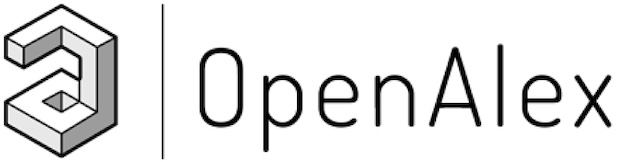
OpenAlex is a bibliographic catalogue of scientific papers, authors and institutions accessible in open access mode, named after the Library of Alexandria. It's citation coverage is excellent and I hope you will find utility in this listing of citing articles!
If you click the article title, you'll navigate to the article, as listed in CrossRef. If you click the Open Access links, you'll navigate to the "best Open Access location". Clicking the citation count will open this listing for that article. Lastly at the bottom of the page, you'll find basic pagination options.
Requested Article:
Sleep classification using Consumer Sleep Technologies and AI: A review of the current landscape
Shagen Djanian, Anders Bruun, Thomas D. Nielsen
Sleep Medicine (2022) Vol. 100, pp. 390-403
Open Access | Times Cited: 22
Shagen Djanian, Anders Bruun, Thomas D. Nielsen
Sleep Medicine (2022) Vol. 100, pp. 390-403
Open Access | Times Cited: 22
Showing 22 citing articles:
The Role of AI in Hospitals and Clinics: Transforming Healthcare in the 21st Century
Shiva Maleki Varnosfaderani, Mohamad Forouzanfar
Bioengineering (2024) Vol. 11, Iss. 4, pp. 337-337
Open Access | Times Cited: 174
Shiva Maleki Varnosfaderani, Mohamad Forouzanfar
Bioengineering (2024) Vol. 11, Iss. 4, pp. 337-337
Open Access | Times Cited: 174
Evaluating Accuracy in Five Commercial Sleep-Tracking Devices Compared to Research-Grade Actigraphy and Polysomnography
Kyle A. Kainec, Jamie Caccavaro, Morgan Barnes, et al.
Sensors (2024) Vol. 24, Iss. 2, pp. 635-635
Open Access | Times Cited: 21
Kyle A. Kainec, Jamie Caccavaro, Morgan Barnes, et al.
Sensors (2024) Vol. 24, Iss. 2, pp. 635-635
Open Access | Times Cited: 21
Evaluating reliability in wearable devices for sleep staging
Vera Birrer, Mohamed Elgendi, Olivier Lambercy, et al.
npj Digital Medicine (2024) Vol. 7, Iss. 1
Open Access | Times Cited: 16
Vera Birrer, Mohamed Elgendi, Olivier Lambercy, et al.
npj Digital Medicine (2024) Vol. 7, Iss. 1
Open Access | Times Cited: 16
Research and application of deep learning-based sleep staging: Data, modeling, validation, and clinical practice
Huijun Yue, Zhuqi Chen, Wenbin Guo, et al.
Sleep Medicine Reviews (2024) Vol. 74, pp. 101897-101897
Open Access | Times Cited: 14
Huijun Yue, Zhuqi Chen, Wenbin Guo, et al.
Sleep Medicine Reviews (2024) Vol. 74, pp. 101897-101897
Open Access | Times Cited: 14
Applying Machine Learning Algorithms for the Classification of Sleep Disorders
Talal Alshammari
IEEE Access (2024) Vol. 12, pp. 36110-36121
Open Access | Times Cited: 9
Talal Alshammari
IEEE Access (2024) Vol. 12, pp. 36110-36121
Open Access | Times Cited: 9
EEGNet classification of sleep EEG for individual specialization based on data augmentation
Mo Xia, Xuyang Zhao, Rui Deng, et al.
Cognitive Neurodynamics (2024) Vol. 18, Iss. 4, pp. 1539-1547
Closed Access | Times Cited: 8
Mo Xia, Xuyang Zhao, Rui Deng, et al.
Cognitive Neurodynamics (2024) Vol. 18, Iss. 4, pp. 1539-1547
Closed Access | Times Cited: 8
Current status and prospects of automatic sleep stages scoring: Review
Maksym Gaiduk, Ángel Serrano Alarcón, Ralf Seepold, et al.
Biomedical Engineering Letters (2023) Vol. 13, Iss. 3, pp. 247-272
Open Access | Times Cited: 21
Maksym Gaiduk, Ángel Serrano Alarcón, Ralf Seepold, et al.
Biomedical Engineering Letters (2023) Vol. 13, Iss. 3, pp. 247-272
Open Access | Times Cited: 21
Digital outcome measures from smartwatch data relate to non-motor features of Parkinson’s disease
Ann‐Kathrin Schalkamp, Neil A. Harrison, Kathryn J. Peall, et al.
npj Parkinson s Disease (2024) Vol. 10, Iss. 1
Open Access | Times Cited: 4
Ann‐Kathrin Schalkamp, Neil A. Harrison, Kathryn J. Peall, et al.
npj Parkinson s Disease (2024) Vol. 10, Iss. 1
Open Access | Times Cited: 4
Classification of Sleep Disorders Using Ensemble Techniques
S. Anandamurugan, G. Sanjuga, S. Viswa, et al.
SSRN Electronic Journal (2025)
Closed Access
S. Anandamurugan, G. Sanjuga, S. Viswa, et al.
SSRN Electronic Journal (2025)
Closed Access
A Comprehensive Review of Home Sleep Monitoring Technologies: Smartphone Apps, Smartwatches, and Smart Mattresses
Bhekumuzi M. Mathunjwa, Randy Yan Jie Kor, Wanida Ngarnkuekool, et al.
Sensors (2025) Vol. 25, Iss. 6, pp. 1771-1771
Open Access
Bhekumuzi M. Mathunjwa, Randy Yan Jie Kor, Wanida Ngarnkuekool, et al.
Sensors (2025) Vol. 25, Iss. 6, pp. 1771-1771
Open Access
EnsembleSleepNet: a novel ensemble deep learning model based on transformers and attention mechanisms using multimodal data for sleep stages classification
Sahar Hassanzadeh Mostafaei, Jafar Tanha, Amir Sharafkhaneh
Applied Intelligence (2025) Vol. 55, Iss. 7
Closed Access
Sahar Hassanzadeh Mostafaei, Jafar Tanha, Amir Sharafkhaneh
Applied Intelligence (2025) Vol. 55, Iss. 7
Closed Access
Consumer Wearable Sleep Trackers
Ambrose A. Chiang, Seema Khosla
Sleep Medicine Clinics (2023) Vol. 18, Iss. 3, pp. 311-330
Closed Access | Times Cited: 8
Ambrose A. Chiang, Seema Khosla
Sleep Medicine Clinics (2023) Vol. 18, Iss. 3, pp. 311-330
Closed Access | Times Cited: 8
Multi-stage sleep classification using photoplethysmographic sensor
Mohammod Abdul Motin, Chandan Karmakar, Marimuthu Palaniswami, et al.
Royal Society Open Science (2023) Vol. 10, Iss. 4
Open Access | Times Cited: 5
Mohammod Abdul Motin, Chandan Karmakar, Marimuthu Palaniswami, et al.
Royal Society Open Science (2023) Vol. 10, Iss. 4
Open Access | Times Cited: 5
Sleep stage prediction using multimodal body network and circadian rhythm
Sahar Waqar, Muhammad Usman Ghani Khan
PeerJ Computer Science (2024) Vol. 10, pp. e1988-e1988
Open Access
Sahar Waqar, Muhammad Usman Ghani Khan
PeerJ Computer Science (2024) Vol. 10, pp. e1988-e1988
Open Access
Validation of ballistocardiography data for predicting the sleep efficiency in healthy individuals and patients with insomnia
Г. В. Ковров, С. И. Посохов, А. Г. Черникова, et al.
S S Korsakov Journal of Neurology and Psychiatry (2024) Vol. 124, Iss. 10, pp. 60-60
Closed Access
Г. В. Ковров, С. И. Посохов, А. Г. Черникова, et al.
S S Korsakov Journal of Neurology and Psychiatry (2024) Vol. 124, Iss. 10, pp. 60-60
Closed Access
Long-term self-supervised learning for accelerometer-based sleep–wake recognition
Aleksej Logacjov, Kerstin Bach, Paul Jarle Mork
Engineering Applications of Artificial Intelligence (2024) Vol. 141, pp. 109758-109758
Open Access
Aleksej Logacjov, Kerstin Bach, Paul Jarle Mork
Engineering Applications of Artificial Intelligence (2024) Vol. 141, pp. 109758-109758
Open Access
Domain Adversarial Convolutional Neural Network Improves the Accuracy and Generalizability of Wearable Sleep Assessment Technology
Adonay S. Nunes, Matthew Patterson, Dawid Gerstel, et al.
Sensors (2024) Vol. 24, Iss. 24, pp. 7982-7982
Open Access
Adonay S. Nunes, Matthew Patterson, Dawid Gerstel, et al.
Sensors (2024) Vol. 24, Iss. 24, pp. 7982-7982
Open Access
Using actigraphy to assess sleep characteristics
Г. А. Трусов, А. В. Коробейникова, Lyubov Getmantseva, et al.
Acta Biomedica Scientifica (East Siberian Biomedical Journal) (2024) Vol. 9, Iss. 6, pp. 100-110
Open Access
Г. А. Трусов, А. В. Коробейникова, Lyubov Getmantseva, et al.
Acta Biomedica Scientifica (East Siberian Biomedical Journal) (2024) Vol. 9, Iss. 6, pp. 100-110
Open Access
Federated Learning for Sleep Stage Classification on Edge Devices via a Model-Agnostic Meta-Learning-Based Pre-Trained Model
SungHwan Moon, Tae Seong Kim, Jihye Ryu, et al.
(2023), pp. 188-192
Closed Access | Times Cited: 1
SungHwan Moon, Tae Seong Kim, Jihye Ryu, et al.
(2023), pp. 188-192
Closed Access | Times Cited: 1
[Pediatric oral maxillofacial management and artificial intelligence].
Shuwen Guan, Dianquan Liu, Qing‐Feng Zhang
PubMed (2023) Vol. 37, Iss. 8, pp. 658-661
Closed Access
Shuwen Guan, Dianquan Liu, Qing‐Feng Zhang
PubMed (2023) Vol. 37, Iss. 8, pp. 658-661
Closed Access
Digital markers from smartwatch data relate to non-motor clinical examinations of Parkinson’s disease
Ann‐Kathrin Schalkamp, Neil A. Harrison, Kathryn J. Peall, et al.
medRxiv (Cold Spring Harbor Laboratory) (2023)
Open Access
Ann‐Kathrin Schalkamp, Neil A. Harrison, Kathryn J. Peall, et al.
medRxiv (Cold Spring Harbor Laboratory) (2023)
Open Access
Classification of Sleep EEG Signals for Individual Specialization by 1D Deep Convolutional Network and Data Augmentation
Mo Xia, Hongxi Xue, Jianting Cao
(2023), pp. 187-194
Closed Access
Mo Xia, Hongxi Xue, Jianting Cao
(2023), pp. 187-194
Closed Access