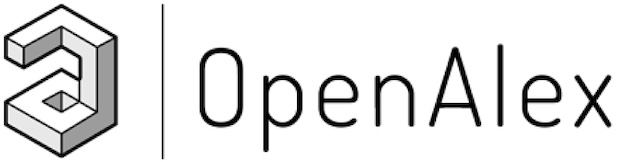
OpenAlex is a bibliographic catalogue of scientific papers, authors and institutions accessible in open access mode, named after the Library of Alexandria. It's citation coverage is excellent and I hope you will find utility in this listing of citing articles!
If you click the article title, you'll navigate to the article, as listed in CrossRef. If you click the Open Access links, you'll navigate to the "best Open Access location". Clicking the citation count will open this listing for that article. Lastly at the bottom of the page, you'll find basic pagination options.
Requested Article:
Physics-informed machine learning for low-cycle fatigue life prediction of 316 stainless steels
Lvfeng Jiang, Yanan Hu, Yuxuan Liu, et al.
International Journal of Fatigue (2024) Vol. 182, pp. 108187-108187
Closed Access | Times Cited: 28
Lvfeng Jiang, Yanan Hu, Yuxuan Liu, et al.
International Journal of Fatigue (2024) Vol. 182, pp. 108187-108187
Closed Access | Times Cited: 28
Showing 1-25 of 28 citing articles:
Neural network integrated with symbolic regression for multiaxial fatigue life prediction
Peng Zhang, Keke Tang, Anbin Wang, et al.
International Journal of Fatigue (2024) Vol. 188, pp. 108535-108535
Closed Access | Times Cited: 11
Peng Zhang, Keke Tang, Anbin Wang, et al.
International Journal of Fatigue (2024) Vol. 188, pp. 108535-108535
Closed Access | Times Cited: 11
Critical damage events of 3D printed AlSi10Mg alloy via in situ synchrotron X-ray tomography
Zhengkai Wu, Shengchuan Wu, Jamie J. Kruzic, et al.
Acta Materialia (2024) Vol. 282, pp. 120464-120464
Closed Access | Times Cited: 11
Zhengkai Wu, Shengchuan Wu, Jamie J. Kruzic, et al.
Acta Materialia (2024) Vol. 282, pp. 120464-120464
Closed Access | Times Cited: 11
Critical physics informed fatigue life prediction of laser 3D printed AlSi10Mg alloys with mass internal defects
Yanan Hu, Yufeng She, Shengchuan Wu, et al.
International Journal of Mechanical Sciences (2024), pp. 109730-109730
Closed Access | Times Cited: 9
Yanan Hu, Yufeng She, Shengchuan Wu, et al.
International Journal of Mechanical Sciences (2024), pp. 109730-109730
Closed Access | Times Cited: 9
High-cycle and very-high-cycle fatigue life prediction in additive manufacturing using hybrid physics-informed neural networks
Isaac Abiria, Chan Wang, Qicheng Zhang, et al.
Engineering Fracture Mechanics (2025), pp. 111026-111026
Closed Access | Times Cited: 1
Isaac Abiria, Chan Wang, Qicheng Zhang, et al.
Engineering Fracture Mechanics (2025), pp. 111026-111026
Closed Access | Times Cited: 1
Multi-fidelity physics-informed machine learning framework for fatigue life prediction of additive manufactured materials
Lanyi Wang, Shun‐Peng Zhu, Borui Wu, et al.
Computer Methods in Applied Mechanics and Engineering (2025) Vol. 439, pp. 117924-117924
Closed Access | Times Cited: 1
Lanyi Wang, Shun‐Peng Zhu, Borui Wu, et al.
Computer Methods in Applied Mechanics and Engineering (2025) Vol. 439, pp. 117924-117924
Closed Access | Times Cited: 1
Stored energy density solution for TSV-Cu structure deformation under thermal cyclic loading based on PINN
Hongjiang Qian, Jiebin Shen, Zhiyong Huang, et al.
International Journal of Plasticity (2024) Vol. 179, pp. 104046-104046
Closed Access | Times Cited: 7
Hongjiang Qian, Jiebin Shen, Zhiyong Huang, et al.
International Journal of Plasticity (2024) Vol. 179, pp. 104046-104046
Closed Access | Times Cited: 7
Physics-informed machine learning for loading history dependent fatigue delamination of composite laminates
Liaojun Yao, Jiexiong Wang, Mingyue Chuai, et al.
Composites Part A Applied Science and Manufacturing (2024), pp. 108474-108474
Closed Access | Times Cited: 6
Liaojun Yao, Jiexiong Wang, Mingyue Chuai, et al.
Composites Part A Applied Science and Manufacturing (2024), pp. 108474-108474
Closed Access | Times Cited: 6
Prediction of multiaxial fatigue life with a data-driven knowledge transfer model
Lei Gan, Zhi‐Ming Fan, Hao Wu, et al.
International Journal of Fatigue (2024), pp. 108636-108636
Closed Access | Times Cited: 4
Lei Gan, Zhi‐Ming Fan, Hao Wu, et al.
International Journal of Fatigue (2024), pp. 108636-108636
Closed Access | Times Cited: 4
Improving out-of-distribution generalization for online weld expulsion inspection using physics-informed neural networks使用物理信息神经网络改进在线焊缝飞溅检测的分布外泛化
Yu-Jun Xia, Qiang Song, B Yi, et al.
Welding in the World (2025)
Closed Access
Yu-Jun Xia, Qiang Song, B Yi, et al.
Welding in the World (2025)
Closed Access
Experimental and simulation study on the microstructural evolution and fatigue life of 316L stainless steel under different periodic overload conditions
Haifeng Zhai, Wei Jiang, Yang Wang, et al.
Engineering Failure Analysis (2025), pp. 109475-109475
Closed Access
Haifeng Zhai, Wei Jiang, Yang Wang, et al.
Engineering Failure Analysis (2025), pp. 109475-109475
Closed Access
Study of Hybrid Machine Learning Multiaxial Low‐Cycle Fatigue Life Prediction Model of CP‐Ti
Tian‐Hao Ma, Wei Zhang, Le Chang, et al.
Fatigue & Fracture of Engineering Materials & Structures (2025)
Closed Access
Tian‐Hao Ma, Wei Zhang, Le Chang, et al.
Fatigue & Fracture of Engineering Materials & Structures (2025)
Closed Access
Failure process and fracture surface analysis of aluminum-steel tubular joints fabricated by magnetic pulse crimping under fatigue loading
Yujia Zhao, Chang Chen, Guangyao Li, et al.
Journal of Materials Research and Technology (2025)
Open Access
Yujia Zhao, Chang Chen, Guangyao Li, et al.
Journal of Materials Research and Technology (2025)
Open Access
Study of LSTM-based constitutive modeling for coupled multimodal microstructure time-series damage in DZ125 superalloy
Yu-ming Ye, Weiqing Huang, Dongwei Li, et al.
International Journal of Fatigue (2025), pp. 109002-109002
Closed Access
Yu-ming Ye, Weiqing Huang, Dongwei Li, et al.
International Journal of Fatigue (2025), pp. 109002-109002
Closed Access
A path-dependent adaptive physics-informed neural network for multiaxial fatigue life prediction
H. Liao, Jun Pan, Xinren Su, et al.
International Journal of Fatigue (2024) Vol. 193, pp. 108799-108799
Closed Access | Times Cited: 3
H. Liao, Jun Pan, Xinren Su, et al.
International Journal of Fatigue (2024) Vol. 193, pp. 108799-108799
Closed Access | Times Cited: 3
Machine learning model for predicting the influence of crystallographic orientation on thermomechanical fatigue of Ni-base superalloys
Rohan Acharya, Alexander N. Caputo, Richard W. Neu
International Journal of Fatigue (2025), pp. 108832-108832
Closed Access
Rohan Acharya, Alexander N. Caputo, Richard W. Neu
International Journal of Fatigue (2025), pp. 108832-108832
Closed Access
Data-driven nonlocal damage mechanics and fracture of shells
Daoping Liu, Xuejiao Shao, Xiaolong Fu, et al.
Engineering Fracture Mechanics (2025), pp. 110864-110864
Closed Access
Daoping Liu, Xuejiao Shao, Xiaolong Fu, et al.
Engineering Fracture Mechanics (2025), pp. 110864-110864
Closed Access
A Novel Physical Neural Network Based on Transformer Framework for Multiaxial Fatigue Life Prediction
Rui Pan, Jianxiong Gao, Yiping Yuan, et al.
Fatigue & Fracture of Engineering Materials & Structures (2025)
Open Access
Rui Pan, Jianxiong Gao, Yiping Yuan, et al.
Fatigue & Fracture of Engineering Materials & Structures (2025)
Open Access
A general physics-informed neural network framework for fatigue life prediction of metallic materials
Shuwei Zhou, Manuel Henrich, Zhichao Wei, et al.
Engineering Fracture Mechanics (2025), pp. 111136-111136
Closed Access
Shuwei Zhou, Manuel Henrich, Zhichao Wei, et al.
Engineering Fracture Mechanics (2025), pp. 111136-111136
Closed Access
Fatigue life prediction of aluminum-steel magnetic pulse crimped joints based on point cloud measurement and gradient boosting regression trees
Yujia Zhao, Ming Yong Lai, Yuqi Wu, et al.
International Journal of Fatigue (2025), pp. 109020-109020
Closed Access
Yujia Zhao, Ming Yong Lai, Yuqi Wu, et al.
International Journal of Fatigue (2025), pp. 109020-109020
Closed Access
A cGAN-based fatigue life prediction of 316 austenitic stainless steel in high-temperature and high-pressure water environments
Lvfeng Jiang, Yanan Hu, Hui Li, et al.
International Journal of Fatigue (2024), pp. 108633-108633
Closed Access | Times Cited: 2
Lvfeng Jiang, Yanan Hu, Hui Li, et al.
International Journal of Fatigue (2024), pp. 108633-108633
Closed Access | Times Cited: 2
A modified physics-informed neural network to fatigue life prediction of deck-rib double-side welded joints
Xincheng Li, Zhongqiu Fu, Jiakai Shu, et al.
International Journal of Fatigue (2024) Vol. 189, pp. 108566-108566
Closed Access | Times Cited: 1
Xincheng Li, Zhongqiu Fu, Jiakai Shu, et al.
International Journal of Fatigue (2024) Vol. 189, pp. 108566-108566
Closed Access | Times Cited: 1
A stacking ensemble model for predicting the flexural fatigue life of fiber-reinforced concrete
Wan-lin Min, Weiliang Jin, Yen-yi Hoo, et al.
International Journal of Fatigue (2024) Vol. 190, pp. 108599-108599
Closed Access | Times Cited: 1
Wan-lin Min, Weiliang Jin, Yen-yi Hoo, et al.
International Journal of Fatigue (2024) Vol. 190, pp. 108599-108599
Closed Access | Times Cited: 1
Machine learning for ULCF life prediction of structural steels with synthetic data
Mingming Yu, Shuailing Li, Xu Xie
Journal of Constructional Steel Research (2024) Vol. 224, pp. 109152-109152
Closed Access | Times Cited: 1
Mingming Yu, Shuailing Li, Xu Xie
Journal of Constructional Steel Research (2024) Vol. 224, pp. 109152-109152
Closed Access | Times Cited: 1
Graphical Feature Construction-Based Deep Learning Model for Fatigue Life Prediction of AM Alloys
Hao Wu, Anbin Wang, Zhiqiang Gan, et al.
Materials (2024) Vol. 18, Iss. 1, pp. 11-11
Open Access | Times Cited: 1
Hao Wu, Anbin Wang, Zhiqiang Gan, et al.
Materials (2024) Vol. 18, Iss. 1, pp. 11-11
Open Access | Times Cited: 1
Study of Lstm-Based Constitutive Modeling for Coupled Multimodal Microstructure Time-Series Damage in Dz125 Superalloy
Dongwei Li, Jinxiang Liu, Weiqing Huang, et al.
(2024)
Closed Access
Dongwei Li, Jinxiang Liu, Weiqing Huang, et al.
(2024)
Closed Access