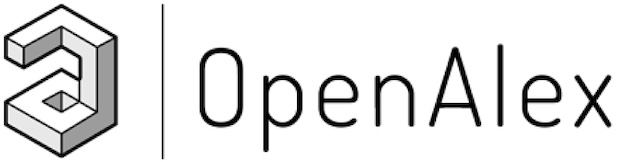
OpenAlex is a bibliographic catalogue of scientific papers, authors and institutions accessible in open access mode, named after the Library of Alexandria. It's citation coverage is excellent and I hope you will find utility in this listing of citing articles!
If you click the article title, you'll navigate to the article, as listed in CrossRef. If you click the Open Access links, you'll navigate to the "best Open Access location". Clicking the citation count will open this listing for that article. Lastly at the bottom of the page, you'll find basic pagination options.
Requested Article:
Machine learned coarse-grained protein force-fields: Are we there yet?
Aleksander E. P. Durumeric, Nicholas E. Charron, Clark Templeton, et al.
Current Opinion in Structural Biology (2023) Vol. 79, pp. 102533-102533
Open Access | Times Cited: 53
Aleksander E. P. Durumeric, Nicholas E. Charron, Clark Templeton, et al.
Current Opinion in Structural Biology (2023) Vol. 79, pp. 102533-102533
Open Access | Times Cited: 53
Showing 1-25 of 53 citing articles:
Machine Learning-Guided Protein Engineering
Petr Kouba, Pavel Kohout, Faraneh Haddadi, et al.
ACS Catalysis (2023) Vol. 13, Iss. 21, pp. 13863-13895
Open Access | Times Cited: 90
Petr Kouba, Pavel Kohout, Faraneh Haddadi, et al.
ACS Catalysis (2023) Vol. 13, Iss. 21, pp. 13863-13895
Open Access | Times Cited: 90
Perspective: Advances, Challenges, and Insight for Predictive Coarse-Grained Models
W. G. Noid
The Journal of Physical Chemistry B (2023) Vol. 127, Iss. 19, pp. 4174-4207
Open Access | Times Cited: 78
W. G. Noid
The Journal of Physical Chemistry B (2023) Vol. 127, Iss. 19, pp. 4174-4207
Open Access | Times Cited: 78
Progress at protein structure prediction, as seen in CASP15
Arne Elofsson
Current Opinion in Structural Biology (2023) Vol. 80, pp. 102594-102594
Open Access | Times Cited: 69
Arne Elofsson
Current Opinion in Structural Biology (2023) Vol. 80, pp. 102594-102594
Open Access | Times Cited: 69
Machine learning coarse-grained potentials of protein thermodynamics
Maciej Majewski, Adrià Pérez, Philipp Thölke, et al.
Nature Communications (2023) Vol. 14, Iss. 1
Open Access | Times Cited: 56
Maciej Majewski, Adrià Pérez, Philipp Thölke, et al.
Nature Communications (2023) Vol. 14, Iss. 1
Open Access | Times Cited: 56
Pragmatic Coarse-Graining of Proteins: Models and Applications
Luís Borges-Araújo, Ilias Patmanidis, Akhil Pratap Singh, et al.
Journal of Chemical Theory and Computation (2023) Vol. 19, Iss. 20, pp. 7112-7135
Open Access | Times Cited: 48
Luís Borges-Araújo, Ilias Patmanidis, Akhil Pratap Singh, et al.
Journal of Chemical Theory and Computation (2023) Vol. 19, Iss. 20, pp. 7112-7135
Open Access | Times Cited: 48
Crash testing machine learning force fields for molecules, materials, and interfaces: model analysis in the TEA Challenge 2023
Igor Poltavsky, Anton Charkin-Gorbulin, Mirela Puleva, et al.
Chemical Science (2025)
Open Access | Times Cited: 2
Igor Poltavsky, Anton Charkin-Gorbulin, Mirela Puleva, et al.
Chemical Science (2025)
Open Access | Times Cited: 2
The Potential of Neural Network Potentials
Timothy T. Duignan
ACS Physical Chemistry Au (2024) Vol. 4, Iss. 3, pp. 232-241
Open Access | Times Cited: 14
Timothy T. Duignan
ACS Physical Chemistry Au (2024) Vol. 4, Iss. 3, pp. 232-241
Open Access | Times Cited: 14
Transferable deep generative modeling of intrinsically disordered protein conformations
Giacomo Janson, Michael Feig
PLoS Computational Biology (2024) Vol. 20, Iss. 5, pp. e1012144-e1012144
Open Access | Times Cited: 11
Giacomo Janson, Michael Feig
PLoS Computational Biology (2024) Vol. 20, Iss. 5, pp. e1012144-e1012144
Open Access | Times Cited: 11
Scientific machine learning for closure models in multiscale problems: a review
Benjamin Sanderse, Panos Stinis, Romit Maulik, et al.
Foundations of Data Science (2024) Vol. 7, Iss. 1, pp. 298-337
Open Access | Times Cited: 10
Benjamin Sanderse, Panos Stinis, Romit Maulik, et al.
Foundations of Data Science (2024) Vol. 7, Iss. 1, pp. 298-337
Open Access | Times Cited: 10
Machine Learning in Soft Matter: From Simulations to Experiments
Kaihua Zhang, Xiangrui Gong, Ying Jiang
Advanced Functional Materials (2024) Vol. 34, Iss. 24
Closed Access | Times Cited: 9
Kaihua Zhang, Xiangrui Gong, Ying Jiang
Advanced Functional Materials (2024) Vol. 34, Iss. 24
Closed Access | Times Cited: 9
Scaling Graph Neural Networks to Large Proteins
Justin Airas, Bin Zhang
Journal of Chemical Theory and Computation (2025)
Open Access | Times Cited: 1
Justin Airas, Bin Zhang
Journal of Chemical Theory and Computation (2025)
Open Access | Times Cited: 1
One bead per residue can describe all-atom protein structures
Lim Heo, Michael Feig
Structure (2023) Vol. 32, Iss. 1, pp. 97-111.e6
Open Access | Times Cited: 22
Lim Heo, Michael Feig
Structure (2023) Vol. 32, Iss. 1, pp. 97-111.e6
Open Access | Times Cited: 22
Statistically Optimal Force Aggregation for Coarse-Graining Molecular Dynamics
Andreas Krämer, Aleksander E. P. Durumeric, Nicholas E. Charron, et al.
The Journal of Physical Chemistry Letters (2023) Vol. 14, Iss. 17, pp. 3970-3979
Open Access | Times Cited: 20
Andreas Krämer, Aleksander E. P. Durumeric, Nicholas E. Charron, et al.
The Journal of Physical Chemistry Letters (2023) Vol. 14, Iss. 17, pp. 3970-3979
Open Access | Times Cited: 20
Perspectives on Ligand/Protein Binding Kinetics Simulations: Force Fields, Machine Learning, Sampling, and User-Friendliness
Paolo Conflitti, Stefano Raniolo, Vittorio Limongelli
Journal of Chemical Theory and Computation (2023) Vol. 19, Iss. 18, pp. 6047-6061
Open Access | Times Cited: 16
Paolo Conflitti, Stefano Raniolo, Vittorio Limongelli
Journal of Chemical Theory and Computation (2023) Vol. 19, Iss. 18, pp. 6047-6061
Open Access | Times Cited: 16
Transferable Implicit Solvation via Contrastive Learning of Graph Neural Networks
Justin Airas, Xinqiang Ding, Bin Zhang
ACS Central Science (2023) Vol. 9, Iss. 12, pp. 2286-2297
Open Access | Times Cited: 14
Justin Airas, Xinqiang Ding, Bin Zhang
ACS Central Science (2023) Vol. 9, Iss. 12, pp. 2286-2297
Open Access | Times Cited: 14
DiAMoNDBack: Diffusion-Denoising Autoregressive Model for Non-Deterministic Backmapping of Cα Protein Traces
Michael S. Jones, Kirill Shmilovich, Andrew L. Ferguson
Journal of Chemical Theory and Computation (2023) Vol. 19, Iss. 21, pp. 7908-7923
Closed Access | Times Cited: 11
Michael S. Jones, Kirill Shmilovich, Andrew L. Ferguson
Journal of Chemical Theory and Computation (2023) Vol. 19, Iss. 21, pp. 7908-7923
Closed Access | Times Cited: 11
Transferable deep generative modeling of intrinsically disordered protein conformations
Giacomo Janson, Michael Feig
bioRxiv (Cold Spring Harbor Laboratory) (2024)
Open Access | Times Cited: 4
Giacomo Janson, Michael Feig
bioRxiv (Cold Spring Harbor Laboratory) (2024)
Open Access | Times Cited: 4
Structural Coarse-Graining via Multiobjective Optimization with Differentiable Simulation
Zhenghao Wu, Tianhang Zhou
Journal of Chemical Theory and Computation (2024) Vol. 20, Iss. 6, pp. 2605-2617
Closed Access | Times Cited: 4
Zhenghao Wu, Tianhang Zhou
Journal of Chemical Theory and Computation (2024) Vol. 20, Iss. 6, pp. 2605-2617
Closed Access | Times Cited: 4
Deciphering driving forces of biomolecular phase separation from simulations
Lars V. Schäfer, Lukas S. Stelzl
Current Opinion in Structural Biology (2025) Vol. 92, pp. 103026-103026
Open Access
Lars V. Schäfer, Lukas S. Stelzl
Current Opinion in Structural Biology (2025) Vol. 92, pp. 103026-103026
Open Access
“Assessing Impact of Hinge Flexibility on Predicted Second Osmotic Virial Coefficients”
Terrance Shoemaker, Brendan R. Amer, Vladimir I. Razinkov, et al.
European Journal of Pharmaceutical Sciences (2025), pp. 107064-107064
Open Access
Terrance Shoemaker, Brendan R. Amer, Vladimir I. Razinkov, et al.
European Journal of Pharmaceutical Sciences (2025), pp. 107064-107064
Open Access
Martini 3 coarse-grained model of enzymes: Framework with validation by all-atom simulations and x-ray diffraction measurements
Mason Hooten, N. Sanjeeva Murthy, Nityananda Pal, et al.
The Journal of Chemical Physics (2025) Vol. 162, Iss. 13
Closed Access
Mason Hooten, N. Sanjeeva Murthy, Nityananda Pal, et al.
The Journal of Chemical Physics (2025) Vol. 162, Iss. 13
Closed Access
Deep learning guided design of dynamic proteins
Amy B Guo, Deniz Akpinaroglu, Mark J. S. Kelly, et al.
bioRxiv (Cold Spring Harbor Laboratory) (2024)
Open Access | Times Cited: 3
Amy B Guo, Deniz Akpinaroglu, Mark J. S. Kelly, et al.
bioRxiv (Cold Spring Harbor Laboratory) (2024)
Open Access | Times Cited: 3
Data-driven dynamical coarse-graining for condensed matter systems
Mauricio J. del Razo, Daan Crommelin, Peter G. Bolhuis
The Journal of Chemical Physics (2024) Vol. 160, Iss. 2
Open Access | Times Cited: 2
Mauricio J. del Razo, Daan Crommelin, Peter G. Bolhuis
The Journal of Chemical Physics (2024) Vol. 160, Iss. 2
Open Access | Times Cited: 2
A perspective on coarse-graining methodologies for biomolecules: resolving self-assembly over extended spatiotemporal scales
Akash Banerjee, Mason Hooten, Nour Srouji, et al.
Frontiers in Soft Matter (2024) Vol. 4
Open Access | Times Cited: 2
Akash Banerjee, Mason Hooten, Nour Srouji, et al.
Frontiers in Soft Matter (2024) Vol. 4
Open Access | Times Cited: 2
Supramolecular Chemistry: Exploring the Use of Electronic Structure, Molecular Dynamics, and Machine Learning Approaches
Matheus Cachoeira Colaço, Vinícius A. Glitz, A. Jacobs, et al.
European Journal of Organic Chemistry (2024) Vol. 27, Iss. 27
Closed Access | Times Cited: 2
Matheus Cachoeira Colaço, Vinícius A. Glitz, A. Jacobs, et al.
European Journal of Organic Chemistry (2024) Vol. 27, Iss. 27
Closed Access | Times Cited: 2