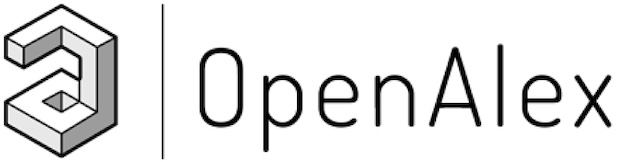
OpenAlex is a bibliographic catalogue of scientific papers, authors and institutions accessible in open access mode, named after the Library of Alexandria. It's citation coverage is excellent and I hope you will find utility in this listing of citing articles!
If you click the article title, you'll navigate to the article, as listed in CrossRef. If you click the Open Access links, you'll navigate to the "best Open Access location". Clicking the citation count will open this listing for that article. Lastly at the bottom of the page, you'll find basic pagination options.
Requested Article:
Effective wind power prediction using novel deep learning network: Stacked independently recurrent autoencoder
Lin Wang, Rui Tao, Huanling Hu, et al.
Renewable Energy (2020) Vol. 164, pp. 642-655
Closed Access | Times Cited: 79
Lin Wang, Rui Tao, Huanling Hu, et al.
Renewable Energy (2020) Vol. 164, pp. 642-655
Closed Access | Times Cited: 79
Showing 1-25 of 79 citing articles:
A review and taxonomy of wind and solar energy forecasting methods based on deep learning
Ghadah Alkhayat, Rashid Mehmood
Energy and AI (2021) Vol. 4, pp. 100060-100060
Open Access | Times Cited: 205
Ghadah Alkhayat, Rashid Mehmood
Energy and AI (2021) Vol. 4, pp. 100060-100060
Open Access | Times Cited: 205
Wind, Solar, and Photovoltaic Renewable Energy Systems with and without Energy Storage Optimization: A Survey of Advanced Machine Learning and Deep Learning Techniques
Laith Abualigah, Raed Abu Zitar, Khaled H. Almotairi, et al.
Energies (2022) Vol. 15, Iss. 2, pp. 578-578
Open Access | Times Cited: 115
Laith Abualigah, Raed Abu Zitar, Khaled H. Almotairi, et al.
Energies (2022) Vol. 15, Iss. 2, pp. 578-578
Open Access | Times Cited: 115
Adaptive VMD based optimized deep learning mixed kernel ELM autoencoder for single and multistep wind power forecasting
Vijaya Krishna Rayi, Sthita Prajna Mishra, Jyotirmayee Naik, et al.
Energy (2021) Vol. 244, pp. 122585-122585
Closed Access | Times Cited: 105
Vijaya Krishna Rayi, Sthita Prajna Mishra, Jyotirmayee Naik, et al.
Energy (2021) Vol. 244, pp. 122585-122585
Closed Access | Times Cited: 105
Deep learning for renewable energy forecasting: A taxonomy, and systematic literature review
Changtian Ying, Weiqing Wang, Jiong Yu, et al.
Journal of Cleaner Production (2022) Vol. 384, pp. 135414-135414
Closed Access | Times Cited: 75
Changtian Ying, Weiqing Wang, Jiong Yu, et al.
Journal of Cleaner Production (2022) Vol. 384, pp. 135414-135414
Closed Access | Times Cited: 75
A wavelet-LSTM model for short-term wind power forecasting using wind farm SCADA data
Zhaohua Liu, Chang-Tong Wang, Hua‐Liang Wei, et al.
Expert Systems with Applications (2024) Vol. 247, pp. 123237-123237
Closed Access | Times Cited: 18
Zhaohua Liu, Chang-Tong Wang, Hua‐Liang Wei, et al.
Expert Systems with Applications (2024) Vol. 247, pp. 123237-123237
Closed Access | Times Cited: 18
Meta-heuristics and deep learning for energy applications: Review and open research challenges (2018–2023)
Eghbal Hosseini, Abbas M. Al-Ghaili, Dler Hussein Kadir, et al.
Energy Strategy Reviews (2024) Vol. 53, pp. 101409-101409
Open Access | Times Cited: 17
Eghbal Hosseini, Abbas M. Al-Ghaili, Dler Hussein Kadir, et al.
Energy Strategy Reviews (2024) Vol. 53, pp. 101409-101409
Open Access | Times Cited: 17
A simple approach for short-term wind speed interval prediction based on independently recurrent neural networks and error probability distribution
Adnan Saeed, Chaoshun Li, Zhenhao Gan, et al.
Energy (2021) Vol. 238, pp. 122012-122012
Closed Access | Times Cited: 62
Adnan Saeed, Chaoshun Li, Zhenhao Gan, et al.
Energy (2021) Vol. 238, pp. 122012-122012
Closed Access | Times Cited: 62
Natural phase space reconstruction-based broad learning system for short-term wind speed prediction: Case studies of an offshore wind farm
Xuefang Xu, Shiting Hu, Peiming Shi, et al.
Energy (2022) Vol. 262, pp. 125342-125342
Closed Access | Times Cited: 58
Xuefang Xu, Shiting Hu, Peiming Shi, et al.
Energy (2022) Vol. 262, pp. 125342-125342
Closed Access | Times Cited: 58
A novel wind power prediction approach using multivariate variational mode decomposition and multi-objective crisscross optimization based deep extreme learning machine
Anbo Meng, Zibin Zhu, Weisi Deng, et al.
Energy (2022) Vol. 260, pp. 124957-124957
Closed Access | Times Cited: 45
Anbo Meng, Zibin Zhu, Weisi Deng, et al.
Energy (2022) Vol. 260, pp. 124957-124957
Closed Access | Times Cited: 45
A novel combined forecasting model based on neural networks, deep learning approaches, and multi-objective optimization for short-term wind speed forecasting
Jianzhou Wang, Yining An, Zhiwu Li, et al.
Energy (2022) Vol. 251, pp. 123960-123960
Closed Access | Times Cited: 42
Jianzhou Wang, Yining An, Zhiwu Li, et al.
Energy (2022) Vol. 251, pp. 123960-123960
Closed Access | Times Cited: 42
An overview of deterministic and probabilistic forecasting methods of wind energy
Yuying Xie, Chaoshun Li, Mengying Li, et al.
iScience (2022) Vol. 26, Iss. 1, pp. 105804-105804
Open Access | Times Cited: 42
Yuying Xie, Chaoshun Li, Mengying Li, et al.
iScience (2022) Vol. 26, Iss. 1, pp. 105804-105804
Open Access | Times Cited: 42
A Review on Sustainable Energy Sources Using Machine Learning and Deep Learning Models
Ashok Bhansali, Namala Narasimhulu, Rocío Pérez de Prado, et al.
Energies (2023) Vol. 16, Iss. 17, pp. 6236-6236
Open Access | Times Cited: 31
Ashok Bhansali, Namala Narasimhulu, Rocío Pérez de Prado, et al.
Energies (2023) Vol. 16, Iss. 17, pp. 6236-6236
Open Access | Times Cited: 31
Two-stage decomposition and temporal fusion transformers for interpretable wind speed forecasting
Binrong Wu, Lin Wang
Energy (2023) Vol. 288, pp. 129728-129728
Closed Access | Times Cited: 26
Binrong Wu, Lin Wang
Energy (2023) Vol. 288, pp. 129728-129728
Closed Access | Times Cited: 26
A novel temporal–spatial graph neural network for wind power forecasting considering blockage effects
Hong Qiu, Kaikai Shi, Renfang Wang, et al.
Renewable Energy (2024) Vol. 227, pp. 120499-120499
Closed Access | Times Cited: 13
Hong Qiu, Kaikai Shi, Renfang Wang, et al.
Renewable Energy (2024) Vol. 227, pp. 120499-120499
Closed Access | Times Cited: 13
A GCN-based adaptive generative adversarial network model for short-term wind speed scenario prediction
Xin Liu, Jingjia Yu, Lin Gong, et al.
Energy (2024) Vol. 294, pp. 130931-130931
Closed Access | Times Cited: 8
Xin Liu, Jingjia Yu, Lin Gong, et al.
Energy (2024) Vol. 294, pp. 130931-130931
Closed Access | Times Cited: 8
An adaptive distribution-matched recurrent network for wind power prediction using time-series distribution period division
Anbo Meng, Haitao Zhang, Zhongfu Dai, et al.
Energy (2024) Vol. 299, pp. 131383-131383
Closed Access | Times Cited: 8
Anbo Meng, Haitao Zhang, Zhongfu Dai, et al.
Energy (2024) Vol. 299, pp. 131383-131383
Closed Access | Times Cited: 8
New Hybrid Deep Neural Architectural Search-Based Ensemble Reinforcement Learning Strategy for Wind Power Forecasting
Seyed Mohammad Jafar Jalali, Gerardo J. Osório, Sajad Ahmadian, et al.
IEEE Transactions on Industry Applications (2021) Vol. 58, Iss. 1, pp. 15-27
Closed Access | Times Cited: 51
Seyed Mohammad Jafar Jalali, Gerardo J. Osório, Sajad Ahmadian, et al.
IEEE Transactions on Industry Applications (2021) Vol. 58, Iss. 1, pp. 15-27
Closed Access | Times Cited: 51
A novel few-shot learning approach for wind power prediction applying secondary evolutionary generative adversarial network
Anbo Meng, Shu Chen, Zuhong Ou, et al.
Energy (2022) Vol. 261, pp. 125276-125276
Closed Access | Times Cited: 35
Anbo Meng, Shu Chen, Zuhong Ou, et al.
Energy (2022) Vol. 261, pp. 125276-125276
Closed Access | Times Cited: 35
Ultra-short-term wind power prediction method based on FTI-VACA-XGB model
Shijie Guan, Yongsheng Wang, Limin Liu, et al.
Expert Systems with Applications (2023) Vol. 235, pp. 121185-121185
Open Access | Times Cited: 20
Shijie Guan, Yongsheng Wang, Limin Liu, et al.
Expert Systems with Applications (2023) Vol. 235, pp. 121185-121185
Open Access | Times Cited: 20
Day-ahead electricity price forecasting employing a novel hybrid frame of deep learning methods: A case study in NSW, Australia
Yong Qiang Tan, Yan Shen, Xin Yu, et al.
Electric Power Systems Research (2023) Vol. 220, pp. 109300-109300
Closed Access | Times Cited: 19
Yong Qiang Tan, Yan Shen, Xin Yu, et al.
Electric Power Systems Research (2023) Vol. 220, pp. 109300-109300
Closed Access | Times Cited: 19
Half-hourly electricity price prediction with a hybrid convolution neural network-random vector functional link deep learning approach
Sujan Ghimire, Ravinesh C. Deo, David Casillas-Pérez, et al.
Applied Energy (2024) Vol. 374, pp. 123920-123920
Open Access | Times Cited: 7
Sujan Ghimire, Ravinesh C. Deo, David Casillas-Pérez, et al.
Applied Energy (2024) Vol. 374, pp. 123920-123920
Open Access | Times Cited: 7
Review of several key processes in wind power forecasting: Mathematical formulations, scientific problems, and logical relations
Mao Yang, Y. Huang, Chuanyu Xu, et al.
Applied Energy (2024) Vol. 377, pp. 124631-124631
Closed Access | Times Cited: 7
Mao Yang, Y. Huang, Chuanyu Xu, et al.
Applied Energy (2024) Vol. 377, pp. 124631-124631
Closed Access | Times Cited: 7
Leveraging Deep Learning to Strengthen the Cyber-Resilience of Renewable Energy Supply Chains: A Survey
Malka N. Halgamuge
IEEE Communications Surveys & Tutorials (2024) Vol. 26, Iss. 3, pp. 2146-2175
Closed Access | Times Cited: 6
Malka N. Halgamuge
IEEE Communications Surveys & Tutorials (2024) Vol. 26, Iss. 3, pp. 2146-2175
Closed Access | Times Cited: 6
Development and trending of deep learning methods for wind power predictions
Hong Liu, Zijun Zhang
Artificial Intelligence Review (2024) Vol. 57, Iss. 5
Open Access | Times Cited: 6
Hong Liu, Zijun Zhang
Artificial Intelligence Review (2024) Vol. 57, Iss. 5
Open Access | Times Cited: 6
Short-term wind power prediction method based on multivariate signal decomposition and RIME optimization algorithm
Y. Wang, Lili Pei, Wei Li, et al.
Expert Systems with Applications (2024) Vol. 259, pp. 125376-125376
Closed Access | Times Cited: 6
Y. Wang, Lili Pei, Wei Li, et al.
Expert Systems with Applications (2024) Vol. 259, pp. 125376-125376
Closed Access | Times Cited: 6