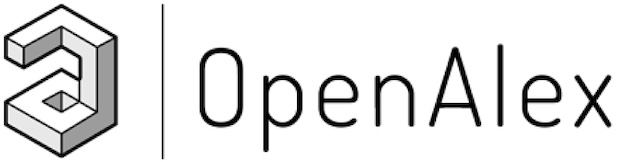
OpenAlex is a bibliographic catalogue of scientific papers, authors and institutions accessible in open access mode, named after the Library of Alexandria. It's citation coverage is excellent and I hope you will find utility in this listing of citing articles!
If you click the article title, you'll navigate to the article, as listed in CrossRef. If you click the Open Access links, you'll navigate to the "best Open Access location". Clicking the citation count will open this listing for that article. Lastly at the bottom of the page, you'll find basic pagination options.
Requested Article:
Intelligent tool wear monitoring and multi-step prediction based on deep learning model
Minghui Cheng, Jiao Li, Pei Yan, et al.
Journal of Manufacturing Systems (2021) Vol. 62, pp. 286-300
Closed Access | Times Cited: 131
Minghui Cheng, Jiao Li, Pei Yan, et al.
Journal of Manufacturing Systems (2021) Vol. 62, pp. 286-300
Closed Access | Times Cited: 131
Showing 1-25 of 131 citing articles:
Artificial intelligence systems for tool condition monitoring in machining: analysis and critical review
Danil Yurievich Pimenov, Andrés Bustillo, Szymon Wojciechowski, et al.
Journal of Intelligent Manufacturing (2022) Vol. 34, Iss. 5, pp. 2079-2121
Closed Access | Times Cited: 224
Danil Yurievich Pimenov, Andrés Bustillo, Szymon Wojciechowski, et al.
Journal of Intelligent Manufacturing (2022) Vol. 34, Iss. 5, pp. 2079-2121
Closed Access | Times Cited: 224
Hybrid robust convolutional autoencoder for unsupervised anomaly detection of machine tools under noises
Shen Yan, Haidong Shao, Yiming Xiao, et al.
Robotics and Computer-Integrated Manufacturing (2022) Vol. 79, pp. 102441-102441
Open Access | Times Cited: 143
Shen Yan, Haidong Shao, Yiming Xiao, et al.
Robotics and Computer-Integrated Manufacturing (2022) Vol. 79, pp. 102441-102441
Open Access | Times Cited: 143
Data-model linkage prediction of tool remaining useful life based on deep feature fusion and Wiener process
Xuebing Li, Xianli Liu, Caixu Yue, et al.
Journal of Manufacturing Systems (2024) Vol. 73, pp. 19-38
Closed Access | Times Cited: 23
Xuebing Li, Xianli Liu, Caixu Yue, et al.
Journal of Manufacturing Systems (2024) Vol. 73, pp. 19-38
Closed Access | Times Cited: 23
Tool wear state recognition and prediction method based on laplacian eigenmap with ensemble learning model
Yang Xie, Shangshang Gao, Chaoyong Zhang, et al.
Advanced Engineering Informatics (2024) Vol. 60, pp. 102382-102382
Closed Access | Times Cited: 16
Yang Xie, Shangshang Gao, Chaoyong Zhang, et al.
Advanced Engineering Informatics (2024) Vol. 60, pp. 102382-102382
Closed Access | Times Cited: 16
Intelligent monitoring system for production lines in smart factories: A hybrid method integrating Transformer and Kalman filter
Xuzhou Fang, Qinghua Song, Zhenyang Li, et al.
Journal of Manufacturing Systems (2025) Vol. 79, pp. 27-47
Closed Access | Times Cited: 2
Xuzhou Fang, Qinghua Song, Zhenyang Li, et al.
Journal of Manufacturing Systems (2025) Vol. 79, pp. 27-47
Closed Access | Times Cited: 2
A Hybrid Attention-Based Paralleled Deep Learning model for tool wear prediction
Jian Duan, Xi Zhang, Tielin Shi
Expert Systems with Applications (2022) Vol. 211, pp. 118548-118548
Closed Access | Times Cited: 68
Jian Duan, Xi Zhang, Tielin Shi
Expert Systems with Applications (2022) Vol. 211, pp. 118548-118548
Closed Access | Times Cited: 68
A milling tool wear monitoring method with sensing generalization capability
Runqiong Wang, Qinghua Song, Yezhen Peng, et al.
Journal of Manufacturing Systems (2023) Vol. 68, pp. 25-41
Closed Access | Times Cited: 29
Runqiong Wang, Qinghua Song, Yezhen Peng, et al.
Journal of Manufacturing Systems (2023) Vol. 68, pp. 25-41
Closed Access | Times Cited: 29
Intelligent tool wear monitoring based on multi-channel hybrid information and deep transfer learning
Pengfei Zhang, Dong Gao, Dongbo Hong, et al.
Journal of Manufacturing Systems (2023) Vol. 69, pp. 31-47
Closed Access | Times Cited: 29
Pengfei Zhang, Dong Gao, Dongbo Hong, et al.
Journal of Manufacturing Systems (2023) Vol. 69, pp. 31-47
Closed Access | Times Cited: 29
Artificial intelligence-based data-driven prognostics in industry: A survey
Mohamed A. El-Brawany, Dina A. Ibrahim, Hamdy K. Elminir, et al.
Computers & Industrial Engineering (2023) Vol. 184, pp. 109605-109605
Closed Access | Times Cited: 23
Mohamed A. El-Brawany, Dina A. Ibrahim, Hamdy K. Elminir, et al.
Computers & Industrial Engineering (2023) Vol. 184, pp. 109605-109605
Closed Access | Times Cited: 23
Digital Twins Enabling Intelligent Manufacturing: From Methodology to Application
Shuguo Hu, Changhe Li, Benkai Li, et al.
Intelligent and sustainable manufacturing (2024) Vol. 1, Iss. 1, pp. 10007-10007
Open Access | Times Cited: 15
Shuguo Hu, Changhe Li, Benkai Li, et al.
Intelligent and sustainable manufacturing (2024) Vol. 1, Iss. 1, pp. 10007-10007
Open Access | Times Cited: 15
An online monitoring method of milling cutter wear condition driven by digital twin
Xintian Zi, Shangshang Gao, Yang Xie
Scientific Reports (2024) Vol. 14, Iss. 1
Open Access | Times Cited: 12
Xintian Zi, Shangshang Gao, Yang Xie
Scientific Reports (2024) Vol. 14, Iss. 1
Open Access | Times Cited: 12
Research on tool wear state identification method driven by multi-source information fusion and multi-dimension attention mechanism
Peining Wei, Rongyi Li, Xianli Liu, et al.
Robotics and Computer-Integrated Manufacturing (2024) Vol. 88, pp. 102741-102741
Closed Access | Times Cited: 10
Peining Wei, Rongyi Li, Xianli Liu, et al.
Robotics and Computer-Integrated Manufacturing (2024) Vol. 88, pp. 102741-102741
Closed Access | Times Cited: 10
Review of advances in tool condition monitoring techniques in the milling process
T. Mohanraj, E S Kirubakaran, Dinesh Kumar Madheswaran, et al.
Measurement Science and Technology (2024) Vol. 35, Iss. 9, pp. 092002-092002
Closed Access | Times Cited: 10
T. Mohanraj, E S Kirubakaran, Dinesh Kumar Madheswaran, et al.
Measurement Science and Technology (2024) Vol. 35, Iss. 9, pp. 092002-092002
Closed Access | Times Cited: 10
Multi-sensor signal fusion for tool wear condition monitoring using denoising transformer auto-encoder Resnet
Hui Wang, Shuhui Wang, Weifang Sun, et al.
Journal of Manufacturing Processes (2024) Vol. 124, pp. 1054-1064
Closed Access | Times Cited: 10
Hui Wang, Shuhui Wang, Weifang Sun, et al.
Journal of Manufacturing Processes (2024) Vol. 124, pp. 1054-1064
Closed Access | Times Cited: 10
Improving milling tool wear prediction through a hybrid NCA-SMA-GRU deep learning model
Zhongyuan Che, Chong Peng, T. Warren Liao, et al.
Expert Systems with Applications (2024) Vol. 255, pp. 124556-124556
Closed Access | Times Cited: 9
Zhongyuan Che, Chong Peng, T. Warren Liao, et al.
Expert Systems with Applications (2024) Vol. 255, pp. 124556-124556
Closed Access | Times Cited: 9
A Physics-Guided RNN-KAN for Multi-Step Prediction of Heat Pump Operation States
Siyi Guo, Ziqing Wei, Yaling Yin, et al.
Energy (2025), pp. 135108-135108
Closed Access | Times Cited: 1
Siyi Guo, Ziqing Wei, Yaling Yin, et al.
Energy (2025), pp. 135108-135108
Closed Access | Times Cited: 1
Tool wear state recognition study based on an MTF and a vision transformer with a Kolmogorov-Arnold network
Shengming Dong, Meng Yue, Shubin Yin, et al.
Mechanical Systems and Signal Processing (2025) Vol. 228, pp. 112473-112473
Closed Access | Times Cited: 1
Shengming Dong, Meng Yue, Shubin Yin, et al.
Mechanical Systems and Signal Processing (2025) Vol. 228, pp. 112473-112473
Closed Access | Times Cited: 1
A novel adversarial domain adaptation transfer learning method for tool wear state prediction
Kai Li, Ming-Song Chen, Y.C. Lin, et al.
Knowledge-Based Systems (2022) Vol. 254, pp. 109537-109537
Closed Access | Times Cited: 37
Kai Li, Ming-Song Chen, Y.C. Lin, et al.
Knowledge-Based Systems (2022) Vol. 254, pp. 109537-109537
Closed Access | Times Cited: 37
Prediction and evaluation of surface roughness with hybrid kernel extreme learning machine and monitored tool wear
Minghui Cheng, Li Jiao, Pei Yan, et al.
Journal of Manufacturing Processes (2022) Vol. 84, pp. 1541-1556
Closed Access | Times Cited: 32
Minghui Cheng, Li Jiao, Pei Yan, et al.
Journal of Manufacturing Processes (2022) Vol. 84, pp. 1541-1556
Closed Access | Times Cited: 32
Online monitoring model of micro-milling force incorporating tool wear prediction process
Pengfei Ding, Xianzhen Huang, Chengying Zhao, et al.
Expert Systems with Applications (2023) Vol. 223, pp. 119886-119886
Closed Access | Times Cited: 22
Pengfei Ding, Xianzhen Huang, Chengying Zhao, et al.
Expert Systems with Applications (2023) Vol. 223, pp. 119886-119886
Closed Access | Times Cited: 22
A hybrid-driven probabilistic state space model for tool wear monitoring
Zhipeng Ma, Ming Zhao, Xuebin Dai, et al.
Mechanical Systems and Signal Processing (2023) Vol. 200, pp. 110599-110599
Closed Access | Times Cited: 21
Zhipeng Ma, Ming Zhao, Xuebin Dai, et al.
Mechanical Systems and Signal Processing (2023) Vol. 200, pp. 110599-110599
Closed Access | Times Cited: 21
Bayesian-based uncertainty-aware tool-wear prediction model in end-milling process of titanium alloy
Gyeongho Kim, Sang Min Yang, Dong Min Kim, et al.
Applied Soft Computing (2023) Vol. 148, pp. 110922-110922
Open Access | Times Cited: 21
Gyeongho Kim, Sang Min Yang, Dong Min Kim, et al.
Applied Soft Computing (2023) Vol. 148, pp. 110922-110922
Open Access | Times Cited: 21
Position-dependent milling process monitoring and surface roughness prediction for complex thin-walled blade component
Zequan Yao, Jingyuan Shen, Ming Wu, et al.
Mechanical Systems and Signal Processing (2023) Vol. 198, pp. 110439-110439
Closed Access | Times Cited: 20
Zequan Yao, Jingyuan Shen, Ming Wu, et al.
Mechanical Systems and Signal Processing (2023) Vol. 198, pp. 110439-110439
Closed Access | Times Cited: 20
A novel deep learning method with partly explainable: Intelligent milling tool wear prediction model based on transformer informed physics
Caihua Hao, Xinyong Mao, Tao Ma, et al.
Advanced Engineering Informatics (2023) Vol. 57, pp. 102106-102106
Closed Access | Times Cited: 20
Caihua Hao, Xinyong Mao, Tao Ma, et al.
Advanced Engineering Informatics (2023) Vol. 57, pp. 102106-102106
Closed Access | Times Cited: 20
Deep learning-based instantaneous cutting force modeling of three-axis CNC milling
Jiejun Xie, Pengcheng Hu, Jihong Chen, et al.
International Journal of Mechanical Sciences (2023) Vol. 246, pp. 108153-108153
Closed Access | Times Cited: 18
Jiejun Xie, Pengcheng Hu, Jihong Chen, et al.
International Journal of Mechanical Sciences (2023) Vol. 246, pp. 108153-108153
Closed Access | Times Cited: 18