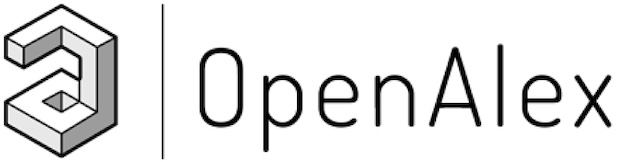
OpenAlex is a bibliographic catalogue of scientific papers, authors and institutions accessible in open access mode, named after the Library of Alexandria. It's citation coverage is excellent and I hope you will find utility in this listing of citing articles!
If you click the article title, you'll navigate to the article, as listed in CrossRef. If you click the Open Access links, you'll navigate to the "best Open Access location". Clicking the citation count will open this listing for that article. Lastly at the bottom of the page, you'll find basic pagination options.
Requested Article:
Denoise Pretraining on Nonequilibrium Molecules for Accurate and Transferable Neural Potentials
Yuyang Wang, Changwen Xu, Zijie Li, et al.
Journal of Chemical Theory and Computation (2023) Vol. 19, Iss. 15, pp. 5077-5087
Open Access | Times Cited: 22
Yuyang Wang, Changwen Xu, Zijie Li, et al.
Journal of Chemical Theory and Computation (2023) Vol. 19, Iss. 15, pp. 5077-5087
Open Access | Times Cited: 22
Showing 22 citing articles:
DPA-2: a large atomic model as a multi-task learner
Duo Zhang, Xinzijian Liu, Xiangyu Zhang, et al.
npj Computational Materials (2024) Vol. 10, Iss. 1
Open Access | Times Cited: 16
Duo Zhang, Xinzijian Liu, Xiangyu Zhang, et al.
npj Computational Materials (2024) Vol. 10, Iss. 1
Open Access | Times Cited: 16
Graph Neural Networks for Molecules
Yuyang Wang, Zijie Li, Amir Barati Farimani
Challenges and advances in computational chemistry and physics (2023), pp. 21-66
Closed Access | Times Cited: 29
Yuyang Wang, Zijie Li, Amir Barati Farimani
Challenges and advances in computational chemistry and physics (2023), pp. 21-66
Closed Access | Times Cited: 29
Machine-learned molecular mechanics force fields from large-scale quantum chemical data
Kenichiro Takaba, Anika J. Friedman, Chapin E. Cavender, et al.
Chemical Science (2024) Vol. 15, Iss. 32, pp. 12861-12878
Open Access | Times Cited: 13
Kenichiro Takaba, Anika J. Friedman, Chapin E. Cavender, et al.
Chemical Science (2024) Vol. 15, Iss. 32, pp. 12861-12878
Open Access | Times Cited: 13
DPA-2: Towards a universal large atomic model for molecular and materials simulation
Han Wang, Duo Zhang, Xinzijian Liu, et al.
Research Square (Research Square) (2024)
Open Access | Times Cited: 8
Han Wang, Duo Zhang, Xinzijian Liu, et al.
Research Square (Research Square) (2024)
Open Access | Times Cited: 8
Synthetic pre-training for neural-network interatomic potentials
John L. A. Gardner, Kathryn T. Baker, Volker L. Deringer
Machine Learning Science and Technology (2023) Vol. 5, Iss. 1, pp. 015003-015003
Open Access | Times Cited: 14
John L. A. Gardner, Kathryn T. Baker, Volker L. Deringer
Machine Learning Science and Technology (2023) Vol. 5, Iss. 1, pp. 015003-015003
Open Access | Times Cited: 14
Evaluation of Rate Coefficients in the Gas Phase Using Machine-Learned Potentials
Carles Martí, Christian Devereux, Habib N. Najm, et al.
The Journal of Physical Chemistry A (2024) Vol. 128, Iss. 10, pp. 1958-1971
Closed Access | Times Cited: 5
Carles Martí, Christian Devereux, Habib N. Najm, et al.
The Journal of Physical Chemistry A (2024) Vol. 128, Iss. 10, pp. 1958-1971
Closed Access | Times Cited: 5
Pre-training with fractional denoising to enhance molecular property prediction
Yuyan Ni, Shikun Feng, Xin Hong, et al.
Nature Machine Intelligence (2024)
Closed Access | Times Cited: 5
Yuyan Ni, Shikun Feng, Xin Hong, et al.
Nature Machine Intelligence (2024)
Closed Access | Times Cited: 5
Machine-learned molecular mechanics force field for the simulation of protein-ligand systems and beyond
Kenichiro Takaba, Iván Pulido, Pavan Kumar Behara, et al.
arXiv (Cornell University) (2023)
Open Access | Times Cited: 12
Kenichiro Takaba, Iván Pulido, Pavan Kumar Behara, et al.
arXiv (Cornell University) (2023)
Open Access | Times Cited: 12
Geometry-enhanced pretraining on interatomic potentials
Taoyong Cui, Chenyu Tang, Mao Su, et al.
Nature Machine Intelligence (2024) Vol. 6, Iss. 4, pp. 428-436
Open Access | Times Cited: 4
Taoyong Cui, Chenyu Tang, Mao Su, et al.
Nature Machine Intelligence (2024) Vol. 6, Iss. 4, pp. 428-436
Open Access | Times Cited: 4
Pretraining graph transformers with atom-in-a-molecule quantum properties for improved ADMET modeling
Alessio Fallani, Ramil Nugmanov, Jose A. Arjona-Medina, et al.
Journal of Cheminformatics (2025) Vol. 17, Iss. 1
Open Access
Alessio Fallani, Ramil Nugmanov, Jose A. Arjona-Medina, et al.
Journal of Cheminformatics (2025) Vol. 17, Iss. 1
Open Access
A Perspective on Foundation Models in Chemistry
Junyoung Choi, Gunwook Nam, Jaesik Choi, et al.
JACS Au (2025)
Open Access
Junyoung Choi, Gunwook Nam, Jaesik Choi, et al.
JACS Au (2025)
Open Access
Derivative-based pre-training of graph neural networks for materials property predictions
Shuyi Jia, Akaash R. Parthasarathy, Rui Feng, et al.
Digital Discovery (2024) Vol. 3, Iss. 3, pp. 586-593
Open Access | Times Cited: 3
Shuyi Jia, Akaash R. Parthasarathy, Rui Feng, et al.
Digital Discovery (2024) Vol. 3, Iss. 3, pp. 586-593
Open Access | Times Cited: 3
Molecular relaxation by reverse diffusion with time step prediction
Khaled Kahouli, Stefaan S. P. Hessmann, Klaus‐Robert Müller, et al.
Machine Learning Science and Technology (2024) Vol. 5, Iss. 3, pp. 035038-035038
Open Access | Times Cited: 3
Khaled Kahouli, Stefaan S. P. Hessmann, Klaus‐Robert Müller, et al.
Machine Learning Science and Technology (2024) Vol. 5, Iss. 3, pp. 035038-035038
Open Access | Times Cited: 3
Online test-time adaptation for better generalization of interatomic potentials to out-of-distribution data
Taoyong Cui, Chenyu Tang, Dongzhan Zhou, et al.
Nature Communications (2025) Vol. 16, Iss. 1
Open Access
Taoyong Cui, Chenyu Tang, Dongzhan Zhou, et al.
Nature Communications (2025) Vol. 16, Iss. 1
Open Access
Online Test-time Adaptation for Interatomic Potentials
Shufei Zhang, Taoyong Cui, Chenyu Tang, et al.
Research Square (Research Square) (2024)
Open Access | Times Cited: 1
Shufei Zhang, Taoyong Cui, Chenyu Tang, et al.
Research Square (Research Square) (2024)
Open Access | Times Cited: 1
Graph Neural Network Integrating Self-Supervised Pretraining for Precise and Interpretable Prediction of Micropollutant Treatability by HO•-Based Advanced Oxidation Processes
Jingyi Zhu, Yuanxi Huang, Lingjun Bu, et al.
ACS ES&T Engineering (2024)
Closed Access | Times Cited: 1
Jingyi Zhu, Yuanxi Huang, Lingjun Bu, et al.
ACS ES&T Engineering (2024)
Closed Access | Times Cited: 1
Atom-Level Quantum Pretraining Enhances the Spectral Perception of Molecular Graphs in Graphormer
Alessio Fallani, Jose A. Arjona-Medina, Konstantin Chernichenko, et al.
Lecture notes in computer science (2024), pp. 71-81
Open Access | Times Cited: 1
Alessio Fallani, Jose A. Arjona-Medina, Konstantin Chernichenko, et al.
Lecture notes in computer science (2024), pp. 71-81
Open Access | Times Cited: 1
Transfer learning for accurate description of atomic transport in Al–Cu melts
E. O. Khazieva, N. M. Chtchelkatchev, R. E. Ryltsev
The Journal of Chemical Physics (2024) Vol. 161, Iss. 17
Closed Access | Times Cited: 1
E. O. Khazieva, N. M. Chtchelkatchev, R. E. Ryltsev
The Journal of Chemical Physics (2024) Vol. 161, Iss. 17
Closed Access | Times Cited: 1
GPIP: Geometry-enhanced Pre-training on Interatomic Potentials
Taoyong Cui, Chenyu Tang, Mao Su, et al.
arXiv (Cornell University) (2023)
Open Access | Times Cited: 2
Taoyong Cui, Chenyu Tang, Mao Su, et al.
arXiv (Cornell University) (2023)
Open Access | Times Cited: 2
Masked pretraining strategy for neural potentials
Z. Zhang, Zijie Li, Amir Barati Farimani
AIP Advances (2024) Vol. 14, Iss. 9
Open Access
Z. Zhang, Zijie Li, Amir Barati Farimani
AIP Advances (2024) Vol. 14, Iss. 9
Open Access
Data-Based Prediction of Redox Potentials via Introducing Chemical Features into the Transformer Architecture
Zhan Si, Deguang Liu, Wan Nie, et al.
Journal of Chemical Information and Modeling (2024) Vol. 64, Iss. 22, pp. 8453-8463
Closed Access
Zhan Si, Deguang Liu, Wan Nie, et al.
Journal of Chemical Information and Modeling (2024) Vol. 64, Iss. 22, pp. 8453-8463
Closed Access
GPIP: Geometry-enhanced Pre-training on Interatomic Potentials
Mao Su, Shufei Zhang, Taoyong Cui, et al.
Research Square (Research Square) (2023)
Open Access
Mao Su, Shufei Zhang, Taoyong Cui, et al.
Research Square (Research Square) (2023)
Open Access