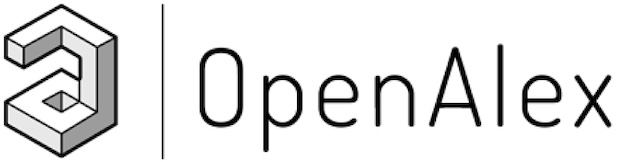
OpenAlex is a bibliographic catalogue of scientific papers, authors and institutions accessible in open access mode, named after the Library of Alexandria. It's citation coverage is excellent and I hope you will find utility in this listing of citing articles!
If you click the article title, you'll navigate to the article, as listed in CrossRef. If you click the Open Access links, you'll navigate to the "best Open Access location". Clicking the citation count will open this listing for that article. Lastly at the bottom of the page, you'll find basic pagination options.
Requested Article:
Grading of Clear Cell Renal Cell Carcinomas by Using Machine Learning Based on Artificial Neural Networks and Radiomic Signatures Extracted From Multidetector Computed Tomography Images
Xiaopeng He, Yi Wei, Hanmei Zhang, et al.
Academic Radiology (2019) Vol. 27, Iss. 2, pp. 157-168
Closed Access | Times Cited: 31
Xiaopeng He, Yi Wei, Hanmei Zhang, et al.
Academic Radiology (2019) Vol. 27, Iss. 2, pp. 157-168
Closed Access | Times Cited: 31
Showing 1-25 of 31 citing articles:
Radiogenomics in Renal Cancer Management—Current Evidence and Future Prospects
Matteo Ferro, Gennaro Musi, Michele Marchioni, et al.
International Journal of Molecular Sciences (2023) Vol. 24, Iss. 5, pp. 4615-4615
Open Access | Times Cited: 56
Matteo Ferro, Gennaro Musi, Michele Marchioni, et al.
International Journal of Molecular Sciences (2023) Vol. 24, Iss. 5, pp. 4615-4615
Open Access | Times Cited: 56
Radiomics Applications in Renal Tumor Assessment: A Comprehensive Review of the Literature
Rodrigo Suarez-Ibarrola, Mario Basulto‐Martínez, Alexander Heinze, et al.
Cancers (2020) Vol. 12, Iss. 6, pp. 1387-1387
Open Access | Times Cited: 46
Rodrigo Suarez-Ibarrola, Mario Basulto‐Martínez, Alexander Heinze, et al.
Cancers (2020) Vol. 12, Iss. 6, pp. 1387-1387
Open Access | Times Cited: 46
Prediction of the Ki-67 marker index in hepatocellular carcinoma based on CT radiomics features
Hongzhen Wu, Xiaorui Han, Zihua Wang, et al.
Physics in Medicine and Biology (2020) Vol. 65, Iss. 23, pp. 235048-235048
Open Access | Times Cited: 37
Hongzhen Wu, Xiaorui Han, Zihua Wang, et al.
Physics in Medicine and Biology (2020) Vol. 65, Iss. 23, pp. 235048-235048
Open Access | Times Cited: 37
Role of DECT-Based Imaging Biomarkers and Machine Learning to Predict Renal Cell Carcinoma Subtypes
Neha Baijal, Amit Gupta, Sanil Garg, et al.
Indian journal of radiology and imaging - new series/Indian journal of radiology and imaging/Indian Journal of Radiology & Imaging (2025)
Open Access
Neha Baijal, Amit Gupta, Sanil Garg, et al.
Indian journal of radiology and imaging - new series/Indian journal of radiology and imaging/Indian Journal of Radiology & Imaging (2025)
Open Access
Learnable image histograms-based deep radiomics for renal cell carcinoma grading and staging
Mohammad Arafat Hussain, Ghassan Hamarneh, Rafeef Garbi
Computerized Medical Imaging and Graphics (2021) Vol. 90, pp. 101924-101924
Closed Access | Times Cited: 24
Mohammad Arafat Hussain, Ghassan Hamarneh, Rafeef Garbi
Computerized Medical Imaging and Graphics (2021) Vol. 90, pp. 101924-101924
Closed Access | Times Cited: 24
Role of AI and Radiomic Markers in Early Diagnosis of Renal Cancer and Clinical Outcome Prediction: A Brief Review
Mohamed Shehata, Rasha T. Abouelkheir, Mallorie Gayhart, et al.
Cancers (2023) Vol. 15, Iss. 10, pp. 2835-2835
Open Access | Times Cited: 9
Mohamed Shehata, Rasha T. Abouelkheir, Mallorie Gayhart, et al.
Cancers (2023) Vol. 15, Iss. 10, pp. 2835-2835
Open Access | Times Cited: 9
Radiomics Machine Learning Analysis of Clear Cell Renal Cell Carcinoma for Tumour Grade Prediction Based on Intra-Tumoural Sub-Region Heterogeneity
Abeer J. Alhussaini, J. Douglas Steele, Adel Jawli, et al.
Cancers (2024) Vol. 16, Iss. 8, pp. 1454-1454
Open Access | Times Cited: 3
Abeer J. Alhussaini, J. Douglas Steele, Adel Jawli, et al.
Cancers (2024) Vol. 16, Iss. 8, pp. 1454-1454
Open Access | Times Cited: 3
Artificial Intelligence in Renal Mass Characterization: A Systematic Review of Methodologic Items Related to Modeling, Performance Evaluation, Clinical Utility, and Transparency
Burak Koçak, Ozlem Korkmaz Kaya, Çağrı Erdim, et al.
American Journal of Roentgenology (2020) Vol. 215, Iss. 5, pp. 1113-1122
Closed Access | Times Cited: 27
Burak Koçak, Ozlem Korkmaz Kaya, Çağrı Erdim, et al.
American Journal of Roentgenology (2020) Vol. 215, Iss. 5, pp. 1113-1122
Closed Access | Times Cited: 27
Prediction models for clear cell renal cell carcinoma ISUP/WHO grade: comparison between CT radiomics and conventional contrast-enhanced CT
Dong Han, Yong Yu, Nan Yu, et al.
British Journal of Radiology (2020) Vol. 93, Iss. 1114
Open Access | Times Cited: 19
Dong Han, Yong Yu, Nan Yu, et al.
British Journal of Radiology (2020) Vol. 93, Iss. 1114
Open Access | Times Cited: 19
Fuhrman nuclear grade prediction of clear cell renal cell carcinoma: influence of volume of interest delineation strategies on machine learning-based dynamic enhanced CT radiomics analysis
Shi‐Wei Luo, Ruili Wei, Songlin Lu, et al.
European Radiology (2021) Vol. 32, Iss. 4, pp. 2340-2350
Closed Access | Times Cited: 17
Shi‐Wei Luo, Ruili Wei, Songlin Lu, et al.
European Radiology (2021) Vol. 32, Iss. 4, pp. 2340-2350
Closed Access | Times Cited: 17
Radiomics Machine Learning Analysis of Clear Cell Renal Cell Carcinoma for Tumour Grade Prediction based on Intra-Tumoural Subregion Heterogeneity
Abeer J. Alhussaini, J. Douglas Steele, Adel Jawli, et al.
(2024)
Open Access | Times Cited: 2
Abeer J. Alhussaini, J. Douglas Steele, Adel Jawli, et al.
(2024)
Open Access | Times Cited: 2
Machine Learning in Radiomic Renal Mass Characterization: Fundamentals, Applications, Challenges, and Future Directions
Burak Koçak, Ece Ateş, Aytül Hande Yardımcı, et al.
American Journal of Roentgenology (2020) Vol. 215, Iss. 4, pp. 920-928
Closed Access | Times Cited: 18
Burak Koçak, Ece Ateş, Aytül Hande Yardımcı, et al.
American Journal of Roentgenology (2020) Vol. 215, Iss. 4, pp. 920-928
Closed Access | Times Cited: 18
Effect of radiomics from different virtual monochromatic images in dual-energy spectral CT on the WHO/ISUP classification of clear cell renal cell carcinoma
Dong Han, Yongqiang Yu, Taiping He, et al.
Clinical Radiology (2021) Vol. 76, Iss. 8, pp. 627.e23-627.e29
Closed Access | Times Cited: 15
Dong Han, Yongqiang Yu, Taiping He, et al.
Clinical Radiology (2021) Vol. 76, Iss. 8, pp. 627.e23-627.e29
Closed Access | Times Cited: 15
Evaluation of radiomics and machine learning in identification of aggressive tumor features in renal cell carcinoma (RCC)
Sidharth Gurbani, Dane Morgan, Varun Jog, et al.
Abdominal Radiology (2021) Vol. 46, Iss. 9, pp. 4278-4288
Open Access | Times Cited: 14
Sidharth Gurbani, Dane Morgan, Varun Jog, et al.
Abdominal Radiology (2021) Vol. 46, Iss. 9, pp. 4278-4288
Open Access | Times Cited: 14
Radiomics to better characterize small renal masses
Teele Kuusk, Joana B. Neves, Maxine Tran, et al.
World Journal of Urology (2021) Vol. 39, Iss. 8, pp. 2861-2868
Open Access | Times Cited: 14
Teele Kuusk, Joana B. Neves, Maxine Tran, et al.
World Journal of Urology (2021) Vol. 39, Iss. 8, pp. 2861-2868
Open Access | Times Cited: 14
Recognition of bovine milk somatic cells based on multi-feature extraction and a GBDT-AdaBoost fusion model
Jie Bai, Heru Xue, Xinhua Jiang, et al.
Mathematical Biosciences & Engineering (2022) Vol. 19, Iss. 6, pp. 5850-5866
Open Access | Times Cited: 8
Jie Bai, Heru Xue, Xinhua Jiang, et al.
Mathematical Biosciences & Engineering (2022) Vol. 19, Iss. 6, pp. 5850-5866
Open Access | Times Cited: 8
Kidney cancer management 3.0: can artificial intelligence make us better?
Matthew Lee, Shuanzeng Wei, Jordan Anaokar, et al.
Current Opinion in Urology (2021) Vol. 31, Iss. 4, pp. 409-415
Closed Access | Times Cited: 11
Matthew Lee, Shuanzeng Wei, Jordan Anaokar, et al.
Current Opinion in Urology (2021) Vol. 31, Iss. 4, pp. 409-415
Closed Access | Times Cited: 11
Deep learning techniques for imaging diagnosis of renal cell carcinoma: current and emerging trends
Zijie Wang, Xiaofei Zhang, Xinning Wang, et al.
Frontiers in Oncology (2023) Vol. 13
Open Access | Times Cited: 4
Zijie Wang, Xiaofei Zhang, Xinning Wang, et al.
Frontiers in Oncology (2023) Vol. 13
Open Access | Times Cited: 4
Characterisation of Intra-tumoural Subregions Heterogeneity and Tumour Grade Prediction using CT Scan Images: An In-depth Radiomics Features Machine Learning Analysis of Clear Cell Renal Cell Carcinoma
Abeer J. Alhussaini, J. Douglas Steele, Adel Jawli, et al.
(2024)
Open Access | Times Cited: 1
Abeer J. Alhussaini, J. Douglas Steele, Adel Jawli, et al.
(2024)
Open Access | Times Cited: 1
Differentiate Xp11.2 Translocation Renal Cell Carcinoma from Computed Tomography Images and Clinical Data with ResNet-18 CNN and XGBoost
Yanwen Lu, Wenliang Ma, Xiang Da Dong, et al.
Computer Modeling in Engineering & Sciences (2023) Vol. 136, Iss. 1, pp. 347-362
Open Access | Times Cited: 3
Yanwen Lu, Wenliang Ma, Xiang Da Dong, et al.
Computer Modeling in Engineering & Sciences (2023) Vol. 136, Iss. 1, pp. 347-362
Open Access | Times Cited: 3
Radiomics Machine Learning Analysis of Clear Cell Renal Cell Carcinoma for Tumour Grade Prediction Based on Intra-tumoural Subregion Heterogeneity
Abeer J. Alhussaini, J. Douglas Steele, Adel Jawli, et al.
(2024)
Open Access
Abeer J. Alhussaini, J. Douglas Steele, Adel Jawli, et al.
(2024)
Open Access
Radiomics Machine Learning Analysis of Clear Cell Renal Cell Carcinoma for Tumour Grade Prediction Based on Intra-tumoural Subregion Heterogeneity
Abeer J. Alhussaini, J. Douglas Steele, Adel Jawli, et al.
(2024)
Open Access
Abeer J. Alhussaini, J. Douglas Steele, Adel Jawli, et al.
(2024)
Open Access
Research Progress on Renal Cell Carcinoma and Radiomics
梦家 王
Advances in Clinical Medicine (2024) Vol. 14, Iss. 04, pp. 1706-1712
Closed Access
梦家 王
Advances in Clinical Medicine (2024) Vol. 14, Iss. 04, pp. 1706-1712
Closed Access
Survival analysis of clear cell renal cell carcinoma based on radiomics and deep learning features from CT images
Zhennan Lu, Sijia Wu, Dan Ni, et al.
Medicine (2024) Vol. 103, Iss. 51, pp. e40723-e40723
Open Access
Zhennan Lu, Sijia Wu, Dan Ni, et al.
Medicine (2024) Vol. 103, Iss. 51, pp. e40723-e40723
Open Access
On High Grade Kidney Cancer and Machine Learning
Nicole E. Curci
Academic Radiology (2019) Vol. 27, Iss. 2, pp. 169-170
Open Access | Times Cited: 3
Nicole E. Curci
Academic Radiology (2019) Vol. 27, Iss. 2, pp. 169-170
Open Access | Times Cited: 3