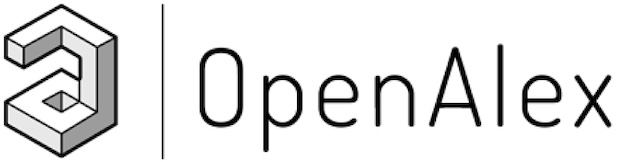
OpenAlex is a bibliographic catalogue of scientific papers, authors and institutions accessible in open access mode, named after the Library of Alexandria. It's citation coverage is excellent and I hope you will find utility in this listing of citing articles!
If you click the article title, you'll navigate to the article, as listed in CrossRef. If you click the Open Access links, you'll navigate to the "best Open Access location". Clicking the citation count will open this listing for that article. Lastly at the bottom of the page, you'll find basic pagination options.
Requested Article:
Spatial modeling of susceptibility to subsidence using machine learning techniques
Majid Mohammady, Hamid Reza Pourghasemi, Mojtaba Amiri, et al.
Stochastic Environmental Research and Risk Assessment (2021)
Closed Access | Times Cited: 28
Majid Mohammady, Hamid Reza Pourghasemi, Mojtaba Amiri, et al.
Stochastic Environmental Research and Risk Assessment (2021)
Closed Access | Times Cited: 28
Showing 1-25 of 28 citing articles:
Explainable Sinkhole Susceptibility Mapping Using Machine-Learning-Based SHAP: Quantifying and Comparing the Effects of Contributing Factors in Konya, Türkiye
Süleyman Sefa Bilgilioğlu, Cemil Gezgin, Muzaffer Can İban, et al.
Applied Sciences (2025) Vol. 15, Iss. 6, pp. 3139-3139
Open Access | Times Cited: 1
Süleyman Sefa Bilgilioğlu, Cemil Gezgin, Muzaffer Can İban, et al.
Applied Sciences (2025) Vol. 15, Iss. 6, pp. 3139-3139
Open Access | Times Cited: 1
An integrated InSAR-machine learning approach for ground deformation rate modeling in arid areas
Seyed Amir Naghibi, Behshid Khodaei, Hossein Hashemi
Journal of Hydrology (2022) Vol. 608, pp. 127627-127627
Open Access | Times Cited: 30
Seyed Amir Naghibi, Behshid Khodaei, Hossein Hashemi
Journal of Hydrology (2022) Vol. 608, pp. 127627-127627
Open Access | Times Cited: 30
Prediction of mining induced subsidence by sparrow search algorithm with extreme gradient boosting and TOPSIS method
Chun Xu, Keping Zhou, Xin Xiong, et al.
Acta Geotechnica (2023) Vol. 18, Iss. 9, pp. 4993-5009
Closed Access | Times Cited: 14
Chun Xu, Keping Zhou, Xin Xiong, et al.
Acta Geotechnica (2023) Vol. 18, Iss. 9, pp. 4993-5009
Closed Access | Times Cited: 14
Application of hybrid model-based machine learning for groundwater potential prediction in the north central of Vietnam
Huu Duy Nguyen, Van Hong Nguyen, Quan Vu Viet Du, et al.
Earth Science Informatics (2024) Vol. 17, Iss. 2, pp. 1569-1589
Closed Access | Times Cited: 6
Huu Duy Nguyen, Van Hong Nguyen, Quan Vu Viet Du, et al.
Earth Science Informatics (2024) Vol. 17, Iss. 2, pp. 1569-1589
Closed Access | Times Cited: 6
Large-Scale Land Subsidence Monitoring and Prediction Based on SBAS-InSAR Technology with Time-Series Sentinel-1A Satellite Data
Hengliang Guo, Yonghao Yuan, Jinyang Wang, et al.
Remote Sensing (2023) Vol. 15, Iss. 11, pp. 2843-2843
Open Access | Times Cited: 12
Hengliang Guo, Yonghao Yuan, Jinyang Wang, et al.
Remote Sensing (2023) Vol. 15, Iss. 11, pp. 2843-2843
Open Access | Times Cited: 12
Enhancing a convolutional neural network model for land subsidence susceptibility mapping using hybrid meta-heuristic algorithms
Ali Asghar Jafari, Ali Asghar Alesheikh, Fatemeh Rezaie, et al.
International Journal of Coal Geology (2023) Vol. 277, pp. 104350-104350
Closed Access | Times Cited: 12
Ali Asghar Jafari, Ali Asghar Alesheikh, Fatemeh Rezaie, et al.
International Journal of Coal Geology (2023) Vol. 277, pp. 104350-104350
Closed Access | Times Cited: 12
Aquifer and Land Subsidence Interaction Assessment Using Sentinel-1 Data and DInSAR Technique
Fatemeh Rafiei, Saeid Gharechelou, Saeed Golian, et al.
ISPRS International Journal of Geo-Information (2022) Vol. 11, Iss. 9, pp. 495-495
Open Access | Times Cited: 17
Fatemeh Rafiei, Saeid Gharechelou, Saeed Golian, et al.
ISPRS International Journal of Geo-Information (2022) Vol. 11, Iss. 9, pp. 495-495
Open Access | Times Cited: 17
Vegetation vulnerability to hydrometeorological stresses in water-scarce areas using machine learning and remote sensing techniques
Ehsan Moradi, Hamid Darabi, Esmail Heydari Alamdarloo, et al.
Ecological Informatics (2022) Vol. 73, pp. 101838-101838
Open Access | Times Cited: 17
Ehsan Moradi, Hamid Darabi, Esmail Heydari Alamdarloo, et al.
Ecological Informatics (2022) Vol. 73, pp. 101838-101838
Open Access | Times Cited: 17
An Assessment of Different Line-of-Sight and Ground Velocity Distributions for a Comprehensive Understanding of Ground Deformation Patterns in East Jharia Coalfield
Aditya Kumar Thakur, Rahul Garg, Kamal Jain
Remote Sensing Applications Society and Environment (2025), pp. 101446-101446
Closed Access
Aditya Kumar Thakur, Rahul Garg, Kamal Jain
Remote Sensing Applications Society and Environment (2025), pp. 101446-101446
Closed Access
Wildfire susceptibility mapping using two empowered machine learning algorithms
Hossein Moayedi, Mohammad Ali Salehi Amin Khasmakhi
Stochastic Environmental Research and Risk Assessment (2022) Vol. 37, Iss. 1, pp. 49-72
Closed Access | Times Cited: 16
Hossein Moayedi, Mohammad Ali Salehi Amin Khasmakhi
Stochastic Environmental Research and Risk Assessment (2022) Vol. 37, Iss. 1, pp. 49-72
Closed Access | Times Cited: 16
Theoretical Analysis of Mining Induced Overburden Subsidence Boundary with the Horizontal Coal Seam Mining
Weitao Yan, Junjie Chen, Yi Tan, et al.
Advances in Civil Engineering (2021) Vol. 2021, Iss. 1
Open Access | Times Cited: 17
Weitao Yan, Junjie Chen, Yi Tan, et al.
Advances in Civil Engineering (2021) Vol. 2021, Iss. 1
Open Access | Times Cited: 17
Assessment of coal mining land subsidence by using an innovative comprehensive weighted cloud model combined with a PSR conceptual model
Chun Xu, Keping Zhou, Xin Xiong, et al.
Environmental Science and Pollution Research (2021) Vol. 29, Iss. 13, pp. 18665-18679
Closed Access | Times Cited: 11
Chun Xu, Keping Zhou, Xin Xiong, et al.
Environmental Science and Pollution Research (2021) Vol. 29, Iss. 13, pp. 18665-18679
Closed Access | Times Cited: 11
Evaluating Machine Learning-Based Approaches in Land Subsidence Susceptibility Mapping
Elham Hosseinzadeh, Sara Anamaghi, Massoud Behboudian, et al.
Land (2024) Vol. 13, Iss. 3, pp. 322-322
Open Access | Times Cited: 1
Elham Hosseinzadeh, Sara Anamaghi, Massoud Behboudian, et al.
Land (2024) Vol. 13, Iss. 3, pp. 322-322
Open Access | Times Cited: 1
COVID-19 mortality analysis from soft-data multivariate curve regression and machine learning
A. Torres-Signes, María Pilar Frías, M. D. Ruiz‐Medina
Stochastic Environmental Research and Risk Assessment (2021) Vol. 35, Iss. 12, pp. 2659-2678
Open Access | Times Cited: 9
A. Torres-Signes, María Pilar Frías, M. D. Ruiz‐Medina
Stochastic Environmental Research and Risk Assessment (2021) Vol. 35, Iss. 12, pp. 2659-2678
Open Access | Times Cited: 9
Land-subsidence susceptibility mapping: assessment of an adaptive neuro-fuzzy inference system–genetic algorithm hybrid model
Tang Wen, Wang Tiewang, Alireza Arabameri, et al.
Geocarto International (2022) Vol. 37, Iss. 26, pp. 12194-12218
Closed Access | Times Cited: 6
Tang Wen, Wang Tiewang, Alireza Arabameri, et al.
Geocarto International (2022) Vol. 37, Iss. 26, pp. 12194-12218
Closed Access | Times Cited: 6
Research on the Prediction Model of Mine Subsidence Based on Object-Oriented and Probability Integration Method
Zhongyuan Gu, Yanlin Zhao, Rugao Gao, et al.
Geofluids (2022) Vol. 2022, pp. 1-14
Open Access | Times Cited: 6
Zhongyuan Gu, Yanlin Zhao, Rugao Gao, et al.
Geofluids (2022) Vol. 2022, pp. 1-14
Open Access | Times Cited: 6
Badland erosion susceptibility mapping using machine learning data mining techniques, Firozkuh watershed, Iran
Majid Mohammady
Natural Hazards (2023) Vol. 117, Iss. 1, pp. 703-721
Open Access | Times Cited: 3
Majid Mohammady
Natural Hazards (2023) Vol. 117, Iss. 1, pp. 703-721
Open Access | Times Cited: 3
Gully Erosion Susceptibility Assessment Using Different Machine Learning Algorithms: A Case Study of Shazand Watershed in Iran
Majid Mohammady, Aliakbar Davudirad
Environmental Modeling & Assessment (2023) Vol. 29, Iss. 2, pp. 249-261
Open Access | Times Cited: 3
Majid Mohammady, Aliakbar Davudirad
Environmental Modeling & Assessment (2023) Vol. 29, Iss. 2, pp. 249-261
Open Access | Times Cited: 3
A Discrete-Time Model-Based Method for Predicting Settlement of Geotechnical Foundations in Buildings
Chengwei Yang, Zhenzhen Jiang
Mobile Information Systems (2022) Vol. 2022, pp. 1-7
Open Access | Times Cited: 4
Chengwei Yang, Zhenzhen Jiang
Mobile Information Systems (2022) Vol. 2022, pp. 1-7
Open Access | Times Cited: 4
A Wide Scale Survey on Weather Prediction Using Machine Learning Techniques
Shabnam Kumari, P. Muthulakshmi
Journal of Information & Knowledge Management (2022) Vol. 22, Iss. 05
Closed Access | Times Cited: 3
Shabnam Kumari, P. Muthulakshmi
Journal of Information & Knowledge Management (2022) Vol. 22, Iss. 05
Closed Access | Times Cited: 3
Analyzing Landslide Susceptibility of Forest Roads by Analytical Hierarchy Process (AHP) in Of Forest Planning Unit of Turkiye
Derya Mumcu Küçüker
Research Square (Research Square) (2023)
Open Access | Times Cited: 1
Derya Mumcu Küçüker
Research Square (Research Square) (2023)
Open Access | Times Cited: 1
Analyzing landslide susceptibility of forest roads by analytical hierarchy process (AHP) in of forest planning unit of Turkiye
Derya Mumcu Küçüker
Natural Hazards (2024)
Closed Access
Derya Mumcu Küçüker
Natural Hazards (2024)
Closed Access
Identification of land subsidence prone areas and their mapping using machine learning algorithms
Zeynab Najafi, Hamid Reza Pourghasemi, Gholamabbas Ghanbarian, et al.
Elsevier eBooks (2021), pp. 535-545
Closed Access | Times Cited: 3
Zeynab Najafi, Hamid Reza Pourghasemi, Gholamabbas Ghanbarian, et al.
Elsevier eBooks (2021), pp. 535-545
Closed Access | Times Cited: 3
Assessment of alternative forest road routes and landslide susceptibility mapping using machine learning
Ender Buğday, Abdullah E. Akay
CERNE (2022) Vol. 28
Open Access | Times Cited: 2
Ender Buğday, Abdullah E. Akay
CERNE (2022) Vol. 28
Open Access | Times Cited: 2
Badland erosion susceptibility mapping using machine-learning data mining techniques, Firozkuh watershed, Iran
Majid Mohammady
Research Square (Research Square) (2022)
Open Access | Times Cited: 1
Majid Mohammady
Research Square (Research Square) (2022)
Open Access | Times Cited: 1