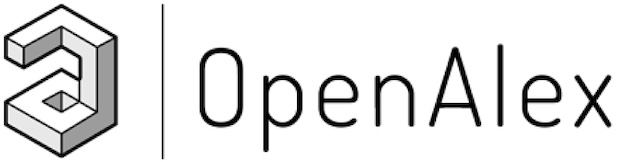
OpenAlex is a bibliographic catalogue of scientific papers, authors and institutions accessible in open access mode, named after the Library of Alexandria. It's citation coverage is excellent and I hope you will find utility in this listing of citing articles!
If you click the article title, you'll navigate to the article, as listed in CrossRef. If you click the Open Access links, you'll navigate to the "best Open Access location". Clicking the citation count will open this listing for that article. Lastly at the bottom of the page, you'll find basic pagination options.
Requested Article:
Retracted: Prediction of the elastic modulus of recycled aggregate concrete applying hybrid artificial intelligence and machine learning algorithms
Qian Zhang, Mansour Afzal
Structural Concrete (2021) Vol. 23, Iss. 4, pp. 2477-2495
Closed Access | Times Cited: 28
Qian Zhang, Mansour Afzal
Structural Concrete (2021) Vol. 23, Iss. 4, pp. 2477-2495
Closed Access | Times Cited: 28
Showing 1-25 of 28 citing articles:
Prediction and optimization model of sustainable concrete properties using machine learning, deep learning and swarm intelligence: A review
Shiqi Wang, Peng Xia, Keyu Chen, et al.
Journal of Building Engineering (2023) Vol. 80, pp. 108065-108065
Closed Access | Times Cited: 62
Shiqi Wang, Peng Xia, Keyu Chen, et al.
Journal of Building Engineering (2023) Vol. 80, pp. 108065-108065
Closed Access | Times Cited: 62
Artificial-intelligence-led revolution of construction materials: From molecules to Industry 4.0
Xing Quan Wang, Pengguang Chen, Cheuk Lun Chow, et al.
Matter (2023) Vol. 6, Iss. 6, pp. 1831-1859
Open Access | Times Cited: 52
Xing Quan Wang, Pengguang Chen, Cheuk Lun Chow, et al.
Matter (2023) Vol. 6, Iss. 6, pp. 1831-1859
Open Access | Times Cited: 52
Predicting compressive and flexural strength of high-performance concrete using a dynamic Catboost Regression model combined with individual and ensemble optimization techniques
Yali Wu, Huan Huang
Materials Today Communications (2024) Vol. 38, pp. 108174-108174
Closed Access | Times Cited: 13
Yali Wu, Huan Huang
Materials Today Communications (2024) Vol. 38, pp. 108174-108174
Closed Access | Times Cited: 13
Predicting the elasticity modulus of sedimentary rocks using Deep Random Forest Optimization (DRFO) algorithm
Yimin Mao, Yican Li, Fei Teng, et al.
Environmental Earth Sciences (2024) Vol. 83, Iss. 16
Closed Access | Times Cited: 10
Yimin Mao, Yican Li, Fei Teng, et al.
Environmental Earth Sciences (2024) Vol. 83, Iss. 16
Closed Access | Times Cited: 10
Retracted: A comparative study on predicting the rapid chloride permeability of self‐compacting concrete using meta‐heuristic algorithm and artificial intelligence techniques
Yuan Jia, Ming Zhao, Mahzad Esmaeili‐Falak
Structural Concrete (2022) Vol. 23, Iss. 2, pp. 753-774
Closed Access | Times Cited: 36
Yuan Jia, Ming Zhao, Mahzad Esmaeili‐Falak
Structural Concrete (2022) Vol. 23, Iss. 2, pp. 753-774
Closed Access | Times Cited: 36
Interpretable Machine Learning for Prediction of Post-Fire Self-Healing of Concrete
Magdalena Rajczakowska, Maciej Szeląg, Karin Habermehl-Cwirzen, et al.
Materials (2023) Vol. 16, Iss. 3, pp. 1273-1273
Open Access | Times Cited: 23
Magdalena Rajczakowska, Maciej Szeląg, Karin Habermehl-Cwirzen, et al.
Materials (2023) Vol. 16, Iss. 3, pp. 1273-1273
Open Access | Times Cited: 23
Artificial intelligence techniques in advanced concrete technology: A comprehensive survey on 10 years research trend
Ramin Kazemi
Engineering Reports (2023) Vol. 5, Iss. 9
Open Access | Times Cited: 21
Ramin Kazemi
Engineering Reports (2023) Vol. 5, Iss. 9
Open Access | Times Cited: 21
Prediction and uncertainty quantification of compressive strength of high‐strength concrete using optimized machine learning algorithms
Bing Han, Yanqi Wu, Lulu Liu
Structural Concrete (2022) Vol. 23, Iss. 6, pp. 3772-3785
Closed Access | Times Cited: 27
Bing Han, Yanqi Wu, Lulu Liu
Structural Concrete (2022) Vol. 23, Iss. 6, pp. 3772-3785
Closed Access | Times Cited: 27
Non-destructive density-corrected estimation of the elastic modulus of slag-cement self-compacting concrete containing recycled aggregate
Víctor Revilla‐Cuesta, Jinyan Shi, Marta Skaf, et al.
Developments in the Built Environment (2022) Vol. 12, pp. 100097-100097
Open Access | Times Cited: 24
Víctor Revilla‐Cuesta, Jinyan Shi, Marta Skaf, et al.
Developments in the Built Environment (2022) Vol. 12, pp. 100097-100097
Open Access | Times Cited: 24
A Detailed Review of Equations for Estimating Elastic Modulus in Specialty Concretes
C. Puttbach, Gary S. Prinz, Cameron D. Murray
Journal of Materials in Civil Engineering (2023) Vol. 35, Iss. 6
Closed Access | Times Cited: 10
C. Puttbach, Gary S. Prinz, Cameron D. Murray
Journal of Materials in Civil Engineering (2023) Vol. 35, Iss. 6
Closed Access | Times Cited: 10
Integrated finite element analysis and machine learning approach for exploring the flexural behavior of steel-spontaneous combustion coal gangue aggregate concrete composite beams with studs
Tirui Zhang, Qinghe Wang, Xinlong Zhang, et al.
Structures (2025) Vol. 72, pp. 108265-108265
Closed Access
Tirui Zhang, Qinghe Wang, Xinlong Zhang, et al.
Structures (2025) Vol. 72, pp. 108265-108265
Closed Access
Machine Learning as an Innovative Engineering Tool for Controlling Concrete Performance: A Comprehensive Review
Fatemeh Mobasheri, Masoud Hosseinpoor, Ammar Yahia, et al.
Archives of Computational Methods in Engineering (2025)
Closed Access
Fatemeh Mobasheri, Masoud Hosseinpoor, Ammar Yahia, et al.
Archives of Computational Methods in Engineering (2025)
Closed Access
A Novel Method for the Estimation of the Elastic Modulus of Ultra-High Performance Concrete using Vibration Data
Huong Duong Nguyen, Samir Khatir, Quoc Bao Nguyen
Engineering Technology & Applied Science Research (2024) Vol. 14, Iss. 4, pp. 15447-15453
Open Access | Times Cited: 3
Huong Duong Nguyen, Samir Khatir, Quoc Bao Nguyen
Engineering Technology & Applied Science Research (2024) Vol. 14, Iss. 4, pp. 15447-15453
Open Access | Times Cited: 3
Data-Driven Prediction of Maximum Settlement in Pipe Piles under Seismic Loads
Sajjad E. Rasheed, Duaa Al-Jeznawi, Musab Aied Qissab Al‐Janabi, et al.
Journal of Marine Science and Engineering (2024) Vol. 12, Iss. 2, pp. 274-274
Open Access | Times Cited: 2
Sajjad E. Rasheed, Duaa Al-Jeznawi, Musab Aied Qissab Al‐Janabi, et al.
Journal of Marine Science and Engineering (2024) Vol. 12, Iss. 2, pp. 274-274
Open Access | Times Cited: 2
Prediction of compressive strength of concrete for high-performance concrete using two combined models, SVR-AVOA and SVR-SSA
Baorong Ding, Qiong Wang, Yue Ma, et al.
Multiscale and Multidisciplinary Modeling Experiments and Design (2023) Vol. 7, Iss. 2, pp. 961-974
Closed Access | Times Cited: 4
Baorong Ding, Qiong Wang, Yue Ma, et al.
Multiscale and Multidisciplinary Modeling Experiments and Design (2023) Vol. 7, Iss. 2, pp. 961-974
Closed Access | Times Cited: 4
Eco-Transformation of construction: Harnessing machine learning and SHAP for crumb rubber concrete sustainability
Nudrat Habib, Muhammad Saqib, Taoufik Najeh, et al.
Heliyon (2024) Vol. 10, Iss. 5, pp. e26927-e26927
Open Access | Times Cited: 1
Nudrat Habib, Muhammad Saqib, Taoufik Najeh, et al.
Heliyon (2024) Vol. 10, Iss. 5, pp. e26927-e26927
Open Access | Times Cited: 1
Investigation of mechanical properties of high-performance concrete via multi-method of regression tree approach
Rui Qi, Haiyan Wu, Yongjun Qi, et al.
Materials Today Communications (2024) Vol. 40, pp. 109922-109922
Closed Access | Times Cited: 1
Rui Qi, Haiyan Wu, Yongjun Qi, et al.
Materials Today Communications (2024) Vol. 40, pp. 109922-109922
Closed Access | Times Cited: 1
Prediction of time-dependent concrete mechanical properties based on advanced deep learning models considering complex variables
Yu Jiang, Jinhao Zhang, Wenqiang Zuo, et al.
Case Studies in Construction Materials (2024) Vol. 21, pp. e03629-e03629
Open Access | Times Cited: 1
Yu Jiang, Jinhao Zhang, Wenqiang Zuo, et al.
Case Studies in Construction Materials (2024) Vol. 21, pp. e03629-e03629
Open Access | Times Cited: 1
Predicting slump for high‐performance concrete using decision tree and support vector regression approaches coupled with phasor particle swarm optimization algorithm
Qingmei Sun, Yu Gongping
Structural Concrete (2024) Vol. 25, Iss. 5, pp. 4103-4118
Closed Access | Times Cited: 1
Qingmei Sun, Yu Gongping
Structural Concrete (2024) Vol. 25, Iss. 5, pp. 4103-4118
Closed Access | Times Cited: 1
Developing a support vector regression model via optimization algorithms to appraise the hardness properties of high‐performance concrete
Xiaoyan Yan, Shasha Lu
Structural Concrete (2022) Vol. 24, Iss. 3, pp. 4047-4063
Closed Access | Times Cited: 5
Xiaoyan Yan, Shasha Lu
Structural Concrete (2022) Vol. 24, Iss. 3, pp. 4047-4063
Closed Access | Times Cited: 5
Estimation of elastic modulus of recycle aggregate concrete based on hybrid and ensemble‐hybrid approaches
Bing Qu
Structural Concrete (2023) Vol. 25, Iss. 2, pp. 1364-1387
Closed Access | Times Cited: 2
Bing Qu
Structural Concrete (2023) Vol. 25, Iss. 2, pp. 1364-1387
Closed Access | Times Cited: 2
The determination of the strongest attributes of high-performance concrete featuring innovative admixtures via optimal regression-based methodologies
Lianchao Zhang, Shizhong Guo, Jianwei Qi
Multiscale and Multidisciplinary Modeling Experiments and Design (2023) Vol. 7, Iss. 2, pp. 883-893
Closed Access | Times Cited: 1
Lianchao Zhang, Shizhong Guo, Jianwei Qi
Multiscale and Multidisciplinary Modeling Experiments and Design (2023) Vol. 7, Iss. 2, pp. 883-893
Closed Access | Times Cited: 1
Hybrid and ensemble models by coupled with automated meta-heuristic algorithms for compressive and flexural strength and slump of high-performance concrete
Mingming Zhang, Wu Qingling
Journal of Intelligent & Fuzzy Systems (2024) Vol. 46, Iss. 2, pp. 5047-5072
Closed Access
Mingming Zhang, Wu Qingling
Journal of Intelligent & Fuzzy Systems (2024) Vol. 46, Iss. 2, pp. 5047-5072
Closed Access
RETRACTED: Hyper ensembled extreme gradient boosting techniques via novel triple algorithms to appraise the hardened properties of modern ternary admixed concrete
Haiyang Shen
Journal of Intelligent & Fuzzy Systems (2024) Vol. 46, Iss. 3, pp. 5767-5782
Closed Access
Haiyang Shen
Journal of Intelligent & Fuzzy Systems (2024) Vol. 46, Iss. 3, pp. 5767-5782
Closed Access
Auto‐regulated radial base function structure implementation in the hybrid and ensemble hybrid domains to assess the hardened properties of novel mixture high performance concrete
Lei Yang
Structural Concrete (2024) Vol. 25, Iss. 5, pp. 3804-3823
Closed Access
Lei Yang
Structural Concrete (2024) Vol. 25, Iss. 5, pp. 3804-3823
Closed Access