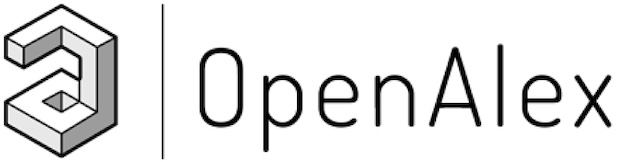
OpenAlex is a bibliographic catalogue of scientific papers, authors and institutions accessible in open access mode, named after the Library of Alexandria. It's citation coverage is excellent and I hope you will find utility in this listing of citing articles!
If you click the article title, you'll navigate to the article, as listed in CrossRef. If you click the Open Access links, you'll navigate to the "best Open Access location". Clicking the citation count will open this listing for that article. Lastly at the bottom of the page, you'll find basic pagination options.
Requested Article:
Machine Learning Accelerates the Discovery of Design Rules and Exceptions in Stable Metal–Oxo Intermediate Formation
Aditya Nandy, Jiazhou Zhu, Jon Paul Janet, et al.
ACS Catalysis (2019) Vol. 9, Iss. 9, pp. 8243-8255
Open Access | Times Cited: 80
Aditya Nandy, Jiazhou Zhu, Jon Paul Janet, et al.
ACS Catalysis (2019) Vol. 9, Iss. 9, pp. 8243-8255
Open Access | Times Cited: 80
Showing 1-25 of 80 citing articles:
Big-Data Science in Porous Materials: Materials Genomics and Machine Learning
Kevin Maik Jablonka, Daniele Ongari, Seyed Mohamad Moosavi, et al.
Chemical Reviews (2020) Vol. 120, Iss. 16, pp. 8066-8129
Open Access | Times Cited: 464
Kevin Maik Jablonka, Daniele Ongari, Seyed Mohamad Moosavi, et al.
Chemical Reviews (2020) Vol. 120, Iss. 16, pp. 8066-8129
Open Access | Times Cited: 464
Understanding the diversity of the metal-organic framework ecosystem
Seyed Mohamad Moosavi, Aditya Nandy, Kevin Maik Jablonka, et al.
Nature Communications (2020) Vol. 11, Iss. 1
Open Access | Times Cited: 430
Seyed Mohamad Moosavi, Aditya Nandy, Kevin Maik Jablonka, et al.
Nature Communications (2020) Vol. 11, Iss. 1
Open Access | Times Cited: 430
The Role of Machine Learning in the Understanding and Design of Materials
Seyed Mohamad Moosavi, Kevin Maik Jablonka, Berend Smit
Journal of the American Chemical Society (2020) Vol. 142, Iss. 48, pp. 20273-20287
Open Access | Times Cited: 308
Seyed Mohamad Moosavi, Kevin Maik Jablonka, Berend Smit
Journal of the American Chemical Society (2020) Vol. 142, Iss. 48, pp. 20273-20287
Open Access | Times Cited: 308
Computational Discovery of Transition-metal Complexes: From High-throughput Screening to Machine Learning
Aditya Nandy, Chenru Duan, Michael G. Taylor, et al.
Chemical Reviews (2021) Vol. 121, Iss. 16, pp. 9927-10000
Closed Access | Times Cited: 218
Aditya Nandy, Chenru Duan, Michael G. Taylor, et al.
Chemical Reviews (2021) Vol. 121, Iss. 16, pp. 9927-10000
Closed Access | Times Cited: 218
Combining Machine Learning and Computational Chemistry for Predictive Insights Into Chemical Systems
John A. Keith, Valentín Vassilev-Galindo, Bingqing Cheng, et al.
Chemical Reviews (2021) Vol. 121, Iss. 16, pp. 9816-9872
Open Access | Times Cited: 180
John A. Keith, Valentín Vassilev-Galindo, Bingqing Cheng, et al.
Chemical Reviews (2021) Vol. 121, Iss. 16, pp. 9816-9872
Open Access | Times Cited: 180
Automated in Silico Design of Homogeneous Catalysts
Marco Foscato, Vidar R. Jensen
ACS Catalysis (2020) Vol. 10, Iss. 3, pp. 2354-2377
Open Access | Times Cited: 176
Marco Foscato, Vidar R. Jensen
ACS Catalysis (2020) Vol. 10, Iss. 3, pp. 2354-2377
Open Access | Times Cited: 176
Accurate Multiobjective Design in a Space of Millions of Transition Metal Complexes with Neural-Network-Driven Efficient Global Optimization
Jon Paul Janet, Sahasrajit Ramesh, Chenru Duan, et al.
ACS Central Science (2020) Vol. 6, Iss. 4, pp. 513-524
Open Access | Times Cited: 152
Jon Paul Janet, Sahasrajit Ramesh, Chenru Duan, et al.
ACS Central Science (2020) Vol. 6, Iss. 4, pp. 513-524
Open Access | Times Cited: 152
Molecular and heterogeneous water oxidation catalysts: recent progress and joint perspectives
Jingguo Li, Carlos A. Triana, Wenchao Wan, et al.
Chemical Society Reviews (2021) Vol. 50, Iss. 4, pp. 2444-2485
Open Access | Times Cited: 145
Jingguo Li, Carlos A. Triana, Wenchao Wan, et al.
Chemical Society Reviews (2021) Vol. 50, Iss. 4, pp. 2444-2485
Open Access | Times Cited: 145
Machine learning reveals key ion selectivity mechanisms in polymeric membranes with subnanometer pores
Cody L. Ritt, Mingjie Liu, Tuan Anh Pham, et al.
Science Advances (2022) Vol. 8, Iss. 2
Open Access | Times Cited: 85
Cody L. Ritt, Mingjie Liu, Tuan Anh Pham, et al.
Science Advances (2022) Vol. 8, Iss. 2
Open Access | Times Cited: 85
Chemical Design of Metal Complexes for Electrochemical Water Oxidation under Acidic Conditions
Zhen‐Tao Yu
Dalton Transactions (2025)
Closed Access | Times Cited: 2
Zhen‐Tao Yu
Dalton Transactions (2025)
Closed Access | Times Cited: 2
Deep dive into machine learning density functional theory for materials science and chemistry
Lenz Fiedler, Karan Shah, Michael Bußmann, et al.
Physical Review Materials (2022) Vol. 6, Iss. 4
Open Access | Times Cited: 69
Lenz Fiedler, Karan Shah, Michael Bußmann, et al.
Physical Review Materials (2022) Vol. 6, Iss. 4
Open Access | Times Cited: 69
Encoding the atomic structure for machine learning in materials science
Shunning Li, Yuanji Liu, Dong Chen, et al.
Wiley Interdisciplinary Reviews Computational Molecular Science (2021) Vol. 12, Iss. 1
Closed Access | Times Cited: 67
Shunning Li, Yuanji Liu, Dong Chen, et al.
Wiley Interdisciplinary Reviews Computational Molecular Science (2021) Vol. 12, Iss. 1
Closed Access | Times Cited: 67
New Strategies for Direct Methane-to-Methanol Conversion from Active Learning Exploration of 16 Million Catalysts
Aditya Nandy, Chenru Duan, Conrad Goffinet, et al.
JACS Au (2022) Vol. 2, Iss. 5, pp. 1200-1213
Open Access | Times Cited: 55
Aditya Nandy, Chenru Duan, Conrad Goffinet, et al.
JACS Au (2022) Vol. 2, Iss. 5, pp. 1200-1213
Open Access | Times Cited: 55
Design and Application of a Screening Set for Monophosphine Ligands in Cross-Coupling
Tobias Gensch, Sleight R. Smith, Thomas J. Colacot, et al.
ACS Catalysis (2022) Vol. 12, Iss. 13, pp. 7773-7780
Closed Access | Times Cited: 44
Tobias Gensch, Sleight R. Smith, Thomas J. Colacot, et al.
ACS Catalysis (2022) Vol. 12, Iss. 13, pp. 7773-7780
Closed Access | Times Cited: 44
Low-cost machine learning prediction of excited state properties of iridium-centered phosphors
Gianmarco Terrones, Chenru Duan, Aditya Nandy, et al.
Chemical Science (2023) Vol. 14, Iss. 6, pp. 1419-1433
Open Access | Times Cited: 25
Gianmarco Terrones, Chenru Duan, Aditya Nandy, et al.
Chemical Science (2023) Vol. 14, Iss. 6, pp. 1419-1433
Open Access | Times Cited: 25
Computational Discovery of Stable Metal–Organic Frameworks for Methane-to-Methanol Catalysis
Husain Adamji, Aditya Nandy, Ilia Kevlishvili, et al.
Journal of the American Chemical Society (2023) Vol. 145, Iss. 26, pp. 14365-14378
Closed Access | Times Cited: 24
Husain Adamji, Aditya Nandy, Ilia Kevlishvili, et al.
Journal of the American Chemical Society (2023) Vol. 145, Iss. 26, pp. 14365-14378
Closed Access | Times Cited: 24
ReDD-COFFEE: a ready-to-use database of covalent organic framework structures and accurate force fields to enable high-throughput screenings
Juul S. De Vos, Sander Borgmans, Pascal Van Der Voort, et al.
Journal of Materials Chemistry A (2023) Vol. 11, Iss. 14, pp. 7468-7487
Open Access | Times Cited: 23
Juul S. De Vos, Sander Borgmans, Pascal Van Der Voort, et al.
Journal of Materials Chemistry A (2023) Vol. 11, Iss. 14, pp. 7468-7487
Open Access | Times Cited: 23
High-Throughput Screening of Covalent Organic Frameworks for Carbon Capture Using Machine Learning
Juul S. De Vos, S. Ravichandran, Sander Borgmans, et al.
Chemistry of Materials (2024) Vol. 36, Iss. 9, pp. 4315-4330
Open Access | Times Cited: 13
Juul S. De Vos, S. Ravichandran, Sander Borgmans, et al.
Chemistry of Materials (2024) Vol. 36, Iss. 9, pp. 4315-4330
Open Access | Times Cited: 13
Prediction of hydration energies of adsorbates at Pt(111) and liquid water interfaces using machine learning
Jiexin Shi, Xiaohong Zhang, Venkata Rohit Punyapu, et al.
The Journal of Chemical Physics (2025) Vol. 162, Iss. 8
Closed Access | Times Cited: 1
Jiexin Shi, Xiaohong Zhang, Venkata Rohit Punyapu, et al.
The Journal of Chemical Physics (2025) Vol. 162, Iss. 8
Closed Access | Times Cited: 1
Quantum-mechanical transition-state model combined with machine learning provides catalyst design features for selective Cr olefin oligomerization
Steven M. Maley, Doo‐Hyun Kwon, Nick E. Rollins, et al.
Chemical Science (2020) Vol. 11, Iss. 35, pp. 9665-9674
Open Access | Times Cited: 66
Steven M. Maley, Doo‐Hyun Kwon, Nick E. Rollins, et al.
Chemical Science (2020) Vol. 11, Iss. 35, pp. 9665-9674
Open Access | Times Cited: 66
Machine Learning in Chemistry
Jon Paul Janet, Heather J. Kulik
ACS in focus (2020)
Closed Access | Times Cited: 66
Jon Paul Janet, Heather J. Kulik
ACS in focus (2020)
Closed Access | Times Cited: 66
In silicohigh throughput screening of bimetallic and single atom alloys using machine learning andab initiomicrokinetic modelling
S Saxena, Tuhin Suvra Khan, Fatima Jalid, et al.
Journal of Materials Chemistry A (2019) Vol. 8, Iss. 1, pp. 107-123
Closed Access | Times Cited: 64
S Saxena, Tuhin Suvra Khan, Fatima Jalid, et al.
Journal of Materials Chemistry A (2019) Vol. 8, Iss. 1, pp. 107-123
Closed Access | Times Cited: 64
Seeing Is Believing: Experimental Spin States from Machine Learning Model Structure Predictions
Michael G. Taylor, Tzuhsiung Yang, Sean Lin, et al.
The Journal of Physical Chemistry A (2020) Vol. 124, Iss. 16, pp. 3286-3299
Open Access | Times Cited: 61
Michael G. Taylor, Tzuhsiung Yang, Sean Lin, et al.
The Journal of Physical Chemistry A (2020) Vol. 124, Iss. 16, pp. 3286-3299
Open Access | Times Cited: 61
Progress in Computational and Machine‐Learning Methods for Heterogeneous Small‐Molecule Activation
Geun Ho Gu, Changhyeok Choi, Yeunhee Lee, et al.
Advanced Materials (2020) Vol. 32, Iss. 35
Closed Access | Times Cited: 55
Geun Ho Gu, Changhyeok Choi, Yeunhee Lee, et al.
Advanced Materials (2020) Vol. 32, Iss. 35
Closed Access | Times Cited: 55
Navigating Transition-Metal Chemical Space: Artificial Intelligence for First-Principles Design
Jon Paul Janet, Chenru Duan, Aditya Nandy, et al.
Accounts of Chemical Research (2021) Vol. 54, Iss. 3, pp. 532-545
Open Access | Times Cited: 55
Jon Paul Janet, Chenru Duan, Aditya Nandy, et al.
Accounts of Chemical Research (2021) Vol. 54, Iss. 3, pp. 532-545
Open Access | Times Cited: 55