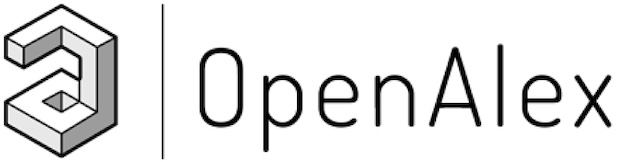
OpenAlex is a bibliographic catalogue of scientific papers, authors and institutions accessible in open access mode, named after the Library of Alexandria. It's citation coverage is excellent and I hope you will find utility in this listing of citing articles!
If you click the article title, you'll navigate to the article, as listed in CrossRef. If you click the Open Access links, you'll navigate to the "best Open Access location". Clicking the citation count will open this listing for that article. Lastly at the bottom of the page, you'll find basic pagination options.
Requested Article:
Optimisation of manufacturing process parameters for variable component geometries using reinforcement learning
Clemens Zimmerling, Christian Poppe, Oliver Stein, et al.
Materials & Design (2022) Vol. 214, pp. 110423-110423
Open Access | Times Cited: 29
Clemens Zimmerling, Christian Poppe, Oliver Stein, et al.
Materials & Design (2022) Vol. 214, pp. 110423-110423
Open Access | Times Cited: 29
Showing 1-25 of 29 citing articles:
Deep reinforcement learning in smart manufacturing: A review and prospects
Chengxi Li, Pai Zheng, Yue Yin, et al.
CIRP journal of manufacturing science and technology (2022) Vol. 40, pp. 75-101
Closed Access | Times Cited: 159
Chengxi Li, Pai Zheng, Yue Yin, et al.
CIRP journal of manufacturing science and technology (2022) Vol. 40, pp. 75-101
Closed Access | Times Cited: 159
Machine learning for polymer composites process simulation – a review
Stefano Cassola, Miro Duhovic, Tim Schmidt, et al.
Composites Part B Engineering (2022) Vol. 246, pp. 110208-110208
Closed Access | Times Cited: 63
Stefano Cassola, Miro Duhovic, Tim Schmidt, et al.
Composites Part B Engineering (2022) Vol. 246, pp. 110208-110208
Closed Access | Times Cited: 63
Machine Learning in Manufacturing towards Industry 4.0: From ‘For Now’ to ‘Four-Know’
Tingting Chen, Vignesh Sampath, Marvin Carl May, et al.
Applied Sciences (2023) Vol. 13, Iss. 3, pp. 1903-1903
Open Access | Times Cited: 37
Tingting Chen, Vignesh Sampath, Marvin Carl May, et al.
Applied Sciences (2023) Vol. 13, Iss. 3, pp. 1903-1903
Open Access | Times Cited: 37
A reinforcement learning approach for process parameter optimization in additive manufacturing
Susheel Dharmadhikari, Nandana Menon, Amrita Basak
Additive manufacturing (2023) Vol. 71, pp. 103556-103556
Open Access | Times Cited: 34
Susheel Dharmadhikari, Nandana Menon, Amrita Basak
Additive manufacturing (2023) Vol. 71, pp. 103556-103556
Open Access | Times Cited: 34
Rapidly predicting the effect of tool geometry on the wrinkling of biaxial NCFs during composites manufacturing using a deep learning surrogate model
J.V. Viisainen, F. Richard Yu, A. Codolini, et al.
Composites Part B Engineering (2023) Vol. 253, pp. 110536-110536
Open Access | Times Cited: 21
J.V. Viisainen, F. Richard Yu, A. Codolini, et al.
Composites Part B Engineering (2023) Vol. 253, pp. 110536-110536
Open Access | Times Cited: 21
Physics-informed neural networks for data-free surrogate modelling and engineering optimization – An example from composite manufacturing
Tobias Würth, Constantin Krauß, Clemens Zimmerling, et al.
Materials & Design (2023) Vol. 231, pp. 112034-112034
Open Access | Times Cited: 20
Tobias Würth, Constantin Krauß, Clemens Zimmerling, et al.
Materials & Design (2023) Vol. 231, pp. 112034-112034
Open Access | Times Cited: 20
A Review of Machine Learning for Progressive Damage Modelling of Fiber-Reinforced Composites
Jimbay Loh, Kirk Ming Yeoh, Karthikayen Raju, et al.
Applied Composite Materials (2024)
Closed Access | Times Cited: 7
Jimbay Loh, Kirk Ming Yeoh, Karthikayen Raju, et al.
Applied Composite Materials (2024)
Closed Access | Times Cited: 7
Fast optimisation of the formability of dry fabric preforms: A Bayesian approach
Siyuan Chen, Adam J. Thompson, Tim Dodwell, et al.
Materials & Design (2023) Vol. 230, pp. 111986-111986
Open Access | Times Cited: 15
Siyuan Chen, Adam J. Thompson, Tim Dodwell, et al.
Materials & Design (2023) Vol. 230, pp. 111986-111986
Open Access | Times Cited: 15
Process models: A cornerstone to composites 4.0
Jonathan P.-H. Belnoue, Stephen R. Hallett
Composites Part B Engineering (2024) Vol. 283, pp. 111621-111621
Open Access | Times Cited: 4
Jonathan P.-H. Belnoue, Stephen R. Hallett
Composites Part B Engineering (2024) Vol. 283, pp. 111621-111621
Open Access | Times Cited: 4
Parametric study on the effect of material properties, tool geometry, and tolerances on preform quality in wind turbine blade manufacturing
Peter H. Broberg, Esben Lindgaard, Adam J. Thompson, et al.
Composite Structures (2024) Vol. 344, pp. 118324-118324
Open Access | Times Cited: 4
Peter H. Broberg, Esben Lindgaard, Adam J. Thompson, et al.
Composite Structures (2024) Vol. 344, pp. 118324-118324
Open Access | Times Cited: 4
Machine Learning-Based Modeling for Structural Engineering: A Comprehensive Survey and Applications Overview
Bassey B. Etim, Alia Al-Ghosoun, Jamil Renno, et al.
Buildings (2024) Vol. 14, Iss. 11, pp. 3515-3515
Open Access | Times Cited: 4
Bassey B. Etim, Alia Al-Ghosoun, Jamil Renno, et al.
Buildings (2024) Vol. 14, Iss. 11, pp. 3515-3515
Open Access | Times Cited: 4
Advancements of machine learning techniques in fiber-filled polymer composites: a review
R. Alagulakshmi, R. Ramalakshmi, V. Arumugaprabu, et al.
Polymer Bulletin (2025)
Closed Access
R. Alagulakshmi, R. Ramalakshmi, V. Arumugaprabu, et al.
Polymer Bulletin (2025)
Closed Access
A comparison between robust design and digital twin approaches for Non-Crimp fabric (NCF) forming
Siyuan Chen, Adam J. Thompson, Tim Dodwell, et al.
Composites Part A Applied Science and Manufacturing (2025), pp. 108864-108864
Open Access
Siyuan Chen, Adam J. Thompson, Tim Dodwell, et al.
Composites Part A Applied Science and Manufacturing (2025), pp. 108864-108864
Open Access
Preforming characteristics and defect mitigation strategies for multi-layered biaxial pillar-stitched non-crimp fabric
Ming Mei, Yujia He, Kai Wei, et al.
International Journal of Solids and Structures (2023) Vol. 267, pp. 112150-112150
Closed Access | Times Cited: 9
Ming Mei, Yujia He, Kai Wei, et al.
International Journal of Solids and Structures (2023) Vol. 267, pp. 112150-112150
Closed Access | Times Cited: 9
Predicting the effect of voids generated during RTM on the low-velocity impact behaviour by machine learning-based surrogate models
Julen Mendikute, M. Baskaran, Iñigo Llavori, et al.
Composites Part B Engineering (2023) Vol. 260, pp. 110790-110790
Closed Access | Times Cited: 9
Julen Mendikute, M. Baskaran, Iñigo Llavori, et al.
Composites Part B Engineering (2023) Vol. 260, pp. 110790-110790
Closed Access | Times Cited: 9
A Review of the Intelligent Optimization and Decision in Plastic Forming
Xuefeng Tang, Zhizhou Wang, Lei Deng, et al.
Materials (2022) Vol. 15, Iss. 19, pp. 7019-7019
Open Access | Times Cited: 14
Xuefeng Tang, Zhizhou Wang, Lei Deng, et al.
Materials (2022) Vol. 15, Iss. 19, pp. 7019-7019
Open Access | Times Cited: 14
Additive manufacturing process parameter design for variable component geometries using reinforcement learning
Ehsan Vaghefi, Seyedmehrab Hosseini, Amir Hossein Afsharinejad, et al.
Additive manufacturing (2024) Vol. 84, pp. 104121-104121
Closed Access | Times Cited: 2
Ehsan Vaghefi, Seyedmehrab Hosseini, Amir Hossein Afsharinejad, et al.
Additive manufacturing (2024) Vol. 84, pp. 104121-104121
Closed Access | Times Cited: 2
Machine learning in polymer additive manufacturing: a review
Mohammad Hossein Nikooharf, Mohammadali Shirinbayan, Mahsa Arabkoohi, et al.
International Journal of Material Forming (2024) Vol. 17, Iss. 6
Open Access | Times Cited: 2
Mohammad Hossein Nikooharf, Mohammadali Shirinbayan, Mahsa Arabkoohi, et al.
International Journal of Material Forming (2024) Vol. 17, Iss. 6
Open Access | Times Cited: 2
A novel heuristic approach to detect induced forming defects using point cloud scans
Muhammad Shahrukh Saeed, Sheharyar Faisal, Boris Eisenbart, et al.
Proceedings of the Design Society (2024) Vol. 4, pp. 723-734
Open Access | Times Cited: 1
Muhammad Shahrukh Saeed, Sheharyar Faisal, Boris Eisenbart, et al.
Proceedings of the Design Society (2024) Vol. 4, pp. 723-734
Open Access | Times Cited: 1
Physics-informed MeshGraphNets (PI-MGNs): Neural finite element solvers for non-stationary and nonlinear simulations on arbitrary meshes
Tobias Würth, Niklas Freymuth, Clemens Zimmerling, et al.
Computer Methods in Applied Mechanics and Engineering (2024) Vol. 429, pp. 117102-117102
Open Access | Times Cited: 1
Tobias Würth, Niklas Freymuth, Clemens Zimmerling, et al.
Computer Methods in Applied Mechanics and Engineering (2024) Vol. 429, pp. 117102-117102
Open Access | Times Cited: 1
Promoting Synergies to Improve Manufacturing Efficiency in Industrial Material Processing: A Systematic Review of Industry 4.0 and AI
Md. Sazol Ahmmed, Sriram Praneeth Isanaka, Frank Liou
Machines (2024) Vol. 12, Iss. 10, pp. 681-681
Open Access | Times Cited: 1
Md. Sazol Ahmmed, Sriram Praneeth Isanaka, Frank Liou
Machines (2024) Vol. 12, Iss. 10, pp. 681-681
Open Access | Times Cited: 1
Machining parameter optimization for a batch milling system using multi-task deep reinforcement learning
Pei Wang, Yixin Cui, Haizhen Tao, et al.
Journal of Manufacturing Systems (2024) Vol. 78, pp. 124-152
Open Access | Times Cited: 1
Pei Wang, Yixin Cui, Haizhen Tao, et al.
Journal of Manufacturing Systems (2024) Vol. 78, pp. 124-152
Open Access | Times Cited: 1
Predictive Simulation of the Creation of Wrinkles during Preform Manufacturing
Peter H. Broberg
(2024)
Open Access
Peter H. Broberg
(2024)
Open Access
Kri2aw: A Process Industry Causal Discovery Method with Domain Knowledge Utilization and Residual Reconstruction Via Independent Adversarial and Adaptive Weighting
Yiquan An, Zhimin Lv, Xi Sun, et al.
(2024)
Closed Access
Yiquan An, Zhimin Lv, Xi Sun, et al.
(2024)
Closed Access
Combining simulation methods and machine learning for efficient forming process development
Clemens Zimmerling, Luise Kärger
Elsevier eBooks (2024), pp. 577-605
Closed Access
Clemens Zimmerling, Luise Kärger
Elsevier eBooks (2024), pp. 577-605
Closed Access