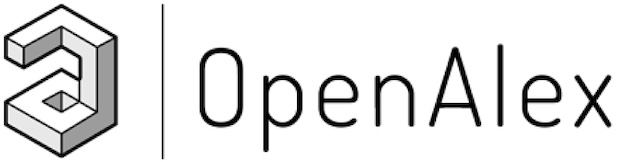
OpenAlex is a bibliographic catalogue of scientific papers, authors and institutions accessible in open access mode, named after the Library of Alexandria. It's citation coverage is excellent and I hope you will find utility in this listing of citing articles!
If you click the article title, you'll navigate to the article, as listed in CrossRef. If you click the Open Access links, you'll navigate to the "best Open Access location". Clicking the citation count will open this listing for that article. Lastly at the bottom of the page, you'll find basic pagination options.
Requested Article:
A comparison of machine learning methods for predicting the compressive strength of field-placed concrete
M.A. DeRousseau, Emil Laftchiev, Joseph Kasprzyk, et al.
Construction and Building Materials (2019) Vol. 228, pp. 116661-116661
Open Access | Times Cited: 155
M.A. DeRousseau, Emil Laftchiev, Joseph Kasprzyk, et al.
Construction and Building Materials (2019) Vol. 228, pp. 116661-116661
Open Access | Times Cited: 155
Showing 1-25 of 155 citing articles:
Machine learning for structural engineering: A state-of-the-art review
Huu‐Tai Thai
Structures (2022) Vol. 38, pp. 448-491
Closed Access | Times Cited: 414
Huu‐Tai Thai
Structures (2022) Vol. 38, pp. 448-491
Closed Access | Times Cited: 414
Machine learning-based prediction for compressive and flexural strengths of steel fiber-reinforced concrete
Min-Chang Kang, Doo‐Yeol Yoo, Rishi Gupta
Construction and Building Materials (2020) Vol. 266, pp. 121117-121117
Closed Access | Times Cited: 293
Min-Chang Kang, Doo‐Yeol Yoo, Rishi Gupta
Construction and Building Materials (2020) Vol. 266, pp. 121117-121117
Closed Access | Times Cited: 293
A novel approach to explain the black-box nature of machine learning in compressive strength predictions of concrete using Shapley additive explanations (SHAP)
I.U. Ekanayake, D.P.P. Meddage, Upaka Rathnayake
Case Studies in Construction Materials (2022) Vol. 16, pp. e01059-e01059
Open Access | Times Cited: 260
I.U. Ekanayake, D.P.P. Meddage, Upaka Rathnayake
Case Studies in Construction Materials (2022) Vol. 16, pp. e01059-e01059
Open Access | Times Cited: 260
Interpretable Ensemble-Machine-Learning models for predicting creep behavior of concrete
Minfei Liang, Ze Chang, Zhi Wan, et al.
Cement and Concrete Composites (2021) Vol. 125, pp. 104295-104295
Open Access | Times Cited: 205
Minfei Liang, Ze Chang, Zhi Wan, et al.
Cement and Concrete Composites (2021) Vol. 125, pp. 104295-104295
Open Access | Times Cited: 205
Machine learning in concrete science: applications, challenges, and best practices
Zhanzhao Li, Jinyoung Yoon, Rui Zhang, et al.
npj Computational Materials (2022) Vol. 8, Iss. 1
Open Access | Times Cited: 201
Zhanzhao Li, Jinyoung Yoon, Rui Zhang, et al.
npj Computational Materials (2022) Vol. 8, Iss. 1
Open Access | Times Cited: 201
Revealing the nature of metakaolin-based concrete materials using artificial intelligence techniques
Panagiotis G. Asteris, Paulo B. Lourénço, Panayiotis C. Roussis, et al.
Construction and Building Materials (2022) Vol. 322, pp. 126500-126500
Closed Access | Times Cited: 123
Panagiotis G. Asteris, Paulo B. Lourénço, Panayiotis C. Roussis, et al.
Construction and Building Materials (2022) Vol. 322, pp. 126500-126500
Closed Access | Times Cited: 123
Machine Learning for Risk and Resilience Assessment in Structural Engineering: Progress and Future Trends
Xiaowei Wang, Ram K. Mazumder, Babak Salarieh, et al.
Journal of Structural Engineering (2022) Vol. 148, Iss. 8
Closed Access | Times Cited: 110
Xiaowei Wang, Ram K. Mazumder, Babak Salarieh, et al.
Journal of Structural Engineering (2022) Vol. 148, Iss. 8
Closed Access | Times Cited: 110
To predict the compressive strength of self compacting concrete with recycled aggregates utilizing ensemble machine learning models
Jesús de‐Prado‐Gil, Covadonga Palencia, Neemias Silva-Monteiro, et al.
Case Studies in Construction Materials (2022) Vol. 16, pp. e01046-e01046
Open Access | Times Cited: 94
Jesús de‐Prado‐Gil, Covadonga Palencia, Neemias Silva-Monteiro, et al.
Case Studies in Construction Materials (2022) Vol. 16, pp. e01046-e01046
Open Access | Times Cited: 94
Artificial Intelligence in Physical Sciences: Symbolic Regression Trends and Perspectives
Dimitrios Angelis, Filippos Sofos, Theodoros E. Karakasidis
Archives of Computational Methods in Engineering (2023) Vol. 30, Iss. 6, pp. 3845-3865
Open Access | Times Cited: 82
Dimitrios Angelis, Filippos Sofos, Theodoros E. Karakasidis
Archives of Computational Methods in Engineering (2023) Vol. 30, Iss. 6, pp. 3845-3865
Open Access | Times Cited: 82
Prediction and optimization model of sustainable concrete properties using machine learning, deep learning and swarm intelligence: A review
Shiqi Wang, Peng Xia, Keyu Chen, et al.
Journal of Building Engineering (2023) Vol. 80, pp. 108065-108065
Closed Access | Times Cited: 65
Shiqi Wang, Peng Xia, Keyu Chen, et al.
Journal of Building Engineering (2023) Vol. 80, pp. 108065-108065
Closed Access | Times Cited: 65
Hybrid data-driven approaches to predicting the compressive strength of ultra-high-performance concrete using SHAP and PDP analyses
Abul Kashem, Rezaul Karim, Somir Chandra Malo, et al.
Case Studies in Construction Materials (2024) Vol. 20, pp. e02991-e02991
Open Access | Times Cited: 58
Abul Kashem, Rezaul Karim, Somir Chandra Malo, et al.
Case Studies in Construction Materials (2024) Vol. 20, pp. e02991-e02991
Open Access | Times Cited: 58
Predicting ultra-high-performance concrete compressive strength using gene expression programming method
Hisham Alabduljabbar, Majid Khan, Hamad Hassan Awan, et al.
Case Studies in Construction Materials (2023) Vol. 18, pp. e02074-e02074
Open Access | Times Cited: 53
Hisham Alabduljabbar, Majid Khan, Hamad Hassan Awan, et al.
Case Studies in Construction Materials (2023) Vol. 18, pp. e02074-e02074
Open Access | Times Cited: 53
Efficient machine learning method for evaluating compressive strength of cement stabilized soft soil
Chen Zhang, Zhiduo Zhu, Fa Liu, et al.
Construction and Building Materials (2023) Vol. 392, pp. 131887-131887
Closed Access | Times Cited: 47
Chen Zhang, Zhiduo Zhu, Fa Liu, et al.
Construction and Building Materials (2023) Vol. 392, pp. 131887-131887
Closed Access | Times Cited: 47
Estimating compressive strength of coral sand aggregate concrete in marine environment by combining physical experiments and machine learning-based techniques
Zhiming Chao, Zhikang Li, Youkou Dong, et al.
Ocean Engineering (2024) Vol. 308, pp. 118320-118320
Closed Access | Times Cited: 30
Zhiming Chao, Zhikang Li, Youkou Dong, et al.
Ocean Engineering (2024) Vol. 308, pp. 118320-118320
Closed Access | Times Cited: 30
Transfer learning framework for modelling the compressive strength of ultra-high performance geopolymer concrete
Ho Anh Thu Nguyen, Duy Hoang Pham, Anh Tuấn Lê, et al.
Construction and Building Materials (2025) Vol. 459, pp. 139746-139746
Closed Access | Times Cited: 3
Ho Anh Thu Nguyen, Duy Hoang Pham, Anh Tuấn Lê, et al.
Construction and Building Materials (2025) Vol. 459, pp. 139746-139746
Closed Access | Times Cited: 3
A new method to evaluate features importance in machine-learning based prediction of concrete compressive strength
Yuanhao Dong, Jinhui Tang, Xiaohang Xu, et al.
Journal of Building Engineering (2025), pp. 111874-111874
Closed Access | Times Cited: 2
Yuanhao Dong, Jinhui Tang, Xiaohang Xu, et al.
Journal of Building Engineering (2025), pp. 111874-111874
Closed Access | Times Cited: 2
Analyzing the Compressive Strength of Ceramic Waste-Based Concrete Using Experiment and Artificial Neural Network (ANN) Approach
Hongwei Song, Ayaz Ahmad, Krzysztof Adam Ostrowski, et al.
Materials (2021) Vol. 14, Iss. 16, pp. 4518-4518
Open Access | Times Cited: 78
Hongwei Song, Ayaz Ahmad, Krzysztof Adam Ostrowski, et al.
Materials (2021) Vol. 14, Iss. 16, pp. 4518-4518
Open Access | Times Cited: 78
Novel machine learning applications on fly ash based concrete: An overview
Geetanjli Khambra, Prashant Kumar Shukla
Materials Today Proceedings (2021) Vol. 80, pp. 3411-3417
Closed Access | Times Cited: 77
Geetanjli Khambra, Prashant Kumar Shukla
Materials Today Proceedings (2021) Vol. 80, pp. 3411-3417
Closed Access | Times Cited: 77
Data-driven model for ternary-blend concrete compressive strength prediction using machine learning approach
Babatunde Abiodun Salami, Teslim Olayiwola, Tajudeen A. Oyehan, et al.
Construction and Building Materials (2021) Vol. 301, pp. 124152-124152
Closed Access | Times Cited: 70
Babatunde Abiodun Salami, Teslim Olayiwola, Tajudeen A. Oyehan, et al.
Construction and Building Materials (2021) Vol. 301, pp. 124152-124152
Closed Access | Times Cited: 70
Prediction of seven-day compressive strength of field concrete
Xueqing Zhang, Muhammad Zeshan Akber, Wei Zheng
Construction and Building Materials (2021) Vol. 305, pp. 124604-124604
Closed Access | Times Cited: 68
Xueqing Zhang, Muhammad Zeshan Akber, Wei Zheng
Construction and Building Materials (2021) Vol. 305, pp. 124604-124604
Closed Access | Times Cited: 68
Structural material demand and associated embodied carbon emissions of the United States building stock: 2020–2100
Jay H. Arehart, Francesco Pomponi, Bernardino D’Amico, et al.
Resources Conservation and Recycling (2022) Vol. 186, pp. 106583-106583
Open Access | Times Cited: 60
Jay H. Arehart, Francesco Pomponi, Bernardino D’Amico, et al.
Resources Conservation and Recycling (2022) Vol. 186, pp. 106583-106583
Open Access | Times Cited: 60
Application of Multivariate Adaptive Regression Splines (MARS) approach in prediction of compressive strength of eco-friendly concrete
Ali H. Naser, Ali H. Badr, Sadiq N. Henedy, et al.
Case Studies in Construction Materials (2022) Vol. 17, pp. e01262-e01262
Open Access | Times Cited: 56
Ali H. Naser, Ali H. Badr, Sadiq N. Henedy, et al.
Case Studies in Construction Materials (2022) Vol. 17, pp. e01262-e01262
Open Access | Times Cited: 56
Evaluating the mechanical strength prediction performances of fly ash-based MPC mortar with artificial intelligence approaches
M. Aminul Haque, Bing Chen, Muhammad Faisal Javed, et al.
Journal of Cleaner Production (2022) Vol. 355, pp. 131815-131815
Closed Access | Times Cited: 45
M. Aminul Haque, Bing Chen, Muhammad Faisal Javed, et al.
Journal of Cleaner Production (2022) Vol. 355, pp. 131815-131815
Closed Access | Times Cited: 45
Design of concrete incorporating microencapsulated phase change materials for clean energy: A ternary machine learning approach based on generative adversarial networks
Afshin Marani, Lei Zhang, Moncef L. Nehdi
Engineering Applications of Artificial Intelligence (2022) Vol. 118, pp. 105652-105652
Closed Access | Times Cited: 42
Afshin Marani, Lei Zhang, Moncef L. Nehdi
Engineering Applications of Artificial Intelligence (2022) Vol. 118, pp. 105652-105652
Closed Access | Times Cited: 42
Geopolymer concrete for clean and sustainable construction – A state-of-the-art review on the mix design approaches
Mohd Asif Ansari, M. Shariq, Fareed Mahdi
Structures (2023) Vol. 55, pp. 1045-1070
Closed Access | Times Cited: 40
Mohd Asif Ansari, M. Shariq, Fareed Mahdi
Structures (2023) Vol. 55, pp. 1045-1070
Closed Access | Times Cited: 40