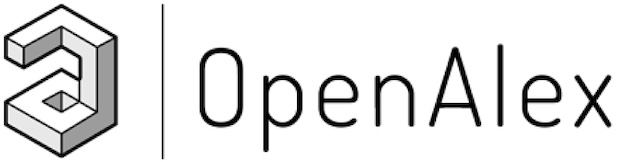
OpenAlex is a bibliographic catalogue of scientific papers, authors and institutions accessible in open access mode, named after the Library of Alexandria. It's citation coverage is excellent and I hope you will find utility in this listing of citing articles!
If you click the article title, you'll navigate to the article, as listed in CrossRef. If you click the Open Access links, you'll navigate to the "best Open Access location". Clicking the citation count will open this listing for that article. Lastly at the bottom of the page, you'll find basic pagination options.
Requested Article:
Machine learning-accelerated quantum mechanics-based atomistic simulations for industrial applications
Tobias Morawietz, Nongnuch Artrith
Journal of Computer-Aided Molecular Design (2020) Vol. 35, Iss. 4, pp. 557-586
Open Access | Times Cited: 49
Tobias Morawietz, Nongnuch Artrith
Journal of Computer-Aided Molecular Design (2020) Vol. 35, Iss. 4, pp. 557-586
Open Access | Times Cited: 49
Showing 1-25 of 49 citing articles:
Machine-learning interatomic potentials for materials science
Y. Mishin
Acta Materialia (2021) Vol. 214, pp. 116980-116980
Open Access | Times Cited: 225
Y. Mishin
Acta Materialia (2021) Vol. 214, pp. 116980-116980
Open Access | Times Cited: 225
Opportunities of Flexible and Portable Electrochemical Devices for Energy Storage: Expanding the Spotlight onto Semi-solid/Solid Electrolytes
Xiayue Fan, Cheng Zhong, Jie Liu, et al.
Chemical Reviews (2022) Vol. 122, Iss. 23, pp. 17155-17239
Closed Access | Times Cited: 167
Xiayue Fan, Cheng Zhong, Jie Liu, et al.
Chemical Reviews (2022) Vol. 122, Iss. 23, pp. 17155-17239
Closed Access | Times Cited: 167
Modeling Operando Electrochemical CO2 Reduction
Federico Dattila, Ranga Rohit Seemakurthi, Yecheng Zhou, et al.
Chemical Reviews (2022) Vol. 122, Iss. 12, pp. 11085-11130
Closed Access | Times Cited: 105
Federico Dattila, Ranga Rohit Seemakurthi, Yecheng Zhou, et al.
Chemical Reviews (2022) Vol. 122, Iss. 12, pp. 11085-11130
Closed Access | Times Cited: 105
Transition metal nanoparticles as nanocatalysts for Suzuki, Heck and Sonogashira cross-coupling reactions
Muhammad Ashraf, Muhammad Sohail Ahmad, Yusuke Inomata, et al.
Coordination Chemistry Reviews (2022) Vol. 476, pp. 214928-214928
Closed Access | Times Cited: 82
Muhammad Ashraf, Muhammad Sohail Ahmad, Yusuke Inomata, et al.
Coordination Chemistry Reviews (2022) Vol. 476, pp. 214928-214928
Closed Access | Times Cited: 82
Operando Characterization of Organic Mixed Ionic/Electronic Conducting Materials
Ruiheng Wu, Micaela Matta, Bryan D. Paulsen, et al.
Chemical Reviews (2022) Vol. 122, Iss. 4, pp. 4493-4551
Open Access | Times Cited: 74
Ruiheng Wu, Micaela Matta, Bryan D. Paulsen, et al.
Chemical Reviews (2022) Vol. 122, Iss. 4, pp. 4493-4551
Open Access | Times Cited: 74
The Local Vibrational Mode Theory and Its Place in the Vibrational Spectroscopy Arena
Elfi Kraka, Mateus Quintano, Hunter La Force, et al.
The Journal of Physical Chemistry A (2022) Vol. 126, Iss. 47, pp. 8781-8798
Closed Access | Times Cited: 58
Elfi Kraka, Mateus Quintano, Hunter La Force, et al.
The Journal of Physical Chemistry A (2022) Vol. 126, Iss. 47, pp. 8781-8798
Closed Access | Times Cited: 58
Open-Source Machine Learning in Computational Chemistry
Alexander Hagg, Karl N. Kirschner
Journal of Chemical Information and Modeling (2023) Vol. 63, Iss. 15, pp. 4505-4532
Open Access | Times Cited: 28
Alexander Hagg, Karl N. Kirschner
Journal of Chemical Information and Modeling (2023) Vol. 63, Iss. 15, pp. 4505-4532
Open Access | Times Cited: 28
Prediction of hydration energies of adsorbates at Pt(111) and liquid water interfaces using machine learning
Jiexin Shi, Xiaohong Zhang, Venkata Rohit Punyapu, et al.
The Journal of Chemical Physics (2025) Vol. 162, Iss. 8
Closed Access | Times Cited: 1
Jiexin Shi, Xiaohong Zhang, Venkata Rohit Punyapu, et al.
The Journal of Chemical Physics (2025) Vol. 162, Iss. 8
Closed Access | Times Cited: 1
Application-oriented design of machine learning paradigms for battery science
Ying Wang
npj Computational Materials (2025) Vol. 11, Iss. 1
Open Access | Times Cited: 1
Ying Wang
npj Computational Materials (2025) Vol. 11, Iss. 1
Open Access | Times Cited: 1
Predicting thermoelectric properties from chemical formula with explicitly identifying dopant effects
Gyoung S. Na, Seunghun Jang, Hyunju Chang
npj Computational Materials (2021) Vol. 7, Iss. 1
Open Access | Times Cited: 45
Gyoung S. Na, Seunghun Jang, Hyunju Chang
npj Computational Materials (2021) Vol. 7, Iss. 1
Open Access | Times Cited: 45
Unraveling the Synergistic Effect of Re and Cs Promoters on Ethylene Epoxidation over Silver Catalysts with Machine Learning-Accelerated First-Principles Simulations
Benjamin W. J. Chen, Bo Wang, Michael B. Sullivan, et al.
ACS Catalysis (2022) Vol. 12, Iss. 4, pp. 2540-2551
Closed Access | Times Cited: 31
Benjamin W. J. Chen, Bo Wang, Michael B. Sullivan, et al.
ACS Catalysis (2022) Vol. 12, Iss. 4, pp. 2540-2551
Closed Access | Times Cited: 31
A mini review on the applications of artificial intelligence (AI) in surface chemistry and catalysis
Faisal Al-Akayleh, Ahmed S.A. Ali Agha, Rami A. Abdel Rahem, et al.
Tenside Surfactants Detergents (2024) Vol. 61, Iss. 4, pp. 285-296
Closed Access | Times Cited: 8
Faisal Al-Akayleh, Ahmed S.A. Ali Agha, Rami A. Abdel Rahem, et al.
Tenside Surfactants Detergents (2024) Vol. 61, Iss. 4, pp. 285-296
Closed Access | Times Cited: 8
Deciphering the Lexicon of Protein Targets: A Review on Multifaceted Drug Discovery in the Era of Artificial Intelligence
Suvendu Nandi, Soumyadeep Bhaduri, Debraj Das, et al.
Molecular Pharmaceutics (2024) Vol. 21, Iss. 4, pp. 1563-1590
Closed Access | Times Cited: 7
Suvendu Nandi, Soumyadeep Bhaduri, Debraj Das, et al.
Molecular Pharmaceutics (2024) Vol. 21, Iss. 4, pp. 1563-1590
Closed Access | Times Cited: 7
Machine learning as a surrogate model for EnergyPLAN: Speeding up energy system optimization at the country level
Matteo Giacomo Prina, Mattia Dallapiccola, David Moser, et al.
Energy (2024) Vol. 307, pp. 132735-132735
Open Access | Times Cited: 7
Matteo Giacomo Prina, Mattia Dallapiccola, David Moser, et al.
Energy (2024) Vol. 307, pp. 132735-132735
Open Access | Times Cited: 7
Enhancing Molecular Energy Predictions with Physically Constrained Modifications to the Neural Network Potential
Weiqiang Fu, Yujie Mo, Xiao Yi, et al.
Journal of Chemical Theory and Computation (2024) Vol. 20, Iss. 11, pp. 4533-4544
Open Access | Times Cited: 5
Weiqiang Fu, Yujie Mo, Xiao Yi, et al.
Journal of Chemical Theory and Computation (2024) Vol. 20, Iss. 11, pp. 4533-4544
Open Access | Times Cited: 5
Accurate and efficient molecular dynamics based on machine learning and non von Neumann architecture
Pinghui Mo, Chang Li, Dan Zhao, et al.
npj Computational Materials (2022) Vol. 8, Iss. 1
Open Access | Times Cited: 22
Pinghui Mo, Chang Li, Dan Zhao, et al.
npj Computational Materials (2022) Vol. 8, Iss. 1
Open Access | Times Cited: 22
Hybrid interatomic potential for Sn
Mashroor S. Nitol, Khanh Dang, Saryu Fensin, et al.
Physical Review Materials (2023) Vol. 7, Iss. 4
Open Access | Times Cited: 10
Mashroor S. Nitol, Khanh Dang, Saryu Fensin, et al.
Physical Review Materials (2023) Vol. 7, Iss. 4
Open Access | Times Cited: 10
Foundations of molecular dynamics simulations: how and what
Giovanni Ciccotti, Sergio Decherchi, Simone Meloni
Deleted Journal (2025)
Open Access
Giovanni Ciccotti, Sergio Decherchi, Simone Meloni
Deleted Journal (2025)
Open Access
Unsupervised Machine Learning-Based Image Recognition of Raw Infrared Spectra: Toward Chemist-like Chemical Structural Classification and Beyond Numerical Data
Kentaro Fuku, Takefumi Yoshida
Journal of Chemical Information and Modeling (2025)
Closed Access
Kentaro Fuku, Takefumi Yoshida
Journal of Chemical Information and Modeling (2025)
Closed Access
Introduction of basic theory and principle of Raman scattering and spectroscopy
P. Patel, Mihir Shah, Neha R. Raghani, et al.
Elsevier eBooks (2025), pp. 1-11
Closed Access
P. Patel, Mihir Shah, Neha R. Raghani, et al.
Elsevier eBooks (2025), pp. 1-11
Closed Access
ОПИСАНИЯ МЕЖМОЛЕКУЛЯРНЫХ ВЗАИМОДЕЙСТВИЙ В ДИМЕРАХ (CH4)2, CH4NE, NE2
Bogdan Rutskoy
Вестник НИЯУ МИФИ (2025) Vol. 14, Iss. 2, pp. 103-115
Closed Access
Bogdan Rutskoy
Вестник НИЯУ МИФИ (2025) Vol. 14, Iss. 2, pp. 103-115
Closed Access
Unlocking the Potential of Machine Learning in Enhancing Quantum Chemical Calculations for Infrared Spectral Prediction
Adithya Ranjith Kartha, Dhanush P. Ajayakumar, Muhammad Zaffwan Idris, et al.
ACS Omega (2025)
Open Access
Adithya Ranjith Kartha, Dhanush P. Ajayakumar, Muhammad Zaffwan Idris, et al.
ACS Omega (2025)
Open Access
Strategies for the construction of machine-learning potentials for accurate and efficient atomic-scale simulations
April M Miksch, Tobias Morawietz, Johannes Kästner, et al.
Machine Learning Science and Technology (2021) Vol. 2, Iss. 3, pp. 031001-031001
Open Access | Times Cited: 19
April M Miksch, Tobias Morawietz, Johannes Kästner, et al.
Machine Learning Science and Technology (2021) Vol. 2, Iss. 3, pp. 031001-031001
Open Access | Times Cited: 19
AENET–LAMMPS and AENET–TINKER: Interfaces for accurate and efficient molecular dynamics simulations with machine learning potentials
Michael S. Chen, Tobias Morawietz, Hideki Mori, et al.
The Journal of Chemical Physics (2021) Vol. 155, Iss. 7
Open Access | Times Cited: 19
Michael S. Chen, Tobias Morawietz, Hideki Mori, et al.
The Journal of Chemical Physics (2021) Vol. 155, Iss. 7
Open Access | Times Cited: 19
The Role of Bond Functions in Describing Intermolecular Electron Correlation for Van der Waals Dimers: A Study of (CH4)2 and Ne2
Bogdan Rutskoy, Г. К. Озеров, Dmitry S. Bezrukov
International Journal of Molecular Sciences (2024) Vol. 25, Iss. 3, pp. 1472-1472
Open Access | Times Cited: 2
Bogdan Rutskoy, Г. К. Озеров, Dmitry S. Bezrukov
International Journal of Molecular Sciences (2024) Vol. 25, Iss. 3, pp. 1472-1472
Open Access | Times Cited: 2