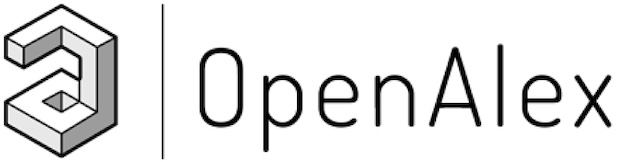
OpenAlex is a bibliographic catalogue of scientific papers, authors and institutions accessible in open access mode, named after the Library of Alexandria. It's citation coverage is excellent and I hope you will find utility in this listing of citing articles!
If you click the article title, you'll navigate to the article, as listed in CrossRef. If you click the Open Access links, you'll navigate to the "best Open Access location". Clicking the citation count will open this listing for that article. Lastly at the bottom of the page, you'll find basic pagination options.
Requested Article:
Occurrence prediction of cotton pests and diseases by bidirectional long short-term memory networks with climate and atmosphere circulation
Peng Chen, Qingxin Xiao, Jun Zhang, et al.
Computers and Electronics in Agriculture (2020) Vol. 176, pp. 105612-105612
Closed Access | Times Cited: 55
Peng Chen, Qingxin Xiao, Jun Zhang, et al.
Computers and Electronics in Agriculture (2020) Vol. 176, pp. 105612-105612
Closed Access | Times Cited: 55
Showing 1-25 of 55 citing articles:
Computer Vision, IoT and Data Fusion for Crop Disease Detection Using Machine Learning: A Survey and Ongoing Research
Maryam Ouhami, Adel Hafiane, Youssef Es-Saady, et al.
Remote Sensing (2021) Vol. 13, Iss. 13, pp. 2486-2486
Open Access | Times Cited: 173
Maryam Ouhami, Adel Hafiane, Youssef Es-Saady, et al.
Remote Sensing (2021) Vol. 13, Iss. 13, pp. 2486-2486
Open Access | Times Cited: 173
Rice-Fusion: A Multimodality Data Fusion Framework for Rice Disease Diagnosis
Rutuja Rajendra Patil, Sumit Kumar
IEEE Access (2022) Vol. 10, pp. 5207-5222
Open Access | Times Cited: 120
Rutuja Rajendra Patil, Sumit Kumar
IEEE Access (2022) Vol. 10, pp. 5207-5222
Open Access | Times Cited: 120
Survey on crop pest detection using deep learning and machine learning approaches
M. Chithambarathanu, M. K. Jeyakumar
Multimedia Tools and Applications (2023) Vol. 82, Iss. 27, pp. 42277-42310
Open Access | Times Cited: 58
M. Chithambarathanu, M. K. Jeyakumar
Multimedia Tools and Applications (2023) Vol. 82, Iss. 27, pp. 42277-42310
Open Access | Times Cited: 58
Smart applications and digital technologies in viticulture: A review
Javier Tardáguila, Manfred Stoll, Salvador Gutiérrez, et al.
Smart Agricultural Technology (2021) Vol. 1, pp. 100005-100005
Open Access | Times Cited: 86
Javier Tardáguila, Manfred Stoll, Salvador Gutiérrez, et al.
Smart Agricultural Technology (2021) Vol. 1, pp. 100005-100005
Open Access | Times Cited: 86
Accurate cotton diseases and pests detection in complex background based on an improved YOLOX model
Yuanjia Zhang, Benxue Ma, Yating Hu, et al.
Computers and Electronics in Agriculture (2022) Vol. 203, pp. 107484-107484
Closed Access | Times Cited: 47
Yuanjia Zhang, Benxue Ma, Yating Hu, et al.
Computers and Electronics in Agriculture (2022) Vol. 203, pp. 107484-107484
Closed Access | Times Cited: 47
Explainable deep convolutional neural networks for insect pest recognition
Solemane Coulibaly, Bernard Kamsu-Foguem, Dantouma Kamissoko, et al.
Journal of Cleaner Production (2022) Vol. 371, pp. 133638-133638
Open Access | Times Cited: 46
Solemane Coulibaly, Bernard Kamsu-Foguem, Dantouma Kamissoko, et al.
Journal of Cleaner Production (2022) Vol. 371, pp. 133638-133638
Open Access | Times Cited: 46
Artificial-intelligence and sensing techniques for the management of insect pests and diseases in cotton: a systematic literature review
Raul Toscano-Miranda, Magaly Toro, José Aguilar, et al.
The Journal of Agricultural Science (2022) Vol. 160, Iss. 1-2, pp. 16-31
Open Access | Times Cited: 43
Raul Toscano-Miranda, Magaly Toro, José Aguilar, et al.
The Journal of Agricultural Science (2022) Vol. 160, Iss. 1-2, pp. 16-31
Open Access | Times Cited: 43
A deep learning model for cotton disease prediction using fine-tuning with smart web application in agriculture
Md. Manowarul Islam, Md. Alamin Talukder, Md. Ruhul Amin Sarker, et al.
Intelligent Systems with Applications (2023) Vol. 20, pp. 200278-200278
Open Access | Times Cited: 29
Md. Manowarul Islam, Md. Alamin Talukder, Md. Ruhul Amin Sarker, et al.
Intelligent Systems with Applications (2023) Vol. 20, pp. 200278-200278
Open Access | Times Cited: 29
Detecting diseases in apple tree leaves using FPN–ISResNet–Faster RCNN
Jingwei Hou, Chen Yang, Yonghong He, et al.
European Journal of Remote Sensing (2023) Vol. 56, Iss. 1
Open Access | Times Cited: 24
Jingwei Hou, Chen Yang, Yonghong He, et al.
European Journal of Remote Sensing (2023) Vol. 56, Iss. 1
Open Access | Times Cited: 24
Pest and Disease Prediction and Management for Sugarcane Using a Hybrid Autoregressive Integrated Moving Average—A Long Short-Term Memory Model
Ming-hui Wang, Tong Li
Agriculture (2025) Vol. 15, Iss. 5, pp. 500-500
Open Access | Times Cited: 1
Ming-hui Wang, Tong Li
Agriculture (2025) Vol. 15, Iss. 5, pp. 500-500
Open Access | Times Cited: 1
Insect resistance management in Bacillus thuringiensis cotton by MGPS (multiple genes pyramiding and silencing)
Muhammad Mubashar Zafar, Abdul Razzaq, Muhammad Awais Farooq, et al.
Journal of Cotton Research (2020) Vol. 3, Iss. 1
Open Access | Times Cited: 68
Muhammad Mubashar Zafar, Abdul Razzaq, Muhammad Awais Farooq, et al.
Journal of Cotton Research (2020) Vol. 3, Iss. 1
Open Access | Times Cited: 68
Using Time-Series Generative Adversarial Networks to Synthesize Sensing Data for Pest Incidence Forecasting on Sustainable Agriculture
Chen-Yu Tai, Wun-Jhe Wang, Yueh‐Min Huang
Sustainability (2023) Vol. 15, Iss. 10, pp. 7834-7834
Open Access | Times Cited: 22
Chen-Yu Tai, Wun-Jhe Wang, Yueh‐Min Huang
Sustainability (2023) Vol. 15, Iss. 10, pp. 7834-7834
Open Access | Times Cited: 22
Deep learning models based on hyperspectral data and time-series phenotypes for predicting quality attributes in lettuces under water stress
S.K. Yu, Jiangchuan Fan, Xianju Lu, et al.
Computers and Electronics in Agriculture (2023) Vol. 211, pp. 108034-108034
Closed Access | Times Cited: 19
S.K. Yu, Jiangchuan Fan, Xianju Lu, et al.
Computers and Electronics in Agriculture (2023) Vol. 211, pp. 108034-108034
Closed Access | Times Cited: 19
Managing Practical Resistance of Lepidopteran Pests to Bt Cotton in China
Yudong Quan, Kongming Wu
Insects (2023) Vol. 14, Iss. 2, pp. 179-179
Open Access | Times Cited: 17
Yudong Quan, Kongming Wu
Insects (2023) Vol. 14, Iss. 2, pp. 179-179
Open Access | Times Cited: 17
Cross-comparative review of Machine learning for plant disease detection: apple, cassava, cotton and potato plants
James Daniel Omaye, Emeka Ogbuju, Grace Ataguba, et al.
Artificial Intelligence in Agriculture (2024) Vol. 12, pp. 127-151
Open Access | Times Cited: 7
James Daniel Omaye, Emeka Ogbuju, Grace Ataguba, et al.
Artificial Intelligence in Agriculture (2024) Vol. 12, pp. 127-151
Open Access | Times Cited: 7
Testing the Performance of LSTM and ARIMA Models for In-Season Forecasting of Canopy Cover (CC) in Cotton Crops
Sambandh Bhusan Dhal, Stavros Kalafatis, Ulisses Braga-Neto, et al.
Remote Sensing (2024) Vol. 16, Iss. 11, pp. 1906-1906
Open Access | Times Cited: 7
Sambandh Bhusan Dhal, Stavros Kalafatis, Ulisses Braga-Neto, et al.
Remote Sensing (2024) Vol. 16, Iss. 11, pp. 1906-1906
Open Access | Times Cited: 7
Transformer neural network to predict and interpret pregnancy loss from activity data in Holstein dairy cows
Dan Lin, Ákos Kenéz, J.A.A. McArt, et al.
Computers and Electronics in Agriculture (2023) Vol. 205, pp. 107638-107638
Closed Access | Times Cited: 11
Dan Lin, Ákos Kenéz, J.A.A. McArt, et al.
Computers and Electronics in Agriculture (2023) Vol. 205, pp. 107638-107638
Closed Access | Times Cited: 11
Role of Artificial Intelligence in Agriculture: An Analysis and Advancements With Focus on Plant Diseases
Ruchi Rani, Jayakrushna Sahoo, Sivaiah Bellamkonda, et al.
IEEE Access (2023) Vol. 11, pp. 137999-138019
Open Access | Times Cited: 11
Ruchi Rani, Jayakrushna Sahoo, Sivaiah Bellamkonda, et al.
IEEE Access (2023) Vol. 11, pp. 137999-138019
Open Access | Times Cited: 11
Leveraging machine learning for advancing insect pest control: A bibliometric analysis
Jiale Wang, Yan Chen, Jianxiang Huang, et al.
Journal of Applied Entomology (2024)
Closed Access | Times Cited: 4
Jiale Wang, Yan Chen, Jianxiang Huang, et al.
Journal of Applied Entomology (2024)
Closed Access | Times Cited: 4
Advances in Deep Learning Applications for Plant Disease and Pest Detection: A Review
Shaohua Wang, Dachuan Xu, Haojian Liang, et al.
Remote Sensing (2025) Vol. 17, Iss. 4, pp. 698-698
Open Access
Shaohua Wang, Dachuan Xu, Haojian Liang, et al.
Remote Sensing (2025) Vol. 17, Iss. 4, pp. 698-698
Open Access
Fine extraction of multi-crop planting area based on deep learning with Sentinel- 2 time-series data
Jingmin Jiang, Jiahua Zhang, Xue Wang, et al.
Environmental Science and Pollution Research (2025)
Closed Access
Jingmin Jiang, Jiahua Zhang, Xue Wang, et al.
Environmental Science and Pollution Research (2025)
Closed Access
The application of integrated deep learning models with the Assistance of meteorological factors in forecasting major tobacco diseases
Chen Yuan, Changcheng Li, Can Wang, et al.
Computers and Electronics in Agriculture (2025) Vol. 236, pp. 110429-110429
Closed Access
Chen Yuan, Changcheng Li, Can Wang, et al.
Computers and Electronics in Agriculture (2025) Vol. 236, pp. 110429-110429
Closed Access
Assessing the severity of cotton Verticillium wilt disease from in situ canopy images and spectra using convolutional neural networks
Xiaoyan Kang, Changping Huang, Lifu Zhang, et al.
The Crop Journal (2022) Vol. 11, Iss. 3, pp. 933-940
Open Access | Times Cited: 18
Xiaoyan Kang, Changping Huang, Lifu Zhang, et al.
The Crop Journal (2022) Vol. 11, Iss. 3, pp. 933-940
Open Access | Times Cited: 18
Intelligent pest forecasting with meteorological data: An explainable deep learning approach
Jiale Wang, Dong Zhang
Expert Systems with Applications (2024) Vol. 252, pp. 124137-124137
Closed Access | Times Cited: 3
Jiale Wang, Dong Zhang
Expert Systems with Applications (2024) Vol. 252, pp. 124137-124137
Closed Access | Times Cited: 3
SRNet-YOLO: A model for detecting tiny and very tiny pests in cotton fields based on super-resolution reconstruction
Sen Yang, Gang Zhou, Yuwei Feng, et al.
Frontiers in Plant Science (2024) Vol. 15
Open Access | Times Cited: 3
Sen Yang, Gang Zhou, Yuwei Feng, et al.
Frontiers in Plant Science (2024) Vol. 15
Open Access | Times Cited: 3