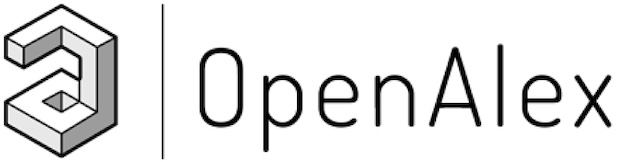
OpenAlex is a bibliographic catalogue of scientific papers, authors and institutions accessible in open access mode, named after the Library of Alexandria. It's citation coverage is excellent and I hope you will find utility in this listing of citing articles!
If you click the article title, you'll navigate to the article, as listed in CrossRef. If you click the Open Access links, you'll navigate to the "best Open Access location". Clicking the citation count will open this listing for that article. Lastly at the bottom of the page, you'll find basic pagination options.
Requested Article:
Using Machine-Learning for Prediction of the Response to Cardiac Resynchronization Therapy
Stacey J. Howell, Tim Stivland, Kenneth M. Steín, et al.
JACC. Clinical electrophysiology (2021) Vol. 7, Iss. 12, pp. 1505-1515
Open Access | Times Cited: 18
Stacey J. Howell, Tim Stivland, Kenneth M. Steín, et al.
JACC. Clinical electrophysiology (2021) Vol. 7, Iss. 12, pp. 1505-1515
Open Access | Times Cited: 18
Showing 18 citing articles:
Artificial Intelligence for Clinical Prediction: Exploring Key Domains and Essential Functions
Mohamed Khalifa, Mona Albadawy
Computer Methods and Programs in Biomedicine Update (2024) Vol. 5, pp. 100148-100148
Open Access | Times Cited: 46
Mohamed Khalifa, Mona Albadawy
Computer Methods and Programs in Biomedicine Update (2024) Vol. 5, pp. 100148-100148
Open Access | Times Cited: 46
Artificial Intelligence in Heart Failure: Friend or Foe?
Angeliki Bourazana, Αndrew Xanthopoulos, Αlexandros Briasoulis, et al.
Life (2024) Vol. 14, Iss. 1, pp. 145-145
Open Access | Times Cited: 15
Angeliki Bourazana, Αndrew Xanthopoulos, Αlexandros Briasoulis, et al.
Life (2024) Vol. 14, Iss. 1, pp. 145-145
Open Access | Times Cited: 15
Quest for the ideal assessment of electrical ventricular dyssynchrony in cardiac resynchronization therapy
Uyên Châu Nguyên, Kevin Vernooy, Frits W. Prinzen
Journal of Molecular and Cellular Cardiology Plus (2024) Vol. 7, pp. 100061-100061
Open Access | Times Cited: 4
Uyên Châu Nguyên, Kevin Vernooy, Frits W. Prinzen
Journal of Molecular and Cellular Cardiology Plus (2024) Vol. 7, pp. 100061-100061
Open Access | Times Cited: 4
No-Reflow Prediction in Acute Coronary Syndrome During Percutaneous Coronary Intervention: The NORPACS Risk Score
L. Dawson, Muhammad Rashid, Diem Dinh, et al.
Circulation Cardiovascular Interventions (2024) Vol. 17, Iss. 4
Closed Access | Times Cited: 4
L. Dawson, Muhammad Rashid, Diem Dinh, et al.
Circulation Cardiovascular Interventions (2024) Vol. 17, Iss. 4
Closed Access | Times Cited: 4
Artificial intelligence models in prediction of response to cardiac resynchronization therapy: a systematic review
Wojciech Nazar, Stanisław Szymanowicz, Krzysztof Nazar, et al.
Heart Failure Reviews (2023) Vol. 29, Iss. 1, pp. 133-150
Open Access | Times Cited: 9
Wojciech Nazar, Stanisław Szymanowicz, Krzysztof Nazar, et al.
Heart Failure Reviews (2023) Vol. 29, Iss. 1, pp. 133-150
Open Access | Times Cited: 9
Machine learning for multidimensional response and survival after cardiac resynchronization therapy using features from cardiac magnetic resonance
Derek Bivona, Srikar Tallavajhala, Mohamad Abdi, et al.
Heart Rhythm O2 (2022) Vol. 3, Iss. 5, pp. 542-552
Open Access | Times Cited: 13
Derek Bivona, Srikar Tallavajhala, Mohamad Abdi, et al.
Heart Rhythm O2 (2022) Vol. 3, Iss. 5, pp. 542-552
Open Access | Times Cited: 13
Machine Learning and Deep Learning Methods for Fast and Accurate Assessment of Transthoracic Echocardiogram Image Quality
Wojciech Nazar, Krzysztof Nazar, Ludmiła Daniłowicz‐Szymanowicz
Life (2024) Vol. 14, Iss. 6, pp. 761-761
Open Access | Times Cited: 2
Wojciech Nazar, Krzysztof Nazar, Ludmiła Daniłowicz‐Szymanowicz
Life (2024) Vol. 14, Iss. 6, pp. 761-761
Open Access | Times Cited: 2
Electrocardiogram Interpretation Using Artificial Intelligence: Diagnosis of Cardiac and Extracardiac Pathologic Conditions. How Far Has Machine Learning Reached?
Gabriela Răileanu, Jonas S.S.G. de Jong
Current Problems in Cardiology (2023) Vol. 49, Iss. 1, pp. 102097-102097
Closed Access | Times Cited: 4
Gabriela Răileanu, Jonas S.S.G. de Jong
Current Problems in Cardiology (2023) Vol. 49, Iss. 1, pp. 102097-102097
Closed Access | Times Cited: 4
Interpretable machine learning predicts cardiac resynchronization therapy responses from personalized biochemical and biomechanical features
Anamul Haque, Doug Stubbs, Nina Hubig, et al.
BMC Medical Informatics and Decision Making (2022) Vol. 22, Iss. 1
Open Access | Times Cited: 7
Anamul Haque, Doug Stubbs, Nina Hubig, et al.
BMC Medical Informatics and Decision Making (2022) Vol. 22, Iss. 1
Open Access | Times Cited: 7
The Use of Machine Learning Algorithms in the Evaluation of the Effectiveness of Resynchronization Therapy
Bartosz Krzowski, Jakub Rokicki, Renata Główczyńska, et al.
Journal of Cardiovascular Development and Disease (2022) Vol. 9, Iss. 1, pp. 17-17
Open Access | Times Cited: 4
Bartosz Krzowski, Jakub Rokicki, Renata Główczyńska, et al.
Journal of Cardiovascular Development and Disease (2022) Vol. 9, Iss. 1, pp. 17-17
Open Access | Times Cited: 4
Primer on Machine Learning in Electrophysiology
Shane Loeffler, Natalia A. Trayanova
Arrhythmia & Electrophysiology Review (2023) Vol. 12
Open Access | Times Cited: 2
Shane Loeffler, Natalia A. Trayanova
Arrhythmia & Electrophysiology Review (2023) Vol. 12
Open Access | Times Cited: 2
Artificial Intelligence and Machine Learning in Electrophysiology—a Short Review
Shahrukh Khan, Chanho Lim, Humza A Chaudhry, et al.
Current Treatment Options in Cardiovascular Medicine (2023) Vol. 25, Iss. 10, pp. 443-460
Closed Access | Times Cited: 1
Shahrukh Khan, Chanho Lim, Humza A Chaudhry, et al.
Current Treatment Options in Cardiovascular Medicine (2023) Vol. 25, Iss. 10, pp. 443-460
Closed Access | Times Cited: 1
Machine learning-based prediction of 1-year all-cause mortality in patients undergoing CRT implantation: Validation of the SEMMELWEIS-CRT score in the European CRT Survey I dataset
Márton Tokodi, Annamária Kosztin, Attila Kovács, et al.
European Heart Journal - Digital Health (2024) Vol. 5, Iss. 5, pp. 563-571
Open Access
Márton Tokodi, Annamária Kosztin, Attila Kovács, et al.
European Heart Journal - Digital Health (2024) Vol. 5, Iss. 5, pp. 563-571
Open Access
Role of Artificial Intelligence and Machine Learning to Create Predictors, Enhance Molecular Understanding, and Implement Purposeful Programs for Myocardial Recovery
Frederick Lang, Benjamin C. Lee, Dor Lotan, et al.
Methodist DeBakey Cardiovascular Journal (2024) Vol. 20, Iss. 4, pp. 76-87
Open Access
Frederick Lang, Benjamin C. Lee, Dor Lotan, et al.
Methodist DeBakey Cardiovascular Journal (2024) Vol. 20, Iss. 4, pp. 76-87
Open Access
Mathematical models and artificial intelligence for studying the ventricular dyssynchrony and improving the effectiveness of cardiac resynchronization therapy
Tatiana Nesterova, Vasily Kabak, Д. С. Лебедев, et al.
Russian Journal of Cardiology (2024) Vol. 29, Iss. 4S, pp. 6194-6194
Open Access
Tatiana Nesterova, Vasily Kabak, Д. С. Лебедев, et al.
Russian Journal of Cardiology (2024) Vol. 29, Iss. 4S, pp. 6194-6194
Open Access
First-in-human noninvasive left ventricular ultrasound pacing: A potential screening tool for cardiac resynchronization therapy
Kenneth C. Bilchick, Ekaterina Morgounova, Pim J. A. Oomen, et al.
Heart Rhythm O2 (2022) Vol. 4, Iss. 2, pp. 79-87
Open Access | Times Cited: 1
Kenneth C. Bilchick, Ekaterina Morgounova, Pim J. A. Oomen, et al.
Heart Rhythm O2 (2022) Vol. 4, Iss. 2, pp. 79-87
Open Access | Times Cited: 1
REPLY
Larisa G. Tereshchenko, Stacey J. Howell, Timothy M. Stivland, et al.
JACC. Clinical electrophysiology (2021) Vol. 7, Iss. 12, pp. 1623-1624
Open Access | Times Cited: 1
Larisa G. Tereshchenko, Stacey J. Howell, Timothy M. Stivland, et al.
JACC. Clinical electrophysiology (2021) Vol. 7, Iss. 12, pp. 1623-1624
Open Access | Times Cited: 1
Updated insights into predictive biomarkers for response to cardiac resynchronization therapy - A literature review
Krisztina Pál, Ionela M. Cotoi, Georgiana G. Vlad, et al.
Revista română de medicină de laborator (2023) Vol. 31, Iss. 4, pp. 225-240
Open Access
Krisztina Pál, Ionela M. Cotoi, Georgiana G. Vlad, et al.
Revista română de medicină de laborator (2023) Vol. 31, Iss. 4, pp. 225-240
Open Access