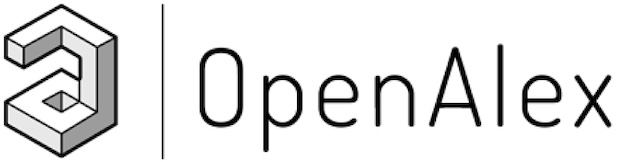
OpenAlex is a bibliographic catalogue of scientific papers, authors and institutions accessible in open access mode, named after the Library of Alexandria. It's citation coverage is excellent and I hope you will find utility in this listing of citing articles!
If you click the article title, you'll navigate to the article, as listed in CrossRef. If you click the Open Access links, you'll navigate to the "best Open Access location". Clicking the citation count will open this listing for that article. Lastly at the bottom of the page, you'll find basic pagination options.
Requested Article:
Predicting ultra-high-performance concrete compressive strength using gene expression programming method
Hisham Alabduljabbar, Majid Khan, Hamad Hassan Awan, et al.
Case Studies in Construction Materials (2023) Vol. 18, pp. e02074-e02074
Open Access | Times Cited: 53
Hisham Alabduljabbar, Majid Khan, Hamad Hassan Awan, et al.
Case Studies in Construction Materials (2023) Vol. 18, pp. e02074-e02074
Open Access | Times Cited: 53
Showing 1-25 of 53 citing articles:
Prediction of concrete and FRC properties at high temperature using machine and deep learning: A review of recent advances and future perspectives
Nizar Faisal Alkayem, Lei Shen, Ali Mayya, et al.
Journal of Building Engineering (2023) Vol. 83, pp. 108369-108369
Closed Access | Times Cited: 104
Nizar Faisal Alkayem, Lei Shen, Ali Mayya, et al.
Journal of Building Engineering (2023) Vol. 83, pp. 108369-108369
Closed Access | Times Cited: 104
Evaluation of water quality indexes with novel machine learning and SHapley Additive ExPlanation (SHAP) approaches
Ali Aldrees, Majid Khan, Abubakr Taha Bakheit Taha, et al.
Journal of Water Process Engineering (2024) Vol. 58, pp. 104789-104789
Closed Access | Times Cited: 70
Ali Aldrees, Majid Khan, Abubakr Taha Bakheit Taha, et al.
Journal of Water Process Engineering (2024) Vol. 58, pp. 104789-104789
Closed Access | Times Cited: 70
Hybrid data-driven approaches to predicting the compressive strength of ultra-high-performance concrete using SHAP and PDP analyses
Abul Kashem, Rezaul Karim, Somir Chandra Malo, et al.
Case Studies in Construction Materials (2024) Vol. 20, pp. e02991-e02991
Open Access | Times Cited: 58
Abul Kashem, Rezaul Karim, Somir Chandra Malo, et al.
Case Studies in Construction Materials (2024) Vol. 20, pp. e02991-e02991
Open Access | Times Cited: 58
Machine learning-driven predictive models for compressive strength of steel fiber reinforced concrete subjected to high temperatures
Rayed Alyousef, Muhammad Faisal Rehman, Majid Khan, et al.
Case Studies in Construction Materials (2023) Vol. 19, pp. e02418-e02418
Open Access | Times Cited: 48
Rayed Alyousef, Muhammad Faisal Rehman, Majid Khan, et al.
Case Studies in Construction Materials (2023) Vol. 19, pp. e02418-e02418
Open Access | Times Cited: 48
Hybrid machine learning approach to prediction of the compressive and flexural strengths of UHPC and parametric analysis with shapley additive explanations
Pobithra Das, Abul Kashem
Case Studies in Construction Materials (2023) Vol. 20, pp. e02723-e02723
Open Access | Times Cited: 48
Pobithra Das, Abul Kashem
Case Studies in Construction Materials (2023) Vol. 20, pp. e02723-e02723
Open Access | Times Cited: 48
Estimating compressive strength of concrete containing rice husk ash using interpretable machine learning-based models
Mana Alyami, Roz‐Ud‐Din Nassar, Majid Khan, et al.
Case Studies in Construction Materials (2024) Vol. 20, pp. e02901-e02901
Open Access | Times Cited: 43
Mana Alyami, Roz‐Ud‐Din Nassar, Majid Khan, et al.
Case Studies in Construction Materials (2024) Vol. 20, pp. e02901-e02901
Open Access | Times Cited: 43
Forecasting the strength of graphene nanoparticles-reinforced cementitious composites using ensemble learning algorithms
Majid Khan, Roz‐Ud‐Din Nassar, Waqar Anwar, et al.
Results in Engineering (2024) Vol. 21, pp. 101837-101837
Open Access | Times Cited: 28
Majid Khan, Roz‐Ud‐Din Nassar, Waqar Anwar, et al.
Results in Engineering (2024) Vol. 21, pp. 101837-101837
Open Access | Times Cited: 28
Metaheuristic optimization algorithms-based prediction modeling for titanium dioxide-Assisted photocatalytic degradation of air contaminants
Muhammad Faisal Javed, Bilal Siddiq, Kennedy C. Onyelowe, et al.
Results in Engineering (2024) Vol. 23, pp. 102637-102637
Open Access | Times Cited: 21
Muhammad Faisal Javed, Bilal Siddiq, Kennedy C. Onyelowe, et al.
Results in Engineering (2024) Vol. 23, pp. 102637-102637
Open Access | Times Cited: 21
Computational prediction of workability and mechanical properties of bentonite plastic concrete using multi-expression programming
Majid Khan, Mujahid Ali, Taoufik Najeh, et al.
Scientific Reports (2024) Vol. 14, Iss. 1
Open Access | Times Cited: 20
Majid Khan, Mujahid Ali, Taoufik Najeh, et al.
Scientific Reports (2024) Vol. 14, Iss. 1
Open Access | Times Cited: 20
Leveraging machine learning to evaluate the effect of raw materials on the compressive strength of ultra-high-performance concrete
Mohamed Abdellatief, G. Murali, Saurav Dixit
Results in Engineering (2025) Vol. 25, pp. 104542-104542
Open Access | Times Cited: 7
Mohamed Abdellatief, G. Murali, Saurav Dixit
Results in Engineering (2025) Vol. 25, pp. 104542-104542
Open Access | Times Cited: 7
Compressive strength prediction models for concrete containing nano materials and exposed to elevated temperatures
Hany A. Dahish, Ahmed D. Almutairi
Results in Engineering (2025), pp. 103975-103975
Open Access | Times Cited: 5
Hany A. Dahish, Ahmed D. Almutairi
Results in Engineering (2025), pp. 103975-103975
Open Access | Times Cited: 5
Predicting Penetration Depth in Ultra-High-Performance Concrete Targets under Ballistic Impact: An Interpretable Machine Learning Approach Augmented by Deep Generative Adversarial Network
Majid Khan, Muhammad Faisal Javed, Nashwan Adnan Othman, et al.
Results in Engineering (2025), pp. 103909-103909
Open Access | Times Cited: 3
Majid Khan, Muhammad Faisal Javed, Nashwan Adnan Othman, et al.
Results in Engineering (2025), pp. 103909-103909
Open Access | Times Cited: 3
Towards sustainable construction: Machine learning based predictive models for strength and durability characteristics of blended cement concrete
Majid Khan, Muhammad Faisal Javed
Materials Today Communications (2023) Vol. 37, pp. 107428-107428
Closed Access | Times Cited: 42
Majid Khan, Muhammad Faisal Javed
Materials Today Communications (2023) Vol. 37, pp. 107428-107428
Closed Access | Times Cited: 42
Forecasting the strength characteristics of concrete incorporating waste foundry sand using advance machine algorithms including deep learning
Rayed Alyousef, Roz‐Ud‐Din Nassar, Majid Khan, et al.
Case Studies in Construction Materials (2023) Vol. 19, pp. e02459-e02459
Open Access | Times Cited: 35
Rayed Alyousef, Roz‐Ud‐Din Nassar, Majid Khan, et al.
Case Studies in Construction Materials (2023) Vol. 19, pp. e02459-e02459
Open Access | Times Cited: 35
Application of metaheuristic optimization algorithms in predicting the compressive strength of 3D-printed fiber-reinforced concrete
Mana Alyami, Majid Khan, Muhammad Faisal Javed, et al.
Developments in the Built Environment (2023) Vol. 17, pp. 100307-100307
Open Access | Times Cited: 33
Mana Alyami, Majid Khan, Muhammad Faisal Javed, et al.
Developments in the Built Environment (2023) Vol. 17, pp. 100307-100307
Open Access | Times Cited: 33
Feature engineering for predicting compressive strength of high-strength concrete with machine learning models
Pramod Kumar, Bheem Pratap
Asian Journal of Civil Engineering (2023) Vol. 25, Iss. 1, pp. 723-736
Closed Access | Times Cited: 31
Pramod Kumar, Bheem Pratap
Asian Journal of Civil Engineering (2023) Vol. 25, Iss. 1, pp. 723-736
Closed Access | Times Cited: 31
Improved forecasting of the compressive strength of ultra‐high‐performance concrete (UHPC ) via the CatBoost model optimized with different algorithms
Metin Katlav, Faruk Ergen
Structural Concrete (2024)
Open Access | Times Cited: 16
Metin Katlav, Faruk Ergen
Structural Concrete (2024)
Open Access | Times Cited: 16
Assessment of the split tensile strength of fiber reinforced recycled aggregate concrete using interpretable approaches with graphical user interface
Hisham Alabduljabbar, Furqan Farooq, Mana Alyami, et al.
Materials Today Communications (2024) Vol. 38, pp. 108009-108009
Closed Access | Times Cited: 15
Hisham Alabduljabbar, Furqan Farooq, Mana Alyami, et al.
Materials Today Communications (2024) Vol. 38, pp. 108009-108009
Closed Access | Times Cited: 15
AI-driven design for the compressive strength of ultra-high performance geopolymer concrete (UHPGC): From explainable ensemble models to the graphical user interface
Metin Katlav, Faruk Ergen, İzzeddin Dönmez
Materials Today Communications (2024) Vol. 40, pp. 109915-109915
Closed Access | Times Cited: 14
Metin Katlav, Faruk Ergen, İzzeddin Dönmez
Materials Today Communications (2024) Vol. 40, pp. 109915-109915
Closed Access | Times Cited: 14
Optimizing Gene Expression Programming to Predict Shear Capacity in Corrugated Web Steel Beams
Mazen Shrif, Zaid A. Al-Sadoon, Samer Barakat, et al.
Civil Engineering Journal (2024) Vol. 10, Iss. 5, pp. 1370-1385
Open Access | Times Cited: 13
Mazen Shrif, Zaid A. Al-Sadoon, Samer Barakat, et al.
Civil Engineering Journal (2024) Vol. 10, Iss. 5, pp. 1370-1385
Open Access | Times Cited: 13
Predicting the properties of concrete incorporating graphene nano platelets by experimental and machine learning approaches
Rayed Alyousef, Roz‐Ud‐Din Nassar, Muhammad Fawad, et al.
Case Studies in Construction Materials (2024) Vol. 20, pp. e03018-e03018
Open Access | Times Cited: 12
Rayed Alyousef, Roz‐Ud‐Din Nassar, Muhammad Fawad, et al.
Case Studies in Construction Materials (2024) Vol. 20, pp. e03018-e03018
Open Access | Times Cited: 12
Application of metaheuristic algorithms for compressive strength prediction of steel fiber reinforced concrete exposed to high temperatures
Muhammad Faisal Javed, Majid Khan, Moncef L. Nehdi, et al.
Materials Today Communications (2024) Vol. 39, pp. 108832-108832
Closed Access | Times Cited: 12
Muhammad Faisal Javed, Majid Khan, Moncef L. Nehdi, et al.
Materials Today Communications (2024) Vol. 39, pp. 108832-108832
Closed Access | Times Cited: 12
Data-driven Models for Predicting Compressive Strength of 3D-printed Fiber-Reinforced Concrete using Interpretable Machine Learning Algorithms
Muhammad Arif, Faizullah Jan, A. Rezzoug, et al.
Case Studies in Construction Materials (2024) Vol. 21, pp. e03935-e03935
Open Access | Times Cited: 11
Muhammad Arif, Faizullah Jan, A. Rezzoug, et al.
Case Studies in Construction Materials (2024) Vol. 21, pp. e03935-e03935
Open Access | Times Cited: 11
Artificial intelligence prediction of the mechanical properties of banana peel-ash and bagasse blended geopolymer concrete
George Uwadiegwu Alaneme, Kolawole Adisa Olonade, Ebenezer Esenogho, et al.
Scientific Reports (2024) Vol. 14, Iss. 1
Open Access | Times Cited: 9
George Uwadiegwu Alaneme, Kolawole Adisa Olonade, Ebenezer Esenogho, et al.
Scientific Reports (2024) Vol. 14, Iss. 1
Open Access | Times Cited: 9
Predictive modeling for durability characteristics of blended cement concrete utilizing machine learning algorithms
Bo Fu, Hua Lei, Irfan Ullah, et al.
Case Studies in Construction Materials (2025), pp. e04209-e04209
Open Access | Times Cited: 1
Bo Fu, Hua Lei, Irfan Ullah, et al.
Case Studies in Construction Materials (2025), pp. e04209-e04209
Open Access | Times Cited: 1