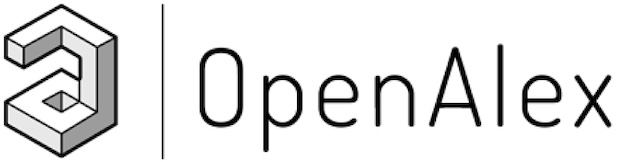
OpenAlex is a bibliographic catalogue of scientific papers, authors and institutions accessible in open access mode, named after the Library of Alexandria. It's citation coverage is excellent and I hope you will find utility in this listing of citing articles!
If you click the article title, you'll navigate to the article, as listed in CrossRef. If you click the Open Access links, you'll navigate to the "best Open Access location". Clicking the citation count will open this listing for that article. Lastly at the bottom of the page, you'll find basic pagination options.
Requested Article:
Statistically Optimal Force Aggregation for Coarse-Graining Molecular Dynamics
Andreas Krämer, Aleksander E. P. Durumeric, Nicholas E. Charron, et al.
The Journal of Physical Chemistry Letters (2023) Vol. 14, Iss. 17, pp. 3970-3979
Open Access | Times Cited: 20
Andreas Krämer, Aleksander E. P. Durumeric, Nicholas E. Charron, et al.
The Journal of Physical Chemistry Letters (2023) Vol. 14, Iss. 17, pp. 3970-3979
Open Access | Times Cited: 20
Showing 20 citing articles:
Perspective: Atomistic simulations of water and aqueous systems with machine learning potentials
Amir Omranpour, Pablo Montero de Hijes, Jörg Behler, et al.
The Journal of Chemical Physics (2024) Vol. 160, Iss. 17
Open Access | Times Cited: 20
Amir Omranpour, Pablo Montero de Hijes, Jörg Behler, et al.
The Journal of Chemical Physics (2024) Vol. 160, Iss. 17
Open Access | Times Cited: 20
OpenMSCG: A Software Tool for Bottom-Up Coarse-Graining
Yuxing Peng, Alexander J. Pak, Aleksander E. P. Durumeric, et al.
The Journal of Physical Chemistry B (2023) Vol. 127, Iss. 40, pp. 8537-8550
Open Access | Times Cited: 32
Yuxing Peng, Alexander J. Pak, Aleksander E. P. Durumeric, et al.
The Journal of Physical Chemistry B (2023) Vol. 127, Iss. 40, pp. 8537-8550
Open Access | Times Cited: 32
DiAMoNDBack: Diffusion-Denoising Autoregressive Model for Non-Deterministic Backmapping of Cα Protein Traces
Michael S. Jones, Kirill Shmilovich, Andrew L. Ferguson
Journal of Chemical Theory and Computation (2023) Vol. 19, Iss. 21, pp. 7908-7923
Closed Access | Times Cited: 11
Michael S. Jones, Kirill Shmilovich, Andrew L. Ferguson
Journal of Chemical Theory and Computation (2023) Vol. 19, Iss. 21, pp. 7908-7923
Closed Access | Times Cited: 11
Structural Coarse-Graining via Multiobjective Optimization with Differentiable Simulation
Zhenghao Wu, Tianhang Zhou
Journal of Chemical Theory and Computation (2024) Vol. 20, Iss. 6, pp. 2605-2617
Closed Access | Times Cited: 4
Zhenghao Wu, Tianhang Zhou
Journal of Chemical Theory and Computation (2024) Vol. 20, Iss. 6, pp. 2605-2617
Closed Access | Times Cited: 4
On the emergence of machine-learning methods in bottom-up coarse-graining
Patrick G. Sahrmann, Gregory A. Voth
Current Opinion in Structural Biology (2025) Vol. 90, pp. 102972-102972
Closed Access
Patrick G. Sahrmann, Gregory A. Voth
Current Opinion in Structural Biology (2025) Vol. 90, pp. 102972-102972
Closed Access
Distilling coarse-grained representations of molecular electronic structure with continuously gated message passing
J. Charlie Maier, Chun-I Wang, Nicholas E. Jackson
The Journal of Chemical Physics (2024) Vol. 160, Iss. 2
Open Access | Times Cited: 3
J. Charlie Maier, Chun-I Wang, Nicholas E. Jackson
The Journal of Chemical Physics (2024) Vol. 160, Iss. 2
Open Access | Times Cited: 3
AMARO: All Heavy-Atom Transferable Neural Network Potentials of Protein Thermodynamics
Antonio Mirarchi, Raúl P. Peláez, Guillem Simeon, et al.
Journal of Chemical Theory and Computation (2024) Vol. 20, Iss. 22, pp. 9871-9878
Open Access | Times Cited: 2
Antonio Mirarchi, Raúl P. Peláez, Guillem Simeon, et al.
Journal of Chemical Theory and Computation (2024) Vol. 20, Iss. 22, pp. 9871-9878
Open Access | Times Cited: 2
Enhancing the Assembly Properties of Bottom-Up Coarse-Grained Phospholipids
Patrick G. Sahrmann, Gregory A. Voth
Journal of Chemical Theory and Computation (2024) Vol. 20, Iss. 22, pp. 10235-10246
Closed Access | Times Cited: 2
Patrick G. Sahrmann, Gregory A. Voth
Journal of Chemical Theory and Computation (2024) Vol. 20, Iss. 22, pp. 10235-10246
Closed Access | Times Cited: 2
Developing an Implicit Solvation Machine Learning Model for Molecular Simulations of Ionic Media
Amaury Coste, Ema Slejko, Julija Zavadlav, et al.
Journal of Chemical Theory and Computation (2023) Vol. 20, Iss. 1, pp. 411-420
Open Access | Times Cited: 5
Amaury Coste, Ema Slejko, Julija Zavadlav, et al.
Journal of Chemical Theory and Computation (2023) Vol. 20, Iss. 1, pp. 411-420
Open Access | Times Cited: 5
A temperature-dependent length-scale for transferable local density potentials
Ryan J. Szukalo, W. G. Noid
The Journal of Chemical Physics (2023) Vol. 159, Iss. 7
Closed Access | Times Cited: 4
Ryan J. Szukalo, W. G. Noid
The Journal of Chemical Physics (2023) Vol. 159, Iss. 7
Closed Access | Times Cited: 4
Neural potentials of proteins extrapolate beyond training data
Geemi P. Wellawatte, Glen M. Hocky, Andrew Dickson White
The Journal of Chemical Physics (2023) Vol. 159, Iss. 8
Open Access | Times Cited: 4
Geemi P. Wellawatte, Glen M. Hocky, Andrew Dickson White
The Journal of Chemical Physics (2023) Vol. 159, Iss. 8
Open Access | Times Cited: 4
Thermodynamic Transferability in Coarse-Grained Force Fields Using Graph Neural Networks
Emily Shinkle, Aleksandra Pachalieva, Riti Bahl, et al.
Journal of Chemical Theory and Computation (2024) Vol. 20, Iss. 23, pp. 10524-10539
Open Access | Times Cited: 1
Emily Shinkle, Aleksandra Pachalieva, Riti Bahl, et al.
Journal of Chemical Theory and Computation (2024) Vol. 20, Iss. 23, pp. 10524-10539
Open Access | Times Cited: 1
Neural potentials of proteins extrapolate beyond training data
Geemi P. Wellawatte, Glen M. Hocky, Andrew Dickson White
(2023)
Open Access | Times Cited: 2
Geemi P. Wellawatte, Glen M. Hocky, Andrew Dickson White
(2023)
Open Access | Times Cited: 2
OpenMSCG: A Software Tool for Bottom-up Coarse-graining
Yuxing Peng, Alexander J. Pak, Aleksander E. P. Durumeric, et al.
(2023)
Open Access | Times Cited: 2
Yuxing Peng, Alexander J. Pak, Aleksander E. P. Durumeric, et al.
(2023)
Open Access | Times Cited: 2
Navigating protein landscapes with a machine-learned transferable coarse-grained model
Cecilia Clementi, Nicholas E. Charron, Félix Musil, et al.
Research Square (Research Square) (2023)
Open Access | Times Cited: 2
Cecilia Clementi, Nicholas E. Charron, Félix Musil, et al.
Research Square (Research Square) (2023)
Open Access | Times Cited: 2
Insight into the Density-Dependence of Pair Potentials for Predictive Coarse-Grained Models
Maria C. Lesniewski, W. G. Noid
The Journal of Physical Chemistry B (2024) Vol. 128, Iss. 5, pp. 1298-1316
Closed Access
Maria C. Lesniewski, W. G. Noid
The Journal of Physical Chemistry B (2024) Vol. 128, Iss. 5, pp. 1298-1316
Closed Access
Accurate nuclear quantum statistics on machine-learned classical effective potentials
Iryna Zaporozhets, Félix Musil, Venkat Kapil, et al.
The Journal of Chemical Physics (2024) Vol. 161, Iss. 13
Open Access
Iryna Zaporozhets, Félix Musil, Venkat Kapil, et al.
The Journal of Chemical Physics (2024) Vol. 161, Iss. 13
Open Access
Distilling Coarse-Grained Representations of Molecular Electronic Structure with Continuously Gated Message Passing
Charlie Maier, Chun-I Wang, Nicholas E. Jackson
(2023)
Open Access | Times Cited: 1
Charlie Maier, Chun-I Wang, Nicholas E. Jackson
(2023)
Open Access | Times Cited: 1
Structural Coarse-Graining via Multi-Objective Optimization with Differentiable Simulation
Zhenghao Wu, Tianhang Zhou
(2023)
Open Access
Zhenghao Wu, Tianhang Zhou
(2023)
Open Access
Structural Coarse-Graining via Multi-Objective Optimization with Differentiable Simulation
Zhenghao Wu, Tianhang Zhou
(2023)
Open Access
Zhenghao Wu, Tianhang Zhou
(2023)
Open Access