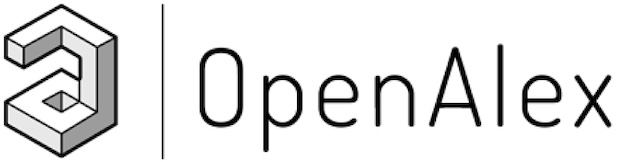
OpenAlex is a bibliographic catalogue of scientific papers, authors and institutions accessible in open access mode, named after the Library of Alexandria. It's citation coverage is excellent and I hope you will find utility in this listing of citing articles!
If you click the article title, you'll navigate to the article, as listed in CrossRef. If you click the Open Access links, you'll navigate to the "best Open Access location". Clicking the citation count will open this listing for that article. Lastly at the bottom of the page, you'll find basic pagination options.
Requested Article:
Ensemble machine learning model for corrosion initiation time estimation of embedded steel reinforced self-compacting concrete
Babatunde Abiodun Salami, Syed Masiur Rahman, Tajudeen A. Oyehan, et al.
Measurement (2020) Vol. 165, pp. 108141-108141
Closed Access | Times Cited: 87
Babatunde Abiodun Salami, Syed Masiur Rahman, Tajudeen A. Oyehan, et al.
Measurement (2020) Vol. 165, pp. 108141-108141
Closed Access | Times Cited: 87
Showing 1-25 of 87 citing articles:
Prediction of Compressive Strength of Fly Ash Based Concrete Using Individual and Ensemble Algorithm
Ayaz Ahmad, Furqan Farooq, Paweł Niewiadomski, et al.
Materials (2021) Vol. 14, Iss. 4, pp. 794-794
Open Access | Times Cited: 206
Ayaz Ahmad, Furqan Farooq, Paweł Niewiadomski, et al.
Materials (2021) Vol. 14, Iss. 4, pp. 794-794
Open Access | Times Cited: 206
Artificial Intelligence, Machine Learning, and Deep Learning in Structural Engineering: A Scientometrics Review of Trends and Best Practices
Arash Teymori Gharah Tapeh, M.Z. Naser
Archives of Computational Methods in Engineering (2022) Vol. 30, Iss. 1, pp. 115-159
Closed Access | Times Cited: 170
Arash Teymori Gharah Tapeh, M.Z. Naser
Archives of Computational Methods in Engineering (2022) Vol. 30, Iss. 1, pp. 115-159
Closed Access | Times Cited: 170
Reviewing machine learning of corrosion prediction in a data-oriented perspective
Leonardo Bertolucci Coelho, Dawei Zhang, Yves Van Ingelgem, et al.
npj Materials Degradation (2022) Vol. 6, Iss. 1
Open Access | Times Cited: 147
Leonardo Bertolucci Coelho, Dawei Zhang, Yves Van Ingelgem, et al.
npj Materials Degradation (2022) Vol. 6, Iss. 1
Open Access | Times Cited: 147
Estimating compressive strength of lightweight foamed concrete using neural, genetic and ensemble machine learning approaches
Babatunde Abiodun Salami, Mudassir Iqbal, Abdulazeez Abdulraheem, et al.
Cement and Concrete Composites (2022) Vol. 133, pp. 104721-104721
Open Access | Times Cited: 94
Babatunde Abiodun Salami, Mudassir Iqbal, Abdulazeez Abdulraheem, et al.
Cement and Concrete Composites (2022) Vol. 133, pp. 104721-104721
Open Access | Times Cited: 94
Compressive Strength Evaluation of Ultra-High-Strength Concrete by Machine Learning
Zhongjie Shen, Ahmed Farouk Deifalla, Paweł Kamiński, et al.
Materials (2022) Vol. 15, Iss. 10, pp. 3523-3523
Open Access | Times Cited: 76
Zhongjie Shen, Ahmed Farouk Deifalla, Paweł Kamiński, et al.
Materials (2022) Vol. 15, Iss. 10, pp. 3523-3523
Open Access | Times Cited: 76
Evolution of corrosion prediction models for oil and gas pipelines: From empirical-driven to data-driven
Qinying Wang, Yuhui Song, Xingshou Zhang, et al.
Engineering Failure Analysis (2023) Vol. 146, pp. 107097-107097
Closed Access | Times Cited: 67
Qinying Wang, Yuhui Song, Xingshou Zhang, et al.
Engineering Failure Analysis (2023) Vol. 146, pp. 107097-107097
Closed Access | Times Cited: 67
Study on the deterioration of concrete performance in saline soil area under the combined effect of high low temperatures, chloride and sulfate salts
Daming Luo, Fan Li, Ditao Niu
Cement and Concrete Composites (2024) Vol. 150, pp. 105531-105531
Closed Access | Times Cited: 36
Daming Luo, Fan Li, Ditao Niu
Cement and Concrete Composites (2024) Vol. 150, pp. 105531-105531
Closed Access | Times Cited: 36
Computational experiments of metal corrosion studies: A review
Shuhao Li, Chunqing Li, Feng Wang
Materials Today Chemistry (2024) Vol. 37, pp. 101986-101986
Open Access | Times Cited: 31
Shuhao Li, Chunqing Li, Feng Wang
Materials Today Chemistry (2024) Vol. 37, pp. 101986-101986
Open Access | Times Cited: 31
A critical review of machine learning algorithms in maritime, offshore, and oil & gas corrosion research: A comprehensive analysis of ANN and RF models
Md Mahadi Hasan Imran, Shahrizan Jamaludin, Ahmad Faisal Mohamad Ayob
Ocean Engineering (2024) Vol. 295, pp. 116796-116796
Closed Access | Times Cited: 24
Md Mahadi Hasan Imran, Shahrizan Jamaludin, Ahmad Faisal Mohamad Ayob
Ocean Engineering (2024) Vol. 295, pp. 116796-116796
Closed Access | Times Cited: 24
Probabilistic models applied to concrete corrosion depth prediction under sulfuric acid environment
Jie Xiao, Linhai Huang, Zhaoxiang He, et al.
Measurement (2024) Vol. 234, pp. 114807-114807
Closed Access | Times Cited: 21
Jie Xiao, Linhai Huang, Zhaoxiang He, et al.
Measurement (2024) Vol. 234, pp. 114807-114807
Closed Access | Times Cited: 21
Data-driven model for ternary-blend concrete compressive strength prediction using machine learning approach
Babatunde Abiodun Salami, Teslim Olayiwola, Tajudeen A. Oyehan, et al.
Construction and Building Materials (2021) Vol. 301, pp. 124152-124152
Closed Access | Times Cited: 70
Babatunde Abiodun Salami, Teslim Olayiwola, Tajudeen A. Oyehan, et al.
Construction and Building Materials (2021) Vol. 301, pp. 124152-124152
Closed Access | Times Cited: 70
Prediction of the sulfate resistance for recycled aggregate concrete based on ensemble learning algorithms
Kaihua Liu, Zihang Dai, Rongbin Zhang, et al.
Construction and Building Materials (2021) Vol. 317, pp. 125917-125917
Closed Access | Times Cited: 64
Kaihua Liu, Zihang Dai, Rongbin Zhang, et al.
Construction and Building Materials (2021) Vol. 317, pp. 125917-125917
Closed Access | Times Cited: 64
Machine learning modeling of time-dependent corrosion rates of carbon steel in presence of corrosion inhibitors
Mohammadreza Aghaaminiha, Ramin Mehrani, Martin Colahan, et al.
Corrosion Science (2021) Vol. 193, pp. 109904-109904
Closed Access | Times Cited: 58
Mohammadreza Aghaaminiha, Ramin Mehrani, Martin Colahan, et al.
Corrosion Science (2021) Vol. 193, pp. 109904-109904
Closed Access | Times Cited: 58
Beyond the chloride threshold concept for predicting corrosion of steel in concrete
Ueli Angst, O. Burkan Isgor, C.M. Hansson, et al.
Applied Physics Reviews (2022) Vol. 9, Iss. 1
Open Access | Times Cited: 42
Ueli Angst, O. Burkan Isgor, C.M. Hansson, et al.
Applied Physics Reviews (2022) Vol. 9, Iss. 1
Open Access | Times Cited: 42
Intelligent optimization for modelling superhydrophobic ceramic membrane oil flux and oil-water separation efficiency: Evidence from wastewater treatment and experimental laboratory
Jamilu Usman, Babatunde Abiodun Salami, Afeez Gbadamosi, et al.
Chemosphere (2023) Vol. 331, pp. 138726-138726
Closed Access | Times Cited: 34
Jamilu Usman, Babatunde Abiodun Salami, Afeez Gbadamosi, et al.
Chemosphere (2023) Vol. 331, pp. 138726-138726
Closed Access | Times Cited: 34
Modeling the emission characteristics of the hydrogen-enriched natural gas engines by multi-output least-squares support vector regression: Comprehensive statistical and operating analyses
Tao Hai, Dler Hussein Kadir, Afshin Ghanbari
Energy (2023) Vol. 276, pp. 127515-127515
Closed Access | Times Cited: 32
Tao Hai, Dler Hussein Kadir, Afshin Ghanbari
Energy (2023) Vol. 276, pp. 127515-127515
Closed Access | Times Cited: 32
New-generation machine learning models as prediction tools for modeling interfacial tension of hydrogen-brine system
Afeez Gbadamosi, Haruna Adamu, Jamilu Usman, et al.
International Journal of Hydrogen Energy (2023) Vol. 50, pp. 1326-1337
Closed Access | Times Cited: 29
Afeez Gbadamosi, Haruna Adamu, Jamilu Usman, et al.
International Journal of Hydrogen Energy (2023) Vol. 50, pp. 1326-1337
Closed Access | Times Cited: 29
Machine learning-based acoustic emission technique for corrosion-induced damage monitoring in reinforced concrete structures
A. Thirumalaiselvi, Saptarshi Sasmal
Engineering Applications of Artificial Intelligence (2024) Vol. 137, pp. 109121-109121
Closed Access | Times Cited: 11
A. Thirumalaiselvi, Saptarshi Sasmal
Engineering Applications of Artificial Intelligence (2024) Vol. 137, pp. 109121-109121
Closed Access | Times Cited: 11
Machine Learning as an Innovative Engineering Tool for Controlling Concrete Performance: A Comprehensive Review
Fatemeh Mobasheri, Masoud Hosseinpoor, Ammar Yahia, et al.
Archives of Computational Methods in Engineering (2025)
Closed Access | Times Cited: 1
Fatemeh Mobasheri, Masoud Hosseinpoor, Ammar Yahia, et al.
Archives of Computational Methods in Engineering (2025)
Closed Access | Times Cited: 1
Research on the critical chloride content of reinforcement corrosion in marine concrete — A review
Maohua Zhang, Shanghui Sun, Ke Liu, et al.
Journal of Building Engineering (2023) Vol. 79, pp. 107838-107838
Closed Access | Times Cited: 23
Maohua Zhang, Shanghui Sun, Ke Liu, et al.
Journal of Building Engineering (2023) Vol. 79, pp. 107838-107838
Closed Access | Times Cited: 23
Interpretable machine learning for maximum corrosion depth and influence factor analysis
Yuhui Song, Qinying Wang, Xingshou Zhang, et al.
npj Materials Degradation (2023) Vol. 7, Iss. 1
Open Access | Times Cited: 20
Yuhui Song, Qinying Wang, Xingshou Zhang, et al.
npj Materials Degradation (2023) Vol. 7, Iss. 1
Open Access | Times Cited: 20
Recovery of Brine Resources Through Crown-Passivated Graphene, Silicene, and Boron Nitride Nanosheets Based on Machine-Learning Structural Predictions
Ismail Abdulazeez, Sani I. Abba, Jamilu Usman, et al.
ACS Applied Nano Materials (2023) Vol. 6, Iss. 24, pp. 23207-23221
Closed Access | Times Cited: 17
Ismail Abdulazeez, Sani I. Abba, Jamilu Usman, et al.
ACS Applied Nano Materials (2023) Vol. 6, Iss. 24, pp. 23207-23221
Closed Access | Times Cited: 17
Novel base predictive model of resilient modulus of compacted subgrade soils by using interpretable approaches with graphical user interface
Loai Alkhattabi, Kiran Arif
Materials Today Communications (2024) Vol. 40, pp. 109764-109764
Closed Access | Times Cited: 8
Loai Alkhattabi, Kiran Arif
Materials Today Communications (2024) Vol. 40, pp. 109764-109764
Closed Access | Times Cited: 8
Analysis, Assessment, and Mitigation of Stress Corrosion Cracking in Austenitic Stainless Steels in the Oil and Gas Sector: A Review
Mohammadtaghi Vakili, Petr Koutnı́k, Jan Kohout, et al.
Surfaces (2024) Vol. 7, Iss. 3, pp. 589-642
Open Access | Times Cited: 8
Mohammadtaghi Vakili, Petr Koutnı́k, Jan Kohout, et al.
Surfaces (2024) Vol. 7, Iss. 3, pp. 589-642
Open Access | Times Cited: 8
Effect of Alumina Additives on Mechanical and Fresh Properties of Self-Compacting Concrete: A Review
Hoofar Shokravi, Seyed Esmaeil Mohammadyan-Yasouj, Seyed Saeid Rahimian Koloor, et al.
Processes (2021) Vol. 9, Iss. 3, pp. 554-554
Open Access | Times Cited: 41
Hoofar Shokravi, Seyed Esmaeil Mohammadyan-Yasouj, Seyed Saeid Rahimian Koloor, et al.
Processes (2021) Vol. 9, Iss. 3, pp. 554-554
Open Access | Times Cited: 41