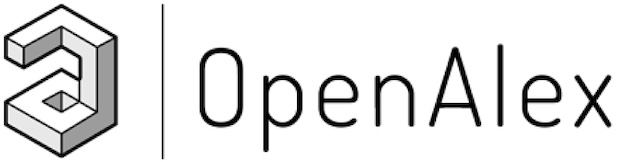
OpenAlex is a bibliographic catalogue of scientific papers, authors and institutions accessible in open access mode, named after the Library of Alexandria. It's citation coverage is excellent and I hope you will find utility in this listing of citing articles!
If you click the article title, you'll navigate to the article, as listed in CrossRef. If you click the Open Access links, you'll navigate to the "best Open Access location". Clicking the citation count will open this listing for that article. Lastly at the bottom of the page, you'll find basic pagination options.
Requested Article:
Machine learning to predict retention time of small molecules in nano-HPLC
Sergey Osipenko, Inga Bashkirova, Sergey Sosnin, et al.
Analytical and Bioanalytical Chemistry (2020) Vol. 412, Iss. 28, pp. 7767-7776
Closed Access | Times Cited: 38
Sergey Osipenko, Inga Bashkirova, Sergey Sosnin, et al.
Analytical and Bioanalytical Chemistry (2020) Vol. 412, Iss. 28, pp. 7767-7776
Closed Access | Times Cited: 38
Showing 1-25 of 38 citing articles:
Explicit relation between thin film chromatography and column chromatography conditions from statistics and machine learning
Hao Xu, Wenchao Wu, Yuntian Chen, et al.
Nature Communications (2025) Vol. 16, Iss. 1
Open Access | Times Cited: 2
Hao Xu, Wenchao Wu, Yuntian Chen, et al.
Nature Communications (2025) Vol. 16, Iss. 1
Open Access | Times Cited: 2
Retention time prediction for chromatographic enantioseparation by quantile geometry-enhanced graph neural network
Hao Xu, Jinglong Lin, Dongxiao Zhang, et al.
Nature Communications (2023) Vol. 14, Iss. 1
Open Access | Times Cited: 34
Hao Xu, Jinglong Lin, Dongxiao Zhang, et al.
Nature Communications (2023) Vol. 14, Iss. 1
Open Access | Times Cited: 34
Prediction of Retention Indices in LC-HRMS for Enhanced Structural Identification of Organic Micropollutants in Water: Selectivity-Based Filtration
Ardiana Kajtazi, Marin Kajtazi, Maike Felipe Santos Barbetta, et al.
Analytical Chemistry (2025) Vol. 97, Iss. 1, pp. 65-74
Closed Access | Times Cited: 1
Ardiana Kajtazi, Marin Kajtazi, Maike Felipe Santos Barbetta, et al.
Analytical Chemistry (2025) Vol. 97, Iss. 1, pp. 65-74
Closed Access | Times Cited: 1
From Reverse Phase Chromatography to HILIC: Graph Transformers Power Method-Independent Machine Learning of Retention Times
Cailum M. K. Stienstra, Emir Nazdrajić, W. Scott Hopkins
Analytical Chemistry (2025)
Closed Access | Times Cited: 1
Cailum M. K. Stienstra, Emir Nazdrajić, W. Scott Hopkins
Analytical Chemistry (2025)
Closed Access | Times Cited: 1
Deep learning for retention time prediction in reversed-phase liquid chromatography
E. S. Fedorova, Dmitriy D. Matyushin, I. V. Plyushchenko, et al.
Journal of Chromatography A (2021) Vol. 1664, pp. 462792-462792
Closed Access | Times Cited: 53
E. S. Fedorova, Dmitriy D. Matyushin, I. V. Plyushchenko, et al.
Journal of Chromatography A (2021) Vol. 1664, pp. 462792-462792
Closed Access | Times Cited: 53
Retention Time Prediction with Message-Passing Neural Networks
Sergey Osipenko, Е. Н. Николаев, Yury Kostyukevich
Separations (2022) Vol. 9, Iss. 10, pp. 291-291
Open Access | Times Cited: 31
Sergey Osipenko, Е. Н. Николаев, Yury Kostyukevich
Separations (2022) Vol. 9, Iss. 10, pp. 291-291
Open Access | Times Cited: 31
Generic and accurate prediction of retention times in liquid chromatography by post–projection calibration
Yan Zhang, Fei Liu, Xiu Qin Li, et al.
Communications Chemistry (2024) Vol. 7, Iss. 1
Open Access | Times Cited: 7
Yan Zhang, Fei Liu, Xiu Qin Li, et al.
Communications Chemistry (2024) Vol. 7, Iss. 1
Open Access | Times Cited: 7
Performance and robustness of small molecule retention time prediction with molecular graph neural networks in industrial drug discovery campaigns
Daniel Vik, David Pii, Chirag Mudaliar, et al.
Scientific Reports (2024) Vol. 14, Iss. 1
Open Access | Times Cited: 7
Daniel Vik, David Pii, Chirag Mudaliar, et al.
Scientific Reports (2024) Vol. 14, Iss. 1
Open Access | Times Cited: 7
Strategies for structure elucidation of small molecules based on LC–MS/MS data from complex biological samples
Zhitao Tian, Fangzhou Liu, Dongqin Li, et al.
Computational and Structural Biotechnology Journal (2022) Vol. 20, pp. 5085-5097
Open Access | Times Cited: 24
Zhitao Tian, Fangzhou Liu, Dongqin Li, et al.
Computational and Structural Biotechnology Journal (2022) Vol. 20, pp. 5085-5097
Open Access | Times Cited: 24
Deep Neural Network Pretrained by Weighted Autoencoders and Transfer Learning for Retention Time Prediction of Small Molecules
Ran Ju, Xinyu Liu, Fujian Zheng, et al.
Analytical Chemistry (2021) Vol. 93, Iss. 47, pp. 15651-15658
Closed Access | Times Cited: 31
Ran Ju, Xinyu Liu, Fujian Zheng, et al.
Analytical Chemistry (2021) Vol. 93, Iss. 47, pp. 15651-15658
Closed Access | Times Cited: 31
Probabilistic metabolite annotation using retention time prediction and meta-learned projections
Constantino Antonio García Martínez, Alberto Gil-de-la-Fuente, Coral Barbas, et al.
Journal of Cheminformatics (2022) Vol. 14, Iss. 1
Open Access | Times Cited: 20
Constantino Antonio García Martínez, Alberto Gil-de-la-Fuente, Coral Barbas, et al.
Journal of Cheminformatics (2022) Vol. 14, Iss. 1
Open Access | Times Cited: 20
A comparative study of the predictive performance of different descriptor calculation tools: Molecular-based elution order modeling and interpretation of retention mechanism for isomeric compounds from METLIN database
Darija Obradović, А. Н. Ставрианиди, E. S. Fedorova, et al.
Journal of Chromatography A (2024) Vol. 1719, pp. 464731-464731
Closed Access | Times Cited: 4
Darija Obradović, А. Н. Ставрианиди, E. S. Fedorova, et al.
Journal of Chromatography A (2024) Vol. 1719, pp. 464731-464731
Closed Access | Times Cited: 4
Revealing the underestimated risks of pharmaceutical transformation products in wastewater by suspect and targeted screening
Gang Wu, Yuli Qian, Chunqiu Zhang, et al.
Water Research (2025) Vol. 276, pp. 123265-123265
Closed Access
Gang Wu, Yuli Qian, Chunqiu Zhang, et al.
Water Research (2025) Vol. 276, pp. 123265-123265
Closed Access
In Silico High-Performance Liquid Chromatography Method Development via Machine Learning
Alberto Marchetto, Monica Tirapelle, Luca Mazzei, et al.
Analytical Chemistry (2025)
Open Access
Alberto Marchetto, Monica Tirapelle, Luca Mazzei, et al.
Analytical Chemistry (2025)
Open Access
Recent developments and workflows of non-targeted LC-HRMS screening in the detection of veterinary drug residues in foods
Yuting Yang, Jiaxi Chen, Guiyuan Li, et al.
TrAC Trends in Analytical Chemistry (2025), pp. 118314-118314
Closed Access
Yuting Yang, Jiaxi Chen, Guiyuan Li, et al.
TrAC Trends in Analytical Chemistry (2025), pp. 118314-118314
Closed Access
Understanding the coordination behavior of antibiotics: Take tetracycline as an example
Qiandi Wang, Qiongying Xu, Siyuan Zhai, et al.
Journal of Hazardous Materials (2023) Vol. 460, pp. 132375-132375
Closed Access | Times Cited: 9
Qiandi Wang, Qiongying Xu, Siyuan Zhai, et al.
Journal of Hazardous Materials (2023) Vol. 460, pp. 132375-132375
Closed Access | Times Cited: 9
Cross-column density functional theory–based quantitative structure-retention relationship model development powered by machine learning
Sargol Mazraedoost, Petar Žuvela, Szymon Ulenberg, et al.
Analytical and Bioanalytical Chemistry (2024) Vol. 416, Iss. 12, pp. 2951-2968
Closed Access | Times Cited: 3
Sargol Mazraedoost, Petar Žuvela, Szymon Ulenberg, et al.
Analytical and Bioanalytical Chemistry (2024) Vol. 416, Iss. 12, pp. 2951-2968
Closed Access | Times Cited: 3
Retention Time Prediction in High-Performance Liquid Chromatography Using Random Forest Regression
V. Selvi, Anirudh R Urs, T. O. B.
Research Square (Research Square) (2025)
Closed Access
V. Selvi, Anirudh R Urs, T. O. B.
Research Square (Research Square) (2025)
Closed Access
Retention Time Prediction through Learning from a Small Training Data Set with a Pretrained Graph Neural Network
Youngchun Kwon, Hyukju Kwon, Jongmin Han, et al.
Analytical Chemistry (2023) Vol. 95, Iss. 47, pp. 17273-17283
Closed Access | Times Cited: 8
Youngchun Kwon, Hyukju Kwon, Jongmin Han, et al.
Analytical Chemistry (2023) Vol. 95, Iss. 47, pp. 17273-17283
Closed Access | Times Cited: 8
Transfer learning for small molecule retention predictions
Sergey Osipenko, Kazii Botashev, Е. Н. Николаев, et al.
Journal of Chromatography A (2021) Vol. 1644, pp. 462119-462119
Closed Access | Times Cited: 16
Sergey Osipenko, Kazii Botashev, Е. Н. Николаев, et al.
Journal of Chromatography A (2021) Vol. 1644, pp. 462119-462119
Closed Access | Times Cited: 16
ReTimeML: a retention time predictor that supports the LC–MS/MS analysis of sphingolipids
Michael Allwright, Boris Guennewig, Anna Hoffmann, et al.
Scientific Reports (2024) Vol. 14, Iss. 1
Open Access | Times Cited: 2
Michael Allwright, Boris Guennewig, Anna Hoffmann, et al.
Scientific Reports (2024) Vol. 14, Iss. 1
Open Access | Times Cited: 2
Recent advances in modelling and control of liquid chromatography
Maximilian O. Besenhard, A. Tsatse, Luca Mazzei, et al.
Current Opinion in Chemical Engineering (2021) Vol. 32, pp. 100685-100685
Open Access | Times Cited: 13
Maximilian O. Besenhard, A. Tsatse, Luca Mazzei, et al.
Current Opinion in Chemical Engineering (2021) Vol. 32, pp. 100685-100685
Open Access | Times Cited: 13
3D chemical structures allow robust deep learning models for retention time prediction
Mark Zaretckii, Inga Bashkirova, Sergey Osipenko, et al.
Digital Discovery (2022) Vol. 1, Iss. 5, pp. 711-718
Open Access | Times Cited: 9
Mark Zaretckii, Inga Bashkirova, Sergey Osipenko, et al.
Digital Discovery (2022) Vol. 1, Iss. 5, pp. 711-718
Open Access | Times Cited: 9
Oxygen Isotope Exchange Reaction for Untargeted LC–MS Analysis
Sergey Osipenko, Alexander Zherebker, Lidiia Rumiantseva, et al.
Journal of the American Society for Mass Spectrometry (2022) Vol. 33, Iss. 2, pp. 390-398
Closed Access | Times Cited: 8
Sergey Osipenko, Alexander Zherebker, Lidiia Rumiantseva, et al.
Journal of the American Society for Mass Spectrometry (2022) Vol. 33, Iss. 2, pp. 390-398
Closed Access | Times Cited: 8
Training Deep Learning Neural Networks for Predicting CCS Using the METLIN-CCS Dataset
Guillermo Ramajo, Constantino Antonio García Martínez, Alberto Gil-de-la-Fuente, et al.
Lecture notes in computer science (2024), pp. 225-236
Closed Access | Times Cited: 1
Guillermo Ramajo, Constantino Antonio García Martínez, Alberto Gil-de-la-Fuente, et al.
Lecture notes in computer science (2024), pp. 225-236
Closed Access | Times Cited: 1