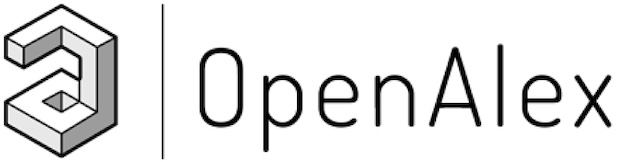
OpenAlex is a bibliographic catalogue of scientific papers, authors and institutions accessible in open access mode, named after the Library of Alexandria. It's citation coverage is excellent and I hope you will find utility in this listing of citing articles!
If you click the article title, you'll navigate to the article, as listed in CrossRef. If you click the Open Access links, you'll navigate to the "best Open Access location". Clicking the citation count will open this listing for that article. Lastly at the bottom of the page, you'll find basic pagination options.
Requested Article:
Tool wear prediction in turning using workpiece surface profile images and deep learning neural networks
Meng Lip Lim, Mohd Naqib Derani, Mani Maran Ratnam, et al.
The International Journal of Advanced Manufacturing Technology (2022) Vol. 120, Iss. 11-12, pp. 8045-8062
Closed Access | Times Cited: 14
Meng Lip Lim, Mohd Naqib Derani, Mani Maran Ratnam, et al.
The International Journal of Advanced Manufacturing Technology (2022) Vol. 120, Iss. 11-12, pp. 8045-8062
Closed Access | Times Cited: 14
Showing 14 citing articles:
State-of-art, challenges, and outlook on deep hole boring: chatter suppression, tool wear monitoring, and error measurement
Jiefu Sun, Chao Sun, Zheping Yan, et al.
The International Journal of Advanced Manufacturing Technology (2025)
Closed Access | Times Cited: 2
Jiefu Sun, Chao Sun, Zheping Yan, et al.
The International Journal of Advanced Manufacturing Technology (2025)
Closed Access | Times Cited: 2
A steps-ahead tool wear prediction method based on support vector regression and particle filtering
Yuxiong Li, Xianzhen Huang, Jiwu Tang, et al.
Measurement (2023) Vol. 218, pp. 113237-113237
Closed Access | Times Cited: 37
Yuxiong Li, Xianzhen Huang, Jiwu Tang, et al.
Measurement (2023) Vol. 218, pp. 113237-113237
Closed Access | Times Cited: 37
Surface roughness and tool wear monitoring in turning processes through vibration analysis using PSD and GRMS
Roumaissa Bouchama, Mohamed Lamine Bouhalais, Abdelhakim Cherfia
The International Journal of Advanced Manufacturing Technology (2024) Vol. 130, Iss. 7-8, pp. 3537-3552
Closed Access | Times Cited: 7
Roumaissa Bouchama, Mohamed Lamine Bouhalais, Abdelhakim Cherfia
The International Journal of Advanced Manufacturing Technology (2024) Vol. 130, Iss. 7-8, pp. 3537-3552
Closed Access | Times Cited: 7
Tool Condition Monitoring Methods Applicable in the Metalworking Process
Melvin Alexis Lara de León, Jakub Kolařík, Radek Byrtus, et al.
Archives of Computational Methods in Engineering (2023) Vol. 31, Iss. 1, pp. 221-242
Open Access | Times Cited: 15
Melvin Alexis Lara de León, Jakub Kolařík, Radek Byrtus, et al.
Archives of Computational Methods in Engineering (2023) Vol. 31, Iss. 1, pp. 221-242
Open Access | Times Cited: 15
Deep learning-based CNC milling tool wear stage estimation with multi-signal analysis
Yunus Emre Karabacak
Eksploatacja i Niezawodnosc - Maintenance and Reliability (2023) Vol. 25, Iss. 3
Open Access | Times Cited: 14
Yunus Emre Karabacak
Eksploatacja i Niezawodnosc - Maintenance and Reliability (2023) Vol. 25, Iss. 3
Open Access | Times Cited: 14
Development of Weighted Ensemble Deep Learning Network for Surface Roughness Prediction and Flank Wear Measurement
Ahmed Alhussen, N. Vinoth, M. R. Archana Jenis, et al.
Journal of Materials Engineering and Performance (2024)
Closed Access | Times Cited: 4
Ahmed Alhussen, N. Vinoth, M. R. Archana Jenis, et al.
Journal of Materials Engineering and Performance (2024)
Closed Access | Times Cited: 4
Research on Tool Remaining Life Prediction Method Based on CNN-LSTM-PSO
Shuo Wang, Zhenliang Yu, G. Xu, et al.
IEEE Access (2023) Vol. 11, pp. 80448-80464
Open Access | Times Cited: 9
Shuo Wang, Zhenliang Yu, G. Xu, et al.
IEEE Access (2023) Vol. 11, pp. 80448-80464
Open Access | Times Cited: 9
A Generative AI approach to improve in-situ vision tool wear monitoring with scarce data
Alberto García-Pérez, María J. Gómez-Silva, Arturo de la Escalera-Hueso
Journal of Intelligent Manufacturing (2024)
Closed Access | Times Cited: 2
Alberto García-Pérez, María J. Gómez-Silva, Arturo de la Escalera-Hueso
Journal of Intelligent Manufacturing (2024)
Closed Access | Times Cited: 2
Genetic algorithm-based error correction algorithm for CNC turning machining of mechanical parts
Qinghong Xue, Ying Miao, Zijian Xue
Journal of Measurements in Engineering (2023) Vol. 11, Iss. 4, pp. 509-524
Open Access | Times Cited: 2
Qinghong Xue, Ying Miao, Zijian Xue
Journal of Measurements in Engineering (2023) Vol. 11, Iss. 4, pp. 509-524
Open Access | Times Cited: 2
Predicting Tool Wear with ParaCRN-AMResNet: A Hybrid Deep Learning Approach
Lian Guo, Yongguo Wang
Machines (2024) Vol. 12, Iss. 5, pp. 341-341
Open Access
Lian Guo, Yongguo Wang
Machines (2024) Vol. 12, Iss. 5, pp. 341-341
Open Access
Cloud maps highlighting dynamic characteristics of surface signal to improve time-varying wear evaluation accuracy
Hongju Li, Ying Liu, Haoran Liao
Measurement (2024) Vol. 236, pp. 115089-115089
Closed Access
Hongju Li, Ying Liu, Haoran Liao
Measurement (2024) Vol. 236, pp. 115089-115089
Closed Access
Sustainable Manufacturing Through Materials Machining Innovations—A Review
Ainur Munira Rosli, Ahmad Shahir Jamaludin, Mohd Nizar Mhd Razali, et al.
Lecture notes in mechanical engineering (2024), pp. 665-674
Closed Access
Ainur Munira Rosli, Ahmad Shahir Jamaludin, Mohd Nizar Mhd Razali, et al.
Lecture notes in mechanical engineering (2024), pp. 665-674
Closed Access
Innovative High-Speed Machining of Aerospace Alloys with Cutting-Edge Thermal Distortion Control—A Review
Muhammad Irfan Khairil Asli, Ahmad Shahir Jamaludin, Mohd Nizar Mhd Razali, et al.
Lecture notes in mechanical engineering (2024), pp. 351-359
Closed Access
Muhammad Irfan Khairil Asli, Ahmad Shahir Jamaludin, Mohd Nizar Mhd Razali, et al.
Lecture notes in mechanical engineering (2024), pp. 351-359
Closed Access
A CNN-LSTM-PSO tool wear prediction method based on multi-channel feature fusion
Shuo Wang, Zhenliang YU, Yongqi GUO, et al.
Mechanical Engineering Science (2022) Vol. 4, Iss. 2, pp. 39-39
Open Access | Times Cited: 1
Shuo Wang, Zhenliang YU, Yongqi GUO, et al.
Mechanical Engineering Science (2022) Vol. 4, Iss. 2, pp. 39-39
Open Access | Times Cited: 1