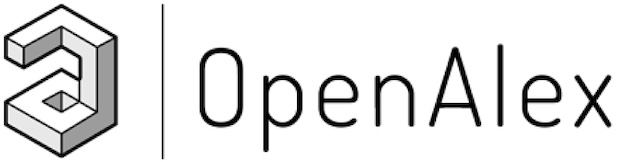
OpenAlex is a bibliographic catalogue of scientific papers, authors and institutions accessible in open access mode, named after the Library of Alexandria. It's citation coverage is excellent and I hope you will find utility in this listing of citing articles!
If you click the article title, you'll navigate to the article, as listed in CrossRef. If you click the Open Access links, you'll navigate to the "best Open Access location". Clicking the citation count will open this listing for that article. Lastly at the bottom of the page, you'll find basic pagination options.
Requested Article:
Toward Predicting Efficiency of Organic Solar Cells via Machine Learning and Improved Descriptors
Harikrishna Sahu, Weining Rao, Alessandro Troisi, et al.
Advanced Energy Materials (2018) Vol. 8, Iss. 24
Closed Access | Times Cited: 226
Harikrishna Sahu, Weining Rao, Alessandro Troisi, et al.
Advanced Energy Materials (2018) Vol. 8, Iss. 24
Closed Access | Times Cited: 226
Showing 1-25 of 226 citing articles:
A Critical Review of Machine Learning of Energy Materials
Chi Chen, Yunxing Zuo, Weike Ye, et al.
Advanced Energy Materials (2020) Vol. 10, Iss. 8
Closed Access | Times Cited: 447
Chi Chen, Yunxing Zuo, Weike Ye, et al.
Advanced Energy Materials (2020) Vol. 10, Iss. 8
Closed Access | Times Cited: 447
Toward ideal hole transport materials: a review on recent progress in dopant-free hole transport materials for fabricating efficient and stable perovskite solar cells
Xinxing Yin, Zhaoning Song, Zaifang Li, et al.
Energy & Environmental Science (2020) Vol. 13, Iss. 11, pp. 4057-4086
Closed Access | Times Cited: 326
Xinxing Yin, Zhaoning Song, Zaifang Li, et al.
Energy & Environmental Science (2020) Vol. 13, Iss. 11, pp. 4057-4086
Closed Access | Times Cited: 326
Machine learning for high performance organic solar cells: current scenario and future prospects
Asif Mahmood, Jin‐Liang Wang
Energy & Environmental Science (2020) Vol. 14, Iss. 1, pp. 90-105
Closed Access | Times Cited: 312
Asif Mahmood, Jin‐Liang Wang
Energy & Environmental Science (2020) Vol. 14, Iss. 1, pp. 90-105
Closed Access | Times Cited: 312
Machine learning: Accelerating materials development for energy storage and conversion
An Chen, Xu Zhang, Zhen Zhou
InfoMat (2020) Vol. 2, Iss. 3, pp. 553-576
Open Access | Times Cited: 289
An Chen, Xu Zhang, Zhen Zhou
InfoMat (2020) Vol. 2, Iss. 3, pp. 553-576
Open Access | Times Cited: 289
Machine learning–assisted molecular design and efficiency prediction for high-performance organic photovoltaic materials
Wenbo Sun, Yu Zheng, Ke Yang, et al.
Science Advances (2019) Vol. 5, Iss. 11
Open Access | Times Cited: 279
Wenbo Sun, Yu Zheng, Ke Yang, et al.
Science Advances (2019) Vol. 5, Iss. 11
Open Access | Times Cited: 279
The Path to 20% Power Conversion Efficiencies in Nonfullerene Acceptor Organic Solar Cells
Akchheta Karki, Alexander J. Gillett, Richard H. Friend, et al.
Advanced Energy Materials (2020) Vol. 11, Iss. 15
Open Access | Times Cited: 196
Akchheta Karki, Alexander J. Gillett, Richard H. Friend, et al.
Advanced Energy Materials (2020) Vol. 11, Iss. 15
Open Access | Times Cited: 196
A time and resource efficient machine learning assisted design of non-fullerene small molecule acceptors for P3HT-based organic solar cells and green solvent selection
Asif Mahmood, Jin‐Liang Wang
Journal of Materials Chemistry A (2021) Vol. 9, Iss. 28, pp. 15684-15695
Closed Access | Times Cited: 183
Asif Mahmood, Jin‐Liang Wang
Journal of Materials Chemistry A (2021) Vol. 9, Iss. 28, pp. 15684-15695
Closed Access | Times Cited: 183
Machine learning on sustainable energy: A review and outlook on renewable energy systems, catalysis, smart grid and energy storage
Daniel Rangel-Martínez, K.D.P. Nigam, Luis Ricardez‐Sandoval
Process Safety and Environmental Protection (2021) Vol. 174, pp. 414-441
Closed Access | Times Cited: 179
Daniel Rangel-Martínez, K.D.P. Nigam, Luis Ricardez‐Sandoval
Process Safety and Environmental Protection (2021) Vol. 174, pp. 414-441
Closed Access | Times Cited: 179
Molecular excited states through a machine learning lens
Pavlo O. Dral, Mario Barbatti
Nature Reviews Chemistry (2021) Vol. 5, Iss. 6, pp. 388-405
Closed Access | Times Cited: 177
Pavlo O. Dral, Mario Barbatti
Nature Reviews Chemistry (2021) Vol. 5, Iss. 6, pp. 388-405
Closed Access | Times Cited: 177
Combining electronic and structural features in machine learning models to predict organic solar cells properties
Daniele Padula, Jack D. Simpson, Alessandro Troisi
Materials Horizons (2018) Vol. 6, Iss. 2, pp. 343-349
Closed Access | Times Cited: 169
Daniele Padula, Jack D. Simpson, Alessandro Troisi
Materials Horizons (2018) Vol. 6, Iss. 2, pp. 343-349
Closed Access | Times Cited: 169
Predictions and Strategies Learned from Machine Learning to Develop High‐Performing Perovskite Solar Cells
Jinxin Li, Basudev Pradhan, Surya Gaur, et al.
Advanced Energy Materials (2019) Vol. 9, Iss. 46
Closed Access | Times Cited: 154
Jinxin Li, Basudev Pradhan, Surya Gaur, et al.
Advanced Energy Materials (2019) Vol. 9, Iss. 46
Closed Access | Times Cited: 154
Directly predicting limiting potentials from easily obtainable physical properties of graphene-supported single-atom electrocatalysts by machine learning
Shiru Lin, Haoxiang Xu, Yekun Wang, et al.
Journal of Materials Chemistry A (2020) Vol. 8, Iss. 11, pp. 5663-5670
Closed Access | Times Cited: 142
Shiru Lin, Haoxiang Xu, Yekun Wang, et al.
Journal of Materials Chemistry A (2020) Vol. 8, Iss. 11, pp. 5663-5670
Closed Access | Times Cited: 142
Gryffin : An algorithm for Bayesian optimization of categorical variables informed by expert knowledge
Florian Häse, Matteo Aldeghi, Riley J. Hickman, et al.
Applied Physics Reviews (2021) Vol. 8, Iss. 3
Open Access | Times Cited: 108
Florian Häse, Matteo Aldeghi, Riley J. Hickman, et al.
Applied Physics Reviews (2021) Vol. 8, Iss. 3
Open Access | Times Cited: 108
Data‐Driven Materials Innovation and Applications
Zhuo Wang, Zhehao Sun, Hang Yin, et al.
Advanced Materials (2022) Vol. 34, Iss. 36
Closed Access | Times Cited: 108
Zhuo Wang, Zhehao Sun, Hang Yin, et al.
Advanced Materials (2022) Vol. 34, Iss. 36
Closed Access | Times Cited: 108
Machine Learning for Perovskite Solar Cells and Component Materials: Key Technologies and Prospects
Yiming Liu, Xinyu Tan, Jie Liang, et al.
Advanced Functional Materials (2023) Vol. 33, Iss. 17
Closed Access | Times Cited: 84
Yiming Liu, Xinyu Tan, Jie Liang, et al.
Advanced Functional Materials (2023) Vol. 33, Iss. 17
Closed Access | Times Cited: 84
AI for Nanomaterials Development in Clean Energy and Carbon Capture, Utilization and Storage (CCUS)
Honghao Chen, Yingzhe Zheng, Jiali Li, et al.
ACS Nano (2023) Vol. 17, Iss. 11, pp. 9763-9792
Closed Access | Times Cited: 50
Honghao Chen, Yingzhe Zheng, Jiali Li, et al.
ACS Nano (2023) Vol. 17, Iss. 11, pp. 9763-9792
Closed Access | Times Cited: 50
A bibliometric analysis of machine learning techniques in photovoltaic cells and solar energy (2014–2022)
Abdelhamid Zaïdi
Energy Reports (2024) Vol. 11, pp. 2768-2779
Open Access | Times Cited: 26
Abdelhamid Zaïdi
Energy Reports (2024) Vol. 11, pp. 2768-2779
Open Access | Times Cited: 26
Machine Learning Approaches in Polymer Science: Progress and Fundamental for a New Paradigm
Chunhui Xie, Haoke Qiu, Lu Liu, et al.
SmartMat (2025) Vol. 6, Iss. 1
Open Access | Times Cited: 4
Chunhui Xie, Haoke Qiu, Lu Liu, et al.
SmartMat (2025) Vol. 6, Iss. 1
Open Access | Times Cited: 4
Coupling a Crystal Graph Multilayer Descriptor to Active Learning for Rapid Discovery of 2D Ferromagnetic Semiconductors/Half‐Metals/Metals
Shuaihua Lu, Qionghua Zhou, Yilv Guo, et al.
Advanced Materials (2020) Vol. 32, Iss. 29
Closed Access | Times Cited: 119
Shuaihua Lu, Qionghua Zhou, Yilv Guo, et al.
Advanced Materials (2020) Vol. 32, Iss. 29
Closed Access | Times Cited: 119
Machine learning for accelerating the discovery of high-performance donor/acceptor pairs in non-fullerene organic solar cells
Yao Wu, Jie Guo, Rui Sun, et al.
npj Computational Materials (2020) Vol. 6, Iss. 1
Open Access | Times Cited: 119
Yao Wu, Jie Guo, Rui Sun, et al.
npj Computational Materials (2020) Vol. 6, Iss. 1
Open Access | Times Cited: 119
Artificial Intelligence to Power the Future of Materials Science and Engineering
Wuxin Sha, Yaqing Guo, Qing Yuan, et al.
Advanced Intelligent Systems (2020) Vol. 2, Iss. 4
Open Access | Times Cited: 107
Wuxin Sha, Yaqing Guo, Qing Yuan, et al.
Advanced Intelligent Systems (2020) Vol. 2, Iss. 4
Open Access | Times Cited: 107
Machine learning property prediction for organic photovoltaic devices
Nastaran Meftahi, Mykhailo V. Klymenko, Andrew J. Christofferson, et al.
npj Computational Materials (2020) Vol. 6, Iss. 1
Open Access | Times Cited: 107
Nastaran Meftahi, Mykhailo V. Klymenko, Andrew J. Christofferson, et al.
npj Computational Materials (2020) Vol. 6, Iss. 1
Open Access | Times Cited: 107
Machine Learning Enables Highly Accurate Predictions of Photophysical Properties of Organic Fluorescent Materials: Emission Wavelengths and Quantum Yields
Cheng‐Wei Ju, Hanzhi Bai, Bo Li, et al.
Journal of Chemical Information and Modeling (2021) Vol. 61, Iss. 3, pp. 1053-1065
Closed Access | Times Cited: 101
Cheng‐Wei Ju, Hanzhi Bai, Bo Li, et al.
Journal of Chemical Information and Modeling (2021) Vol. 61, Iss. 3, pp. 1053-1065
Closed Access | Times Cited: 101
Unraveling Correlations between Molecular Properties and Device Parameters of Organic Solar Cells Using Machine Learning
Harikrishna Sahu, Haibo Ma
The Journal of Physical Chemistry Letters (2019) Vol. 10, Iss. 22, pp. 7277-7284
Closed Access | Times Cited: 100
Harikrishna Sahu, Haibo Ma
The Journal of Physical Chemistry Letters (2019) Vol. 10, Iss. 22, pp. 7277-7284
Closed Access | Times Cited: 100
Designing and understanding light-harvesting devices with machine learning
Florian Häse, Loı̈c M. Roch, Pascal Friederich, et al.
Nature Communications (2020) Vol. 11, Iss. 1
Open Access | Times Cited: 100
Florian Häse, Loı̈c M. Roch, Pascal Friederich, et al.
Nature Communications (2020) Vol. 11, Iss. 1
Open Access | Times Cited: 100