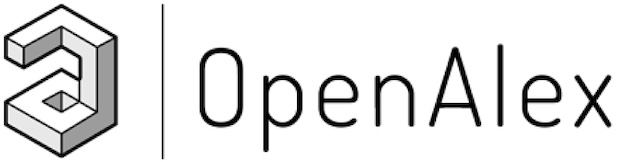
OpenAlex is a bibliographic catalogue of scientific papers, authors and institutions accessible in open access mode, named after the Library of Alexandria. It's citation coverage is excellent and I hope you will find utility in this listing of citing articles!
If you click the article title, you'll navigate to the article, as listed in CrossRef. If you click the Open Access links, you'll navigate to the "best Open Access location". Clicking the citation count will open this listing for that article. Lastly at the bottom of the page, you'll find basic pagination options.
Requested Article:
Strategies and Software for Machine Learning Accelerated Discovery in Transition Metal Chemistry
Aditya Nandy, Chenru Duan, Jon Paul Janet, et al.
Industrial & Engineering Chemistry Research (2018) Vol. 57, Iss. 42, pp. 13973-13986
Open Access | Times Cited: 146
Aditya Nandy, Chenru Duan, Jon Paul Janet, et al.
Industrial & Engineering Chemistry Research (2018) Vol. 57, Iss. 42, pp. 13973-13986
Open Access | Times Cited: 146
Showing 1-25 of 146 citing articles:
The promise of artificial intelligence in chemical engineering: Is it here, finally?
Venkat Venkatasubramanian
AIChE Journal (2018) Vol. 65, Iss. 2, pp. 466-478
Open Access | Times Cited: 532
Venkat Venkatasubramanian
AIChE Journal (2018) Vol. 65, Iss. 2, pp. 466-478
Open Access | Times Cited: 532
Machine Learning for Catalysis Informatics: Recent Applications and Prospects
Takashi Toyao, Zen Maeno, Satoru Takakusagi, et al.
ACS Catalysis (2019) Vol. 10, Iss. 3, pp. 2260-2297
Closed Access | Times Cited: 458
Takashi Toyao, Zen Maeno, Satoru Takakusagi, et al.
ACS Catalysis (2019) Vol. 10, Iss. 3, pp. 2260-2297
Closed Access | Times Cited: 458
Understanding the diversity of the metal-organic framework ecosystem
Seyed Mohamad Moosavi, Aditya Nandy, Kevin Maik Jablonka, et al.
Nature Communications (2020) Vol. 11, Iss. 1
Open Access | Times Cited: 430
Seyed Mohamad Moosavi, Aditya Nandy, Kevin Maik Jablonka, et al.
Nature Communications (2020) Vol. 11, Iss. 1
Open Access | Times Cited: 430
Computational Discovery of Transition-metal Complexes: From High-throughput Screening to Machine Learning
Aditya Nandy, Chenru Duan, Michael G. Taylor, et al.
Chemical Reviews (2021) Vol. 121, Iss. 16, pp. 9927-10000
Closed Access | Times Cited: 218
Aditya Nandy, Chenru Duan, Michael G. Taylor, et al.
Chemical Reviews (2021) Vol. 121, Iss. 16, pp. 9927-10000
Closed Access | Times Cited: 218
Design and Optimization of Catalysts Based on Mechanistic Insights Derived from Quantum Chemical Reaction Modeling
Seihwan Ahn, Mannkyu Hong, Mahesh Sundararajan, et al.
Chemical Reviews (2019) Vol. 119, Iss. 11, pp. 6509-6560
Closed Access | Times Cited: 193
Seihwan Ahn, Mannkyu Hong, Mahesh Sundararajan, et al.
Chemical Reviews (2019) Vol. 119, Iss. 11, pp. 6509-6560
Closed Access | Times Cited: 193
A quantitative uncertainty metric controls error in neural network-driven chemical discovery
Jon Paul Janet, Chenru Duan, Tzuhsiung Yang, et al.
Chemical Science (2019) Vol. 10, Iss. 34, pp. 7913-7922
Open Access | Times Cited: 180
Jon Paul Janet, Chenru Duan, Tzuhsiung Yang, et al.
Chemical Science (2019) Vol. 10, Iss. 34, pp. 7913-7922
Open Access | Times Cited: 180
Automated in Silico Design of Homogeneous Catalysts
Marco Foscato, Vidar R. Jensen
ACS Catalysis (2020) Vol. 10, Iss. 3, pp. 2354-2377
Open Access | Times Cited: 176
Marco Foscato, Vidar R. Jensen
ACS Catalysis (2020) Vol. 10, Iss. 3, pp. 2354-2377
Open Access | Times Cited: 176
Autonomous Discovery in the Chemical Sciences Part I: Progress
Connor W. Coley, Natalie S. Eyke, Klavs F. Jensen
Angewandte Chemie International Edition (2019) Vol. 59, Iss. 51, pp. 22858-22893
Open Access | Times Cited: 175
Connor W. Coley, Natalie S. Eyke, Klavs F. Jensen
Angewandte Chemie International Edition (2019) Vol. 59, Iss. 51, pp. 22858-22893
Open Access | Times Cited: 175
Machine learning dihydrogen activation in the chemical space surrounding Vaska's complex
Pascal Friederich, Gabriel dos Passos Gomes, Riccardo De Bin, et al.
Chemical Science (2020) Vol. 11, Iss. 18, pp. 4584-4601
Open Access | Times Cited: 154
Pascal Friederich, Gabriel dos Passos Gomes, Riccardo De Bin, et al.
Chemical Science (2020) Vol. 11, Iss. 18, pp. 4584-4601
Open Access | Times Cited: 154
Accurate Multiobjective Design in a Space of Millions of Transition Metal Complexes with Neural-Network-Driven Efficient Global Optimization
Jon Paul Janet, Sahasrajit Ramesh, Chenru Duan, et al.
ACS Central Science (2020) Vol. 6, Iss. 4, pp. 513-524
Open Access | Times Cited: 152
Jon Paul Janet, Sahasrajit Ramesh, Chenru Duan, et al.
ACS Central Science (2020) Vol. 6, Iss. 4, pp. 513-524
Open Access | Times Cited: 152
Using Machine Learning and Data Mining to Leverage Community Knowledge for the Engineering of Stable Metal–Organic Frameworks
Aditya Nandy, Chenru Duan, Heather J. Kulik
Journal of the American Chemical Society (2021) Vol. 143, Iss. 42, pp. 17535-17547
Open Access | Times Cited: 148
Aditya Nandy, Chenru Duan, Heather J. Kulik
Journal of the American Chemical Society (2021) Vol. 143, Iss. 42, pp. 17535-17547
Open Access | Times Cited: 148
MOFormer: Self-Supervised Transformer Model for Metal–Organic Framework Property Prediction
Zhonglin Cao, Rishikesh Magar, Yuyang Wang, et al.
Journal of the American Chemical Society (2023) Vol. 145, Iss. 5, pp. 2958-2967
Open Access | Times Cited: 82
Zhonglin Cao, Rishikesh Magar, Yuyang Wang, et al.
Journal of the American Chemical Society (2023) Vol. 145, Iss. 5, pp. 2958-2967
Open Access | Times Cited: 82
Data Generation for Machine Learning Interatomic Potentials and Beyond
Maksim Kulichenko, Benjamin Nebgen, Nicholas Lubbers, et al.
Chemical Reviews (2024) Vol. 124, Iss. 24, pp. 13681-13714
Closed Access | Times Cited: 17
Maksim Kulichenko, Benjamin Nebgen, Nicholas Lubbers, et al.
Chemical Reviews (2024) Vol. 124, Iss. 24, pp. 13681-13714
Closed Access | Times Cited: 17
Designing in the Face of Uncertainty: Exploiting Electronic Structure and Machine Learning Models for Discovery in Inorganic Chemistry
Jon Paul Janet, Fang Liu, Aditya Nandy, et al.
Inorganic Chemistry (2019) Vol. 58, Iss. 16, pp. 10592-10606
Open Access | Times Cited: 119
Jon Paul Janet, Fang Liu, Aditya Nandy, et al.
Inorganic Chemistry (2019) Vol. 58, Iss. 16, pp. 10592-10606
Open Access | Times Cited: 119
Beyond Density Functional Theory: The Multiconfigurational Approach To Model Heterogeneous Catalysis
Carlo Alberto Gaggioli, Samuel J. Stoneburner, Christopher J. Cramer, et al.
ACS Catalysis (2019) Vol. 9, Iss. 9, pp. 8481-8502
Closed Access | Times Cited: 109
Carlo Alberto Gaggioli, Samuel J. Stoneburner, Christopher J. Cramer, et al.
ACS Catalysis (2019) Vol. 9, Iss. 9, pp. 8481-8502
Closed Access | Times Cited: 109
Designing promising molecules for organic solar cells via machine learning assisted virtual screening
Harikrishna Sahu, Feng Yang, Xiaobo Ye, et al.
Journal of Materials Chemistry A (2019) Vol. 7, Iss. 29, pp. 17480-17488
Closed Access | Times Cited: 98
Harikrishna Sahu, Feng Yang, Xiaobo Ye, et al.
Journal of Materials Chemistry A (2019) Vol. 7, Iss. 29, pp. 17480-17488
Closed Access | Times Cited: 98
Representation of molecular structures with persistent homology for machine learning applications in chemistry
Jacob Townsend, Cassie Putman Micucci, John H. Hymel, et al.
Nature Communications (2020) Vol. 11, Iss. 1
Open Access | Times Cited: 98
Jacob Townsend, Cassie Putman Micucci, John H. Hymel, et al.
Nature Communications (2020) Vol. 11, Iss. 1
Open Access | Times Cited: 98
Diversifying Databases of Metal Organic Frameworks for High-Throughput Computational Screening
Sauradeep Majumdar, Seyed Mohamad Moosavi, Kevin Maik Jablonka, et al.
ACS Applied Materials & Interfaces (2021) Vol. 13, Iss. 51, pp. 61004-61014
Open Access | Times Cited: 87
Sauradeep Majumdar, Seyed Mohamad Moosavi, Kevin Maik Jablonka, et al.
ACS Applied Materials & Interfaces (2021) Vol. 13, Iss. 51, pp. 61004-61014
Open Access | Times Cited: 87
Learning from Failure: Predicting Electronic Structure Calculation Outcomes with Machine Learning Models
Chenru Duan, Jon Paul Janet, Fang Liu, et al.
Journal of Chemical Theory and Computation (2019) Vol. 15, Iss. 4, pp. 2331-2345
Open Access | Times Cited: 80
Chenru Duan, Jon Paul Janet, Fang Liu, et al.
Journal of Chemical Theory and Computation (2019) Vol. 15, Iss. 4, pp. 2331-2345
Open Access | Times Cited: 80
Machine Learning Accelerates the Discovery of Design Rules and Exceptions in Stable Metal–Oxo Intermediate Formation
Aditya Nandy, Jiazhou Zhu, Jon Paul Janet, et al.
ACS Catalysis (2019) Vol. 9, Iss. 9, pp. 8243-8255
Open Access | Times Cited: 80
Aditya Nandy, Jiazhou Zhu, Jon Paul Janet, et al.
ACS Catalysis (2019) Vol. 9, Iss. 9, pp. 8243-8255
Open Access | Times Cited: 80
tmQM Dataset—Quantum Geometries and Properties of 86k Transition Metal Complexes
David Balcells, Bastian Bjerkem Skjelstad
Journal of Chemical Information and Modeling (2020) Vol. 60, Iss. 12, pp. 6135-6146
Open Access | Times Cited: 80
David Balcells, Bastian Bjerkem Skjelstad
Journal of Chemical Information and Modeling (2020) Vol. 60, Iss. 12, pp. 6135-6146
Open Access | Times Cited: 80
Machine learning and big data provide crucial insight for future biomaterials discovery and research
Jacob Kerner, Alan B. Dogan, Horst A. von Recum
Acta Biomaterialia (2021) Vol. 130, pp. 54-65
Closed Access | Times Cited: 64
Jacob Kerner, Alan B. Dogan, Horst A. von Recum
Acta Biomaterialia (2021) Vol. 130, pp. 54-65
Closed Access | Times Cited: 64
New Strategies for Direct Methane-to-Methanol Conversion from Active Learning Exploration of 16 Million Catalysts
Aditya Nandy, Chenru Duan, Conrad Goffinet, et al.
JACS Au (2022) Vol. 2, Iss. 5, pp. 1200-1213
Open Access | Times Cited: 55
Aditya Nandy, Chenru Duan, Conrad Goffinet, et al.
JACS Au (2022) Vol. 2, Iss. 5, pp. 1200-1213
Open Access | Times Cited: 55
Unlocking the computational design of metal–organic cages
Andrew Tarzia, Kim E. Jelfs
Chemical Communications (2022) Vol. 58, Iss. 23, pp. 3717-3730
Open Access | Times Cited: 52
Andrew Tarzia, Kim E. Jelfs
Chemical Communications (2022) Vol. 58, Iss. 23, pp. 3717-3730
Open Access | Times Cited: 52
Single-model uncertainty quantification in neural network potentials does not consistently outperform model ensembles
Aik Rui Tan, Shingo Urata, Samuel Goldman, et al.
npj Computational Materials (2023) Vol. 9, Iss. 1
Open Access | Times Cited: 34
Aik Rui Tan, Shingo Urata, Samuel Goldman, et al.
npj Computational Materials (2023) Vol. 9, Iss. 1
Open Access | Times Cited: 34