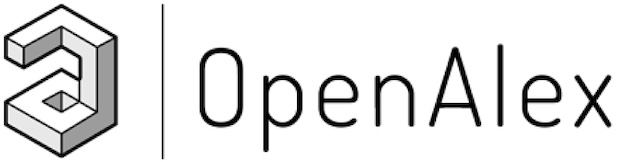
OpenAlex is a bibliographic catalogue of scientific papers, authors and institutions accessible in open access mode, named after the Library of Alexandria. It's citation coverage is excellent and I hope you will find utility in this listing of citing articles!
If you click the article title, you'll navigate to the article, as listed in CrossRef. If you click the Open Access links, you'll navigate to the "best Open Access location". Clicking the citation count will open this listing for that article. Lastly at the bottom of the page, you'll find basic pagination options.
Requested Article:
The Dawes Review 10: The impact of deep learning for the analysis of galaxy surveys
Marc Huertas-Company, François Lanusse
Publications of the Astronomical Society of Australia (2023) Vol. 40
Open Access | Times Cited: 58
Marc Huertas-Company, François Lanusse
Publications of the Astronomical Society of Australia (2023) Vol. 40
Open Access | Times Cited: 58
Showing 1-25 of 58 citing articles:
Astronomia ex machina: a history, primer and outlook on neural networks in astronomy
Michael J. Smith, J. E. Geach
Royal Society Open Science (2023) Vol. 10, Iss. 5
Open Access | Times Cited: 36
Michael J. Smith, J. E. Geach
Royal Society Open Science (2023) Vol. 10, Iss. 5
Open Access | Times Cited: 36
Searching for Strong Gravitational Lenses
C. Lemon, F. Courbin, Anupreeta More, et al.
Space Science Reviews (2024) Vol. 220, Iss. 2
Open Access | Times Cited: 10
C. Lemon, F. Courbin, Anupreeta More, et al.
Space Science Reviews (2024) Vol. 220, Iss. 2
Open Access | Times Cited: 10
AstroCLIP: A cross-modal foundation model for galaxies
Liam Parker, François Lanusse, Siavash Golkar, et al.
Monthly Notices of the Royal Astronomical Society (2024) Vol. 531, Iss. 4, pp. 4990-5011
Open Access | Times Cited: 7
Liam Parker, François Lanusse, Siavash Golkar, et al.
Monthly Notices of the Royal Astronomical Society (2024) Vol. 531, Iss. 4, pp. 4990-5011
Open Access | Times Cited: 7
Zoobot: Adaptable Deep Learning Models for Galaxy Morphology
Mike Walmsley, Campbell Allen, Ben Aussel, et al.
The Journal of Open Source Software (2023) Vol. 8, Iss. 85, pp. 5312-5312
Open Access | Times Cited: 16
Mike Walmsley, Campbell Allen, Ben Aussel, et al.
The Journal of Open Source Software (2023) Vol. 8, Iss. 85, pp. 5312-5312
Open Access | Times Cited: 16
Galaxy morphology from z ∼ 6 through the lens of JWST
Marc Huertas-Company, Kartheik G. Iyer, Eirini Angeloudi, et al.
Astronomy and Astrophysics (2023) Vol. 685, pp. A48-A48
Open Access | Times Cited: 16
Marc Huertas-Company, Kartheik G. Iyer, Eirini Angeloudi, et al.
Astronomy and Astrophysics (2023) Vol. 685, pp. A48-A48
Open Access | Times Cited: 16
Detection, instance segmentation, and classification for astronomical surveys with deep learning (deepdisc ): detectron2 implementation and demonstration with Hyper Suprime-Cam data
Grant Merz, Yichen Liu, Colin J. Burke, et al.
Monthly Notices of the Royal Astronomical Society (2023) Vol. 526, Iss. 1, pp. 1122-1137
Closed Access | Times Cited: 15
Grant Merz, Yichen Liu, Colin J. Burke, et al.
Monthly Notices of the Royal Astronomical Society (2023) Vol. 526, Iss. 1, pp. 1122-1137
Closed Access | Times Cited: 15
Galaxy Zoo DESI: Detailed morphology measurements for 8.7M galaxies in the DESI Legacy Imaging Surveys
Mike Walmsley, Tobias Géron, Sandor Kruk, et al.
Monthly Notices of the Royal Astronomical Society (2023) Vol. 526, Iss. 3, pp. 4768-4786
Open Access | Times Cited: 14
Mike Walmsley, Tobias Géron, Sandor Kruk, et al.
Monthly Notices of the Royal Astronomical Society (2023) Vol. 526, Iss. 3, pp. 4768-4786
Open Access | Times Cited: 14
Galaxy mergers in Subaru HSC-SSP: A deep representation learning approach for identification, and the role of environment on merger incidence
Kiyoaki Christopher Omori, Connor Bottrell, Mike Walmsley, et al.
Astronomy and Astrophysics (2023) Vol. 679, pp. A142-A142
Open Access | Times Cited: 12
Kiyoaki Christopher Omori, Connor Bottrell, Mike Walmsley, et al.
Astronomy and Astrophysics (2023) Vol. 679, pp. A142-A142
Open Access | Times Cited: 12
YOLO-ET: A Machine Learning model for detecting, localising and classifying anthropogenic contaminants and extraterrestrial microparticles optimised for mobile processing systems
L. Pinault, Hajime Yano, Kyoko Okudaira, et al.
Astronomy and Computing (2024) Vol. 47, pp. 100828-100828
Open Access | Times Cited: 4
L. Pinault, Hajime Yano, Kyoko Okudaira, et al.
Astronomy and Computing (2024) Vol. 47, pp. 100828-100828
Open Access | Times Cited: 4
YOLO-CIANNA: Galaxy detection with deep learning in radio data. I. A new YOLO-inspired source detection method applied to the SKAO SDC1
David Cornu, P. Salomé, B. Semelin, et al.
Astronomy and Astrophysics (2024) Vol. 690, pp. A211-A211
Open Access | Times Cited: 4
David Cornu, P. Salomé, B. Semelin, et al.
Astronomy and Astrophysics (2024) Vol. 690, pp. A211-A211
Open Access | Times Cited: 4
Deep Learning-based Detection and Segmentation of Edge-on and Highly Inclined Galaxies
Ž. Chrobáková, Viera Krešňáková, R. Nagy, et al.
Publications of the Astronomical Society of the Pacific (2025) Vol. 137, Iss. 3, pp. 034101-034101
Closed Access
Ž. Chrobáková, Viera Krešňáková, R. Nagy, et al.
Publications of the Astronomical Society of the Pacific (2025) Vol. 137, Iss. 3, pp. 034101-034101
Closed Access
Optimal, fast, and robust inference of reionization-era cosmology with the 21cmPIE-INN
Benedikt Schosser, Caroline Heneka, Tilman Plehn
SciPost Physics Core (2025) Vol. 8, Iss. 2
Open Access
Benedikt Schosser, Caroline Heneka, Tilman Plehn
SciPost Physics Core (2025) Vol. 8, Iss. 2
Open Access
Effective cosmic density field reconstruction with convolutional neural network
Xinyi Chen, Fangzhou Zhu, Sasha Gaines, et al.
Monthly Notices of the Royal Astronomical Society (2023) Vol. 523, Iss. 4, pp. 6272-6281
Open Access | Times Cited: 10
Xinyi Chen, Fangzhou Zhu, Sasha Gaines, et al.
Monthly Notices of the Royal Astronomical Society (2023) Vol. 523, Iss. 4, pp. 6272-6281
Open Access | Times Cited: 10
Galaxy mergers in UNIONS – I. A simulation-driven hybrid deep learning ensemble for pure galaxy merger classification
Leonardo Ferreira, Robert W. Bickley, Sara L. Ellison, et al.
Monthly Notices of the Royal Astronomical Society (2024) Vol. 533, Iss. 3, pp. 2547-2569
Open Access | Times Cited: 3
Leonardo Ferreira, Robert W. Bickley, Sara L. Ellison, et al.
Monthly Notices of the Royal Astronomical Society (2024) Vol. 533, Iss. 3, pp. 2547-2569
Open Access | Times Cited: 3
Insights into Galaxy Evolution from Interpretable Sparse Feature Networks
John F. Wu
The Astrophysical Journal (2025) Vol. 980, Iss. 2, pp. 183-183
Open Access
John F. Wu
The Astrophysical Journal (2025) Vol. 980, Iss. 2, pp. 183-183
Open Access
Semi-empirical models of galaxy formation and evolution
Andrea Lapi, Lumen Boco, Francesco Shankar
Elsevier eBooks (2025)
Closed Access
Andrea Lapi, Lumen Boco, Francesco Shankar
Elsevier eBooks (2025)
Closed Access
DeepAstroUDA: Semi-Supervised Universal Domain Adaptation for Cross-Survey Galaxy Morphology Classification and Anomaly Detection
Aleksandra Ćiprijanović, Ashia Lewis, K. Pedro, et al.
Machine Learning Science and Technology (2023) Vol. 4, Iss. 2, pp. 025013-025013
Open Access | Times Cited: 9
Aleksandra Ćiprijanović, Ashia Lewis, K. Pedro, et al.
Machine Learning Science and Technology (2023) Vol. 4, Iss. 2, pp. 025013-025013
Open Access | Times Cited: 9
Surveying image segmentation approaches in astronomy
Duo Xu, Ye Zhu
Astronomy and Computing (2024) Vol. 48, pp. 100838-100838
Open Access | Times Cited: 3
Duo Xu, Ye Zhu
Astronomy and Computing (2024) Vol. 48, pp. 100838-100838
Open Access | Times Cited: 3
A brief review of contrastive learning applied to astrophysics
Marc Huertas-Company, Regina Sarmiento, J. H. Knapen
RAS Techniques and Instruments (2023) Vol. 2, Iss. 1, pp. 441-452
Open Access | Times Cited: 7
Marc Huertas-Company, Regina Sarmiento, J. H. Knapen
RAS Techniques and Instruments (2023) Vol. 2, Iss. 1, pp. 441-452
Open Access | Times Cited: 7
The three hundred project: mapping the matter distribution in galaxy clusters via deep learning from multiview simulated observations
Daniel de Andrés, Weiguang Cui, Gustavo Yepes, et al.
Monthly Notices of the Royal Astronomical Society (2024) Vol. 528, Iss. 2, pp. 1517-1530
Open Access | Times Cited: 2
Daniel de Andrés, Weiguang Cui, Gustavo Yepes, et al.
Monthly Notices of the Royal Astronomical Society (2024) Vol. 528, Iss. 2, pp. 1517-1530
Open Access | Times Cited: 2
Galaxy merger challenge: A comparison study between machine learning-based detection methods
Berta Margalef-Bentabol, Liming Wang, Antonio La Marca, et al.
Astronomy and Astrophysics (2024) Vol. 687, pp. A24-A24
Open Access | Times Cited: 2
Berta Margalef-Bentabol, Liming Wang, Antonio La Marca, et al.
Astronomy and Astrophysics (2024) Vol. 687, pp. A24-A24
Open Access | Times Cited: 2
Transfer learning for galaxy feature detection: Finding giant star-forming clumps in low-redshift galaxies using Faster Region-based Convolutional Neural Network
Jürgen Popp, H. J. Dickinson, S. Serjeant, et al.
RAS Techniques and Instruments (2024) Vol. 3, Iss. 1, pp. 174-197
Open Access | Times Cited: 2
Jürgen Popp, H. J. Dickinson, S. Serjeant, et al.
RAS Techniques and Instruments (2024) Vol. 3, Iss. 1, pp. 174-197
Open Access | Times Cited: 2
Detecting galaxy tidal features using self-supervised representation learning
Alice Desmons, Sarah Brough, François Lanusse
Monthly Notices of the Royal Astronomical Society (2024) Vol. 531, Iss. 4, pp. 4070-4084
Open Access | Times Cited: 2
Alice Desmons, Sarah Brough, François Lanusse
Monthly Notices of the Royal Astronomical Society (2024) Vol. 531, Iss. 4, pp. 4070-4084
Open Access | Times Cited: 2
CEERS Key Paper. IX. Identifying Galaxy Mergers in CEERS NIRCam Images Using Random Forests and Convolutional Neural Networks
Caitlin Rose, Jeyhan S. Kartaltepe, Gregory F. Snyder, et al.
The Astrophysical Journal Letters (2024) Vol. 976, Iss. 1, pp. L8-L8
Open Access | Times Cited: 2
Caitlin Rose, Jeyhan S. Kartaltepe, Gregory F. Snyder, et al.
The Astrophysical Journal Letters (2024) Vol. 976, Iss. 1, pp. L8-L8
Open Access | Times Cited: 2
Galaxy morphologies revealed with Subaru HSC and super-resolution techniques. II. Environmental dependence of galaxy mergers at z ∼ 2–5
Takatoshi Shibuya, Yohito Ito, K. Asai, et al.
Publications of the Astronomical Society of Japan (2024)
Open Access | Times Cited: 2
Takatoshi Shibuya, Yohito Ito, K. Asai, et al.
Publications of the Astronomical Society of Japan (2024)
Open Access | Times Cited: 2