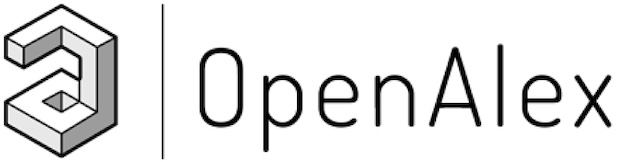
OpenAlex is a bibliographic catalogue of scientific papers, authors and institutions accessible in open access mode, named after the Library of Alexandria. It's citation coverage is excellent and I hope you will find utility in this listing of citing articles!
If you click the article title, you'll navigate to the article, as listed in CrossRef. If you click the Open Access links, you'll navigate to the "best Open Access location". Clicking the citation count will open this listing for that article. Lastly at the bottom of the page, you'll find basic pagination options.
Requested Article:
Predictive modeling for sustainable high-performance concrete from industrial wastes: A comparison and optimization of models using ensemble learners
Furqan Farooq, Wisal Ahmed, Arslan Akbar, et al.
Journal of Cleaner Production (2021) Vol. 292, pp. 126032-126032
Closed Access | Times Cited: 323
Furqan Farooq, Wisal Ahmed, Arslan Akbar, et al.
Journal of Cleaner Production (2021) Vol. 292, pp. 126032-126032
Closed Access | Times Cited: 323
Showing 1-25 of 323 citing articles:
A survey on deep learning tools dealing with data scarcity: definitions, challenges, solutions, tips, and applications
Laith Alzubaidi, Jinshuai Bai, Aiman Al-Sabaawi, et al.
Journal Of Big Data (2023) Vol. 10, Iss. 1
Open Access | Times Cited: 366
Laith Alzubaidi, Jinshuai Bai, Aiman Al-Sabaawi, et al.
Journal Of Big Data (2023) Vol. 10, Iss. 1
Open Access | Times Cited: 366
Predicting the compressive strength of concrete with fly ash admixture using machine learning algorithms
Hongwei Song, Ayaz Ahmad, Furqan Farooq, et al.
Construction and Building Materials (2021) Vol. 308, pp. 125021-125021
Open Access | Times Cited: 285
Hongwei Song, Ayaz Ahmad, Furqan Farooq, et al.
Construction and Building Materials (2021) Vol. 308, pp. 125021-125021
Open Access | Times Cited: 285
Compressive strength prediction of fly ash-based geopolymer concrete via advanced machine learning techniques
Ayaz Ahmad, Waqas Ahmad, Fahid Aslam, et al.
Case Studies in Construction Materials (2021) Vol. 16, pp. e00840-e00840
Open Access | Times Cited: 237
Ayaz Ahmad, Waqas Ahmad, Fahid Aslam, et al.
Case Studies in Construction Materials (2021) Vol. 16, pp. e00840-e00840
Open Access | Times Cited: 237
Prediction of Compressive Strength of Fly Ash Based Concrete Using Individual and Ensemble Algorithm
Ayaz Ahmad, Furqan Farooq, Paweł Niewiadomski, et al.
Materials (2021) Vol. 14, Iss. 4, pp. 794-794
Open Access | Times Cited: 206
Ayaz Ahmad, Furqan Farooq, Paweł Niewiadomski, et al.
Materials (2021) Vol. 14, Iss. 4, pp. 794-794
Open Access | Times Cited: 206
Comparative Study of Supervised Machine Learning Algorithms for Predicting the Compressive Strength of Concrete at High Temperature
Ayaz Ahmad, Krzysztof Adam Ostrowski, Mariusz Maślak, et al.
Materials (2021) Vol. 14, Iss. 15, pp. 4222-4222
Open Access | Times Cited: 159
Ayaz Ahmad, Krzysztof Adam Ostrowski, Mariusz Maślak, et al.
Materials (2021) Vol. 14, Iss. 15, pp. 4222-4222
Open Access | Times Cited: 159
Hybrid machine learning model and Shapley additive explanations for compressive strength of sustainable concrete
Yanqi Wu, Yisong Zhou
Construction and Building Materials (2022) Vol. 330, pp. 127298-127298
Closed Access | Times Cited: 139
Yanqi Wu, Yisong Zhou
Construction and Building Materials (2022) Vol. 330, pp. 127298-127298
Closed Access | Times Cited: 139
Predictive Modeling of Mechanical Properties of Silica Fume-Based Green Concrete Using Artificial Intelligence Approaches: MLPNN, ANFIS, and GEP
Afnan Nafees, Muhammad Faisal Javed, Sherbaz Khan, et al.
Materials (2021) Vol. 14, Iss. 24, pp. 7531-7531
Open Access | Times Cited: 134
Afnan Nafees, Muhammad Faisal Javed, Sherbaz Khan, et al.
Materials (2021) Vol. 14, Iss. 24, pp. 7531-7531
Open Access | Times Cited: 134
Geopolymer Concrete Compressive Strength via Artificial Neural Network, Adaptive Neuro Fuzzy Interface System, and Gene Expression Programming With K-Fold Cross Validation
Mohsin Ali Khan, Adeel Zafar, Furqan Farooq, et al.
Frontiers in Materials (2021) Vol. 8
Open Access | Times Cited: 116
Mohsin Ali Khan, Adeel Zafar, Furqan Farooq, et al.
Frontiers in Materials (2021) Vol. 8
Open Access | Times Cited: 116
Analyzing the mechanical performance of fly ash-based geopolymer concrete with different machine learning techniques
Yiming Peng, Cise Unluer
Construction and Building Materials (2021) Vol. 316, pp. 125785-125785
Open Access | Times Cited: 116
Yiming Peng, Cise Unluer
Construction and Building Materials (2021) Vol. 316, pp. 125785-125785
Open Access | Times Cited: 116
Prediction of Geopolymer Concrete Compressive Strength Using Novel Machine Learning Algorithms
Ayaz Ahmad, Waqas Ahmad, Krisada Chaiyasarn, et al.
Polymers (2021) Vol. 13, Iss. 19, pp. 3389-3389
Open Access | Times Cited: 114
Ayaz Ahmad, Waqas Ahmad, Krisada Chaiyasarn, et al.
Polymers (2021) Vol. 13, Iss. 19, pp. 3389-3389
Open Access | Times Cited: 114
Predictive modeling of compressive strength of sustainable rice husk ash concrete: Ensemble learner optimization and comparison
Bawar Iftikhar, Sophia C. Alih, Mohammadreza Vafaei, et al.
Journal of Cleaner Production (2022) Vol. 348, pp. 131285-131285
Closed Access | Times Cited: 109
Bawar Iftikhar, Sophia C. Alih, Mohammadreza Vafaei, et al.
Journal of Cleaner Production (2022) Vol. 348, pp. 131285-131285
Closed Access | Times Cited: 109
Enhancing property prediction and process optimization in building materials through machine learning: A review
Konstantinos I. Stergiou, Charis Ntakolia, Paris Varytis, et al.
Computational Materials Science (2023) Vol. 220, pp. 112031-112031
Open Access | Times Cited: 97
Konstantinos I. Stergiou, Charis Ntakolia, Paris Varytis, et al.
Computational Materials Science (2023) Vol. 220, pp. 112031-112031
Open Access | Times Cited: 97
Prediction of compressive strength of rice husk ash concrete based on stacking ensemble learning model
Qingfu Li, Zongming Song
Journal of Cleaner Production (2022) Vol. 382, pp. 135279-135279
Closed Access | Times Cited: 92
Qingfu Li, Zongming Song
Journal of Cleaner Production (2022) Vol. 382, pp. 135279-135279
Closed Access | Times Cited: 92
Prediction model for rice husk ash concrete using AI approach: Boosting and bagging algorithms
Muhammad Nasir Amin, Bawar Iftikhar, Kaffayatullah Khan, et al.
Structures (2023) Vol. 50, pp. 745-757
Closed Access | Times Cited: 88
Muhammad Nasir Amin, Bawar Iftikhar, Kaffayatullah Khan, et al.
Structures (2023) Vol. 50, pp. 745-757
Closed Access | Times Cited: 88
Predicting the Mechanical Properties of RCA-Based Concrete Using Supervised Machine Learning Algorithms
Meijun Shang, Hejun Li, Ayaz Ahmad, et al.
Materials (2022) Vol. 15, Iss. 2, pp. 647-647
Open Access | Times Cited: 84
Meijun Shang, Hejun Li, Ayaz Ahmad, et al.
Materials (2022) Vol. 15, Iss. 2, pp. 647-647
Open Access | Times Cited: 84
Durability Studies on Fly Ash Based Geopolymer Concrete Incorporated with Slag and Alkali Solutions
S. Nagajothi, S. Elavenil, S. Angalaeswari, et al.
Advances in Civil Engineering (2022) Vol. 2022, Iss. 1
Open Access | Times Cited: 84
S. Nagajothi, S. Elavenil, S. Angalaeswari, et al.
Advances in Civil Engineering (2022) Vol. 2022, Iss. 1
Open Access | Times Cited: 84
Machine learning method to predict dynamic compressive response of concrete-like material at high strain rates
Xu Long, Minghui Mao, Tianxiong Su, et al.
Defence Technology (2022) Vol. 23, pp. 100-111
Open Access | Times Cited: 81
Xu Long, Minghui Mao, Tianxiong Su, et al.
Defence Technology (2022) Vol. 23, pp. 100-111
Open Access | Times Cited: 81
Forecasting the Mechanical Properties of Plastic Concrete Employing Experimental Data Using Machine Learning Algorithms: DT, MLPNN, SVM, and RF
Afnan Nafees, Sherbaz Khan, Muhammad Faisal Javed, et al.
Polymers (2022) Vol. 14, Iss. 8, pp. 1583-1583
Open Access | Times Cited: 81
Afnan Nafees, Sherbaz Khan, Muhammad Faisal Javed, et al.
Polymers (2022) Vol. 14, Iss. 8, pp. 1583-1583
Open Access | Times Cited: 81
Predicting compressive strength of high-performance concrete with high volume ground granulated blast-furnace slag replacement using boosting machine learning algorithms
Vimal Rathakrishnan, Salmia Beddu, Ali Najah Ahmed
Scientific Reports (2022) Vol. 12, Iss. 1
Open Access | Times Cited: 80
Vimal Rathakrishnan, Salmia Beddu, Ali Najah Ahmed
Scientific Reports (2022) Vol. 12, Iss. 1
Open Access | Times Cited: 80
Data quantity governance for machine learning in materials science
Yue Liu, Zhengwei Yang, Xinxin Zou, et al.
National Science Review (2023) Vol. 10, Iss. 7
Open Access | Times Cited: 80
Yue Liu, Zhengwei Yang, Xinxin Zou, et al.
National Science Review (2023) Vol. 10, Iss. 7
Open Access | Times Cited: 80
Machine Learning Prediction Models to Evaluate the Strength of Recycled Aggregate Concrete
Xiongzhou Yuan, Yuze Tian, Waqas Ahmad, et al.
Materials (2022) Vol. 15, Iss. 8, pp. 2823-2823
Open Access | Times Cited: 77
Xiongzhou Yuan, Yuze Tian, Waqas Ahmad, et al.
Materials (2022) Vol. 15, Iss. 8, pp. 2823-2823
Open Access | Times Cited: 77
Machine learning interpretable-prediction models to evaluate the slump and strength of fly ash-based geopolymer
Sohaib Nazar, Jian Yang, Muhammad Nasir Amin, et al.
Journal of Materials Research and Technology (2023) Vol. 24, pp. 100-124
Open Access | Times Cited: 69
Sohaib Nazar, Jian Yang, Muhammad Nasir Amin, et al.
Journal of Materials Research and Technology (2023) Vol. 24, pp. 100-124
Open Access | Times Cited: 69
Prediction and optimization model of sustainable concrete properties using machine learning, deep learning and swarm intelligence: A review
Shiqi Wang, Peng Xia, Keyu Chen, et al.
Journal of Building Engineering (2023) Vol. 80, pp. 108065-108065
Closed Access | Times Cited: 65
Shiqi Wang, Peng Xia, Keyu Chen, et al.
Journal of Building Engineering (2023) Vol. 80, pp. 108065-108065
Closed Access | Times Cited: 65
Predicting compressive strength of geopolymer concrete using machine learning
Priyanka Gupta, Nakul Gupta, Kuldeep K. Saxena
Innovation and Emerging Technologies (2023) Vol. 10
Closed Access | Times Cited: 55
Priyanka Gupta, Nakul Gupta, Kuldeep K. Saxena
Innovation and Emerging Technologies (2023) Vol. 10
Closed Access | Times Cited: 55
Hybrid machine learning approach to prediction of the compressive and flexural strengths of UHPC and parametric analysis with shapley additive explanations
Pobithra Das, Abul Kashem
Case Studies in Construction Materials (2023) Vol. 20, pp. e02723-e02723
Open Access | Times Cited: 48
Pobithra Das, Abul Kashem
Case Studies in Construction Materials (2023) Vol. 20, pp. e02723-e02723
Open Access | Times Cited: 48