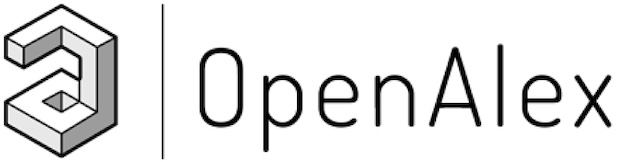
OpenAlex is a bibliographic catalogue of scientific papers, authors and institutions accessible in open access mode, named after the Library of Alexandria. It's citation coverage is excellent and I hope you will find utility in this listing of citing articles!
If you click the article title, you'll navigate to the article, as listed in CrossRef. If you click the Open Access links, you'll navigate to the "best Open Access location". Clicking the citation count will open this listing for that article. Lastly at the bottom of the page, you'll find basic pagination options.
Requested Article:
A quantitative uncertainty metric controls error in neural network-driven chemical discovery
Jon Paul Janet, Chenru Duan, Tzuhsiung Yang, et al.
Chemical Science (2019) Vol. 10, Iss. 34, pp. 7913-7922
Open Access | Times Cited: 180
Jon Paul Janet, Chenru Duan, Tzuhsiung Yang, et al.
Chemical Science (2019) Vol. 10, Iss. 34, pp. 7913-7922
Open Access | Times Cited: 180
Showing 1-25 of 180 citing articles:
Gaussian Process Regression for Materials and Molecules
Volker L. Deringer, Albert P. Bartók, Noam Bernstein, et al.
Chemical Reviews (2021) Vol. 121, Iss. 16, pp. 10073-10141
Open Access | Times Cited: 784
Volker L. Deringer, Albert P. Bartók, Noam Bernstein, et al.
Chemical Reviews (2021) Vol. 121, Iss. 16, pp. 10073-10141
Open Access | Times Cited: 784
Drug discovery with explainable artificial intelligence
José Jiménez-Luna, Francesca Grisoni, Gisbert Schneider
Nature Machine Intelligence (2020) Vol. 2, Iss. 10, pp. 573-584
Open Access | Times Cited: 724
José Jiménez-Luna, Francesca Grisoni, Gisbert Schneider
Nature Machine Intelligence (2020) Vol. 2, Iss. 10, pp. 573-584
Open Access | Times Cited: 724
Big-Data Science in Porous Materials: Materials Genomics and Machine Learning
Kevin Maik Jablonka, Daniele Ongari, Seyed Mohamad Moosavi, et al.
Chemical Reviews (2020) Vol. 120, Iss. 16, pp. 8066-8129
Open Access | Times Cited: 464
Kevin Maik Jablonka, Daniele Ongari, Seyed Mohamad Moosavi, et al.
Chemical Reviews (2020) Vol. 120, Iss. 16, pp. 8066-8129
Open Access | Times Cited: 464
Quantum Chemistry in the Age of Machine Learning
Pavlo O. Dral
The Journal of Physical Chemistry Letters (2020) Vol. 11, Iss. 6, pp. 2336-2347
Closed Access | Times Cited: 413
Pavlo O. Dral
The Journal of Physical Chemistry Letters (2020) Vol. 11, Iss. 6, pp. 2336-2347
Closed Access | Times Cited: 413
Predicting into unknown space? Estimating the area of applicability of spatial prediction models
Hanna Meyer, Edzer Pebesma
Methods in Ecology and Evolution (2021) Vol. 12, Iss. 9, pp. 1620-1633
Open Access | Times Cited: 354
Hanna Meyer, Edzer Pebesma
Methods in Ecology and Evolution (2021) Vol. 12, Iss. 9, pp. 1620-1633
Open Access | Times Cited: 354
On-the-fly active learning of interpretable Bayesian force fields for atomistic rare events
Jonathan Vandermause, Steven B. Torrisi, Simon Batzner, et al.
npj Computational Materials (2020) Vol. 6, Iss. 1
Open Access | Times Cited: 349
Jonathan Vandermause, Steven B. Torrisi, Simon Batzner, et al.
npj Computational Materials (2020) Vol. 6, Iss. 1
Open Access | Times Cited: 349
Machine Learning for Electronically Excited States of Molecules
Julia Westermayr, Philipp Marquetand
Chemical Reviews (2020) Vol. 121, Iss. 16, pp. 9873-9926
Open Access | Times Cited: 343
Julia Westermayr, Philipp Marquetand
Chemical Reviews (2020) Vol. 121, Iss. 16, pp. 9873-9926
Open Access | Times Cited: 343
Machine learning for interatomic potential models
Tim Mueller, Alberto Hernández, Chuhong Wang
The Journal of Chemical Physics (2020) Vol. 152, Iss. 5
Open Access | Times Cited: 319
Tim Mueller, Alberto Hernández, Chuhong Wang
The Journal of Chemical Physics (2020) Vol. 152, Iss. 5
Open Access | Times Cited: 319
Constrained Bayesian optimization for automatic chemical design using variational autoencoders
Ryan‐Rhys Griffiths, José Miguel Herńandez-Lobato
Chemical Science (2019) Vol. 11, Iss. 2, pp. 577-586
Open Access | Times Cited: 282
Ryan‐Rhys Griffiths, José Miguel Herńandez-Lobato
Chemical Science (2019) Vol. 11, Iss. 2, pp. 577-586
Open Access | Times Cited: 282
Uncertainty Quantification Using Neural Networks for Molecular Property Prediction
Lior Hirschfeld, Kyle Swanson, Kevin Yang, et al.
Journal of Chemical Information and Modeling (2020) Vol. 60, Iss. 8, pp. 3770-3780
Open Access | Times Cited: 221
Lior Hirschfeld, Kyle Swanson, Kevin Yang, et al.
Journal of Chemical Information and Modeling (2020) Vol. 60, Iss. 8, pp. 3770-3780
Open Access | Times Cited: 221
Computational Discovery of Transition-metal Complexes: From High-throughput Screening to Machine Learning
Aditya Nandy, Chenru Duan, Michael G. Taylor, et al.
Chemical Reviews (2021) Vol. 121, Iss. 16, pp. 9927-10000
Closed Access | Times Cited: 218
Aditya Nandy, Chenru Duan, Michael G. Taylor, et al.
Chemical Reviews (2021) Vol. 121, Iss. 16, pp. 9927-10000
Closed Access | Times Cited: 218
GPUMD: A package for constructing accurate machine-learned potentials and performing highly efficient atomistic simulations
Zheyong Fan, Yanzhou Wang, Penghua Ying, et al.
The Journal of Chemical Physics (2022) Vol. 157, Iss. 11
Open Access | Times Cited: 182
Zheyong Fan, Yanzhou Wang, Penghua Ying, et al.
The Journal of Chemical Physics (2022) Vol. 157, Iss. 11
Open Access | Times Cited: 182
Automated in Silico Design of Homogeneous Catalysts
Marco Foscato, Vidar R. Jensen
ACS Catalysis (2020) Vol. 10, Iss. 3, pp. 2354-2377
Open Access | Times Cited: 176
Marco Foscato, Vidar R. Jensen
ACS Catalysis (2020) Vol. 10, Iss. 3, pp. 2354-2377
Open Access | Times Cited: 176
Methods for comparing uncertainty quantifications for material property predictions
Kevin Tran, Willie Neiswanger, Junwoong Yoon, et al.
Machine Learning Science and Technology (2020) Vol. 1, Iss. 2, pp. 025006-025006
Open Access | Times Cited: 155
Kevin Tran, Willie Neiswanger, Junwoong Yoon, et al.
Machine Learning Science and Technology (2020) Vol. 1, Iss. 2, pp. 025006-025006
Open Access | Times Cited: 155
Machine learning dihydrogen activation in the chemical space surrounding Vaska's complex
Pascal Friederich, Gabriel dos Passos Gomes, Riccardo De Bin, et al.
Chemical Science (2020) Vol. 11, Iss. 18, pp. 4584-4601
Open Access | Times Cited: 154
Pascal Friederich, Gabriel dos Passos Gomes, Riccardo De Bin, et al.
Chemical Science (2020) Vol. 11, Iss. 18, pp. 4584-4601
Open Access | Times Cited: 154
Accurate Multiobjective Design in a Space of Millions of Transition Metal Complexes with Neural-Network-Driven Efficient Global Optimization
Jon Paul Janet, Sahasrajit Ramesh, Chenru Duan, et al.
ACS Central Science (2020) Vol. 6, Iss. 4, pp. 513-524
Open Access | Times Cited: 152
Jon Paul Janet, Sahasrajit Ramesh, Chenru Duan, et al.
ACS Central Science (2020) Vol. 6, Iss. 4, pp. 513-524
Open Access | Times Cited: 152
Using Machine Learning and Data Mining to Leverage Community Knowledge for the Engineering of Stable Metal–Organic Frameworks
Aditya Nandy, Chenru Duan, Heather J. Kulik
Journal of the American Chemical Society (2021) Vol. 143, Iss. 42, pp. 17535-17547
Open Access | Times Cited: 148
Aditya Nandy, Chenru Duan, Heather J. Kulik
Journal of the American Chemical Society (2021) Vol. 143, Iss. 42, pp. 17535-17547
Open Access | Times Cited: 148
Evidential Deep Learning for Guided Molecular Property Prediction and Discovery
Ava P. Soleimany, Alexander Amini, Samuel Goldman, et al.
ACS Central Science (2021) Vol. 7, Iss. 8, pp. 1356-1367
Open Access | Times Cited: 145
Ava P. Soleimany, Alexander Amini, Samuel Goldman, et al.
ACS Central Science (2021) Vol. 7, Iss. 8, pp. 1356-1367
Open Access | Times Cited: 145
Roadmap on Machine learning in electronic structure
Heather J. Kulik, Thomas Hammerschmidt, Jonathan Schmidt, et al.
Electronic Structure (2022) Vol. 4, Iss. 2, pp. 023004-023004
Open Access | Times Cited: 138
Heather J. Kulik, Thomas Hammerschmidt, Jonathan Schmidt, et al.
Electronic Structure (2022) Vol. 4, Iss. 2, pp. 023004-023004
Open Access | Times Cited: 138
Ab Initio Machine Learning in Chemical Compound Space
Bing Huang, O. Anatole von Lilienfeld
Chemical Reviews (2021) Vol. 121, Iss. 16, pp. 10001-10036
Open Access | Times Cited: 127
Bing Huang, O. Anatole von Lilienfeld
Chemical Reviews (2021) Vol. 121, Iss. 16, pp. 10001-10036
Open Access | Times Cited: 127
Toward Machine Learning-Enhanced High-Throughput Experimentation
Natalie S. Eyke, Brent A. Koscher, Klavs F. Jensen
Trends in Chemistry (2021) Vol. 3, Iss. 2, pp. 120-132
Open Access | Times Cited: 112
Natalie S. Eyke, Brent A. Koscher, Klavs F. Jensen
Trends in Chemistry (2021) Vol. 3, Iss. 2, pp. 120-132
Open Access | Times Cited: 112
Data‐Driven Materials Innovation and Applications
Zhuo Wang, Zhehao Sun, Hang Yin, et al.
Advanced Materials (2022) Vol. 34, Iss. 36
Closed Access | Times Cited: 108
Zhuo Wang, Zhehao Sun, Hang Yin, et al.
Advanced Materials (2022) Vol. 34, Iss. 36
Closed Access | Times Cited: 108
Machine-learning and high-throughput studies for high-entropy materials
E‐Wen Huang, Wen‐Jay Lee, Sudhanshu S. Singh, et al.
Materials Science and Engineering R Reports (2022) Vol. 147, pp. 100645-100645
Open Access | Times Cited: 93
E‐Wen Huang, Wen‐Jay Lee, Sudhanshu S. Singh, et al.
Materials Science and Engineering R Reports (2022) Vol. 147, pp. 100645-100645
Open Access | Times Cited: 93
Spatially autocorrelated training and validation samples inflate performance assessment of convolutional neural networks
Teja Kattenborn, Felix Schiefer, Julian Frey, et al.
ISPRS Open Journal of Photogrammetry and Remote Sensing (2022) Vol. 5, pp. 100018-100018
Open Access | Times Cited: 83
Teja Kattenborn, Felix Schiefer, Julian Frey, et al.
ISPRS Open Journal of Photogrammetry and Remote Sensing (2022) Vol. 5, pp. 100018-100018
Open Access | Times Cited: 83
MOFSimplify, machine learning models with extracted stability data of three thousand metal–organic frameworks
Aditya Nandy, Gianmarco Terrones, N. Arunachalam, et al.
Scientific Data (2022) Vol. 9, Iss. 1
Open Access | Times Cited: 78
Aditya Nandy, Gianmarco Terrones, N. Arunachalam, et al.
Scientific Data (2022) Vol. 9, Iss. 1
Open Access | Times Cited: 78