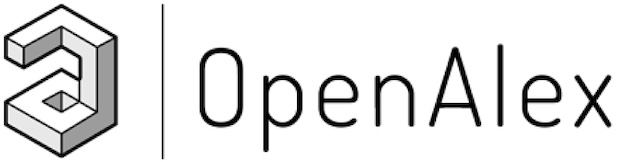
OpenAlex is a bibliographic catalogue of scientific papers, authors and institutions accessible in open access mode, named after the Library of Alexandria. It's citation coverage is excellent and I hope you will find utility in this listing of citing articles!
If you click the article title, you'll navigate to the article, as listed in CrossRef. If you click the Open Access links, you'll navigate to the "best Open Access location". Clicking the citation count will open this listing for that article. Lastly at the bottom of the page, you'll find basic pagination options.
Requested Article:
A physically consistent framework for fatigue life prediction using probabilistic physics-informed neural network
Taotao Zhou, Shan Jiang, Te Han, et al.
International Journal of Fatigue (2022) Vol. 166, pp. 107234-107234
Closed Access | Times Cited: 87
Taotao Zhou, Shan Jiang, Te Han, et al.
International Journal of Fatigue (2022) Vol. 166, pp. 107234-107234
Closed Access | Times Cited: 87
Showing 1-25 of 87 citing articles:
Physics-guided machine learning frameworks for fatigue life prediction of AM materials
Lanyi Wang, Shun‐Peng Zhu, Changqi Luo, et al.
International Journal of Fatigue (2023) Vol. 172, pp. 107658-107658
Closed Access | Times Cited: 70
Lanyi Wang, Shun‐Peng Zhu, Changqi Luo, et al.
International Journal of Fatigue (2023) Vol. 172, pp. 107658-107658
Closed Access | Times Cited: 70
A physics-informed machine learning approach for notch fatigue evaluation of alloys used in aerospace
Wenke Hao, Li Tan, Xiaoguang Yang, et al.
International Journal of Fatigue (2023) Vol. 170, pp. 107536-107536
Closed Access | Times Cited: 49
Wenke Hao, Li Tan, Xiaoguang Yang, et al.
International Journal of Fatigue (2023) Vol. 170, pp. 107536-107536
Closed Access | Times Cited: 49
Physics-Guided, Physics-Informed, and Physics-Encoded Neural Networks and Operators in Scientific Computing: Fluid and Solid Mechanics
Salah A. Faroughi, Nikhil M. Pawar, Célio Fernandes, et al.
Journal of Computing and Information Science in Engineering (2024) Vol. 24, Iss. 4
Closed Access | Times Cited: 49
Salah A. Faroughi, Nikhil M. Pawar, Célio Fernandes, et al.
Journal of Computing and Information Science in Engineering (2024) Vol. 24, Iss. 4
Closed Access | Times Cited: 49
Physics-informed Neural Networks (PINN) for computational solid mechanics: Numerical frameworks and applications
Haoteng Hu, Lehua Qi, Xujiang Chao
Thin-Walled Structures (2024) Vol. 205, pp. 112495-112495
Closed Access | Times Cited: 46
Haoteng Hu, Lehua Qi, Xujiang Chao
Thin-Walled Structures (2024) Vol. 205, pp. 112495-112495
Closed Access | Times Cited: 46
Defect sensitivity and fatigue design: Deterministic and probabilistic aspects in additively manufactured metallic materials
Xiaopeng Niu, Chao He, Shun‐Peng Zhu, et al.
Progress in Materials Science (2024) Vol. 144, pp. 101290-101290
Closed Access | Times Cited: 38
Xiaopeng Niu, Chao He, Shun‐Peng Zhu, et al.
Progress in Materials Science (2024) Vol. 144, pp. 101290-101290
Closed Access | Times Cited: 38
Physics-informed machine learning for low-cycle fatigue life prediction of 316 stainless steels
Lvfeng Jiang, Yanan Hu, Yuxuan Liu, et al.
International Journal of Fatigue (2024) Vol. 182, pp. 108187-108187
Closed Access | Times Cited: 28
Lvfeng Jiang, Yanan Hu, Yuxuan Liu, et al.
International Journal of Fatigue (2024) Vol. 182, pp. 108187-108187
Closed Access | Times Cited: 28
Physics-informed machine learning: A comprehensive review on applications in anomaly detection and condition monitoring
Yuandi Wu, Brett Sicard, S. Andrew Gadsden
Expert Systems with Applications (2024) Vol. 255, pp. 124678-124678
Open Access | Times Cited: 25
Yuandi Wu, Brett Sicard, S. Andrew Gadsden
Expert Systems with Applications (2024) Vol. 255, pp. 124678-124678
Open Access | Times Cited: 25
Evaluating fatigue onset in metallic materials: Problem, current focus and future perspectives
Enrico Salvati
International Journal of Fatigue (2024) Vol. 188, pp. 108487-108487
Open Access | Times Cited: 17
Enrico Salvati
International Journal of Fatigue (2024) Vol. 188, pp. 108487-108487
Open Access | Times Cited: 17
A survey on machine learning approaches for uncertainty quantification of engineering systems
Yan Shi, Pengfei Wei, Ke Feng, et al.
Machine learning for computational science and engineering (2025) Vol. 1, Iss. 1
Open Access | Times Cited: 2
Yan Shi, Pengfei Wei, Ke Feng, et al.
Machine learning for computational science and engineering (2025) Vol. 1, Iss. 1
Open Access | Times Cited: 2
Multi-fidelity physics-informed machine learning framework for fatigue life prediction of additive manufactured materials
Lanyi Wang, Shun‐Peng Zhu, Borui Wu, et al.
Computer Methods in Applied Mechanics and Engineering (2025) Vol. 439, pp. 117924-117924
Closed Access | Times Cited: 2
Lanyi Wang, Shun‐Peng Zhu, Borui Wu, et al.
Computer Methods in Applied Mechanics and Engineering (2025) Vol. 439, pp. 117924-117924
Closed Access | Times Cited: 2
Physics-informed machine learning and its structural integrity applications: state of the art
Shun‐Peng Zhu, Lanyi Wang, Changqi Luo, et al.
Philosophical Transactions of the Royal Society A Mathematical Physical and Engineering Sciences (2023) Vol. 381, Iss. 2260
Closed Access | Times Cited: 32
Shun‐Peng Zhu, Lanyi Wang, Changqi Luo, et al.
Philosophical Transactions of the Royal Society A Mathematical Physical and Engineering Sciences (2023) Vol. 381, Iss. 2260
Closed Access | Times Cited: 32
Multiaxial fatigue life prediction for various metallic materials based on the hybrid CNN‐LSTM neural network
Fei Heng, Jianxiong Gao, Rongxia Xu, et al.
Fatigue & Fracture of Engineering Materials & Structures (2023) Vol. 46, Iss. 5, pp. 1979-1996
Open Access | Times Cited: 27
Fei Heng, Jianxiong Gao, Rongxia Xu, et al.
Fatigue & Fracture of Engineering Materials & Structures (2023) Vol. 46, Iss. 5, pp. 1979-1996
Open Access | Times Cited: 27
Data-driven approach to very high cycle fatigue life prediction
Yu-Ke Liu, Jia-Le Fan, Gang Zhu, et al.
Engineering Fracture Mechanics (2023) Vol. 292, pp. 109630-109630
Closed Access | Times Cited: 25
Yu-Ke Liu, Jia-Le Fan, Gang Zhu, et al.
Engineering Fracture Mechanics (2023) Vol. 292, pp. 109630-109630
Closed Access | Times Cited: 25
Machine-Learning-Assisted Design of a Robust Biomimetic Radiative Cooling Metamaterial
Zhenmin Ding, Xin Li, Qingxiang Ji, et al.
ACS Materials Letters (2024) Vol. 6, Iss. 6, pp. 2416-2424
Open Access | Times Cited: 17
Zhenmin Ding, Xin Li, Qingxiang Ji, et al.
ACS Materials Letters (2024) Vol. 6, Iss. 6, pp. 2416-2424
Open Access | Times Cited: 17
Crack propagation simulation and overload fatigue life prediction via enhanced physics-informed neural networks
Zhiying Chen, Yanwei Dai, Yinghua Liu
International Journal of Fatigue (2024) Vol. 186, pp. 108382-108382
Closed Access | Times Cited: 11
Zhiying Chen, Yanwei Dai, Yinghua Liu
International Journal of Fatigue (2024) Vol. 186, pp. 108382-108382
Closed Access | Times Cited: 11
Critical physics informed fatigue life prediction of laser 3D printed AlSi10Mg alloys with mass internal defects
Yanan Hu, Yufeng She, Shengchuan Wu, et al.
International Journal of Mechanical Sciences (2024), pp. 109730-109730
Closed Access | Times Cited: 9
Yanan Hu, Yufeng She, Shengchuan Wu, et al.
International Journal of Mechanical Sciences (2024), pp. 109730-109730
Closed Access | Times Cited: 9
Physics-informed neural network with fuzzy partial differential equation for pavement performance prediction
Jiale Li, Song Zhang, Xuefei Wang
Automation in Construction (2025) Vol. 171, pp. 105983-105983
Closed Access | Times Cited: 1
Jiale Li, Song Zhang, Xuefei Wang
Automation in Construction (2025) Vol. 171, pp. 105983-105983
Closed Access | Times Cited: 1
A review on full-, zero-, and partial-knowledge based predictive models for industrial applications
Stefano Zampini, Guido Parodi, Luca Oneto, et al.
Information Fusion (2025), pp. 102996-102996
Open Access | Times Cited: 1
Stefano Zampini, Guido Parodi, Luca Oneto, et al.
Information Fusion (2025), pp. 102996-102996
Open Access | Times Cited: 1
Physics-informed Neural Network Supported Wiener Process for Degradation Modeling and Reliability Prediction
Zewen He, Shaoping Wang, Jian Shi, et al.
Reliability Engineering & System Safety (2025), pp. 110906-110906
Closed Access | Times Cited: 1
Zewen He, Shaoping Wang, Jian Shi, et al.
Reliability Engineering & System Safety (2025), pp. 110906-110906
Closed Access | Times Cited: 1
Probabilistic fatigue life prediction in additive manufacturing materials with a physics-informed neural network framework
Feng Feng, Tao Zhu, Bing Yang, et al.
Expert Systems with Applications (2025), pp. 127098-127098
Closed Access | Times Cited: 1
Feng Feng, Tao Zhu, Bing Yang, et al.
Expert Systems with Applications (2025), pp. 127098-127098
Closed Access | Times Cited: 1
High-cycle and very-high-cycle fatigue life prediction in additive manufacturing using hybrid physics-informed neural networks
Isaac Abiria, Chan Wang, Qicheng Zhang, et al.
Engineering Fracture Mechanics (2025), pp. 111026-111026
Closed Access | Times Cited: 1
Isaac Abiria, Chan Wang, Qicheng Zhang, et al.
Engineering Fracture Mechanics (2025), pp. 111026-111026
Closed Access | Times Cited: 1
Efficient prediction method of triple failure pressure for corroded pipelines under complex loads based on a backpropagation neural network
Tieyao Zhang, Jian Shuai, Yi Shuai, et al.
Reliability Engineering & System Safety (2022) Vol. 231, pp. 108990-108990
Closed Access | Times Cited: 34
Tieyao Zhang, Jian Shuai, Yi Shuai, et al.
Reliability Engineering & System Safety (2022) Vol. 231, pp. 108990-108990
Closed Access | Times Cited: 34
A generic physics-informed neural network-based framework for reliability assessment of multi-state systems
Taotao Zhou, Xiaoge Zhang, Enrique López Droguett, et al.
Reliability Engineering & System Safety (2022) Vol. 229, pp. 108835-108835
Open Access | Times Cited: 31
Taotao Zhou, Xiaoge Zhang, Enrique López Droguett, et al.
Reliability Engineering & System Safety (2022) Vol. 229, pp. 108835-108835
Open Access | Times Cited: 31
Defect driven physics-informed neural network framework for fatigue life prediction of additively manufactured materials
Lanyi Wang, Shun‐Peng Zhu, Changqi Luo, et al.
Philosophical Transactions of the Royal Society A Mathematical Physical and Engineering Sciences (2023) Vol. 381, Iss. 2260
Closed Access | Times Cited: 23
Lanyi Wang, Shun‐Peng Zhu, Changqi Luo, et al.
Philosophical Transactions of the Royal Society A Mathematical Physical and Engineering Sciences (2023) Vol. 381, Iss. 2260
Closed Access | Times Cited: 23
Recent developments and future trends in fatigue life assessment of additively manufactured metals with particular emphasis on machine learning modeling
Zhixin Zhan, Xiaofan He, Dingcheng Tang, et al.
Fatigue & Fracture of Engineering Materials & Structures (2023) Vol. 46, Iss. 12, pp. 4425-4464
Closed Access | Times Cited: 22
Zhixin Zhan, Xiaofan He, Dingcheng Tang, et al.
Fatigue & Fracture of Engineering Materials & Structures (2023) Vol. 46, Iss. 12, pp. 4425-4464
Closed Access | Times Cited: 22