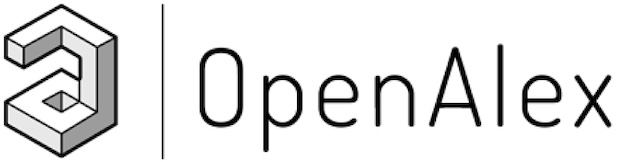
OpenAlex is a bibliographic catalogue of scientific papers, authors and institutions accessible in open access mode, named after the Library of Alexandria. It's citation coverage is excellent and I hope you will find utility in this listing of citing articles!
If you click the article title, you'll navigate to the article, as listed in CrossRef. If you click the Open Access links, you'll navigate to the "best Open Access location". Clicking the citation count will open this listing for that article. Lastly at the bottom of the page, you'll find basic pagination options.
Requested Article:
Highly transferable atomistic machine-learning potentials from curated and compact datasets across the periodic table
Christopher M. Andolina, Wissam A. Saidi
Digital Discovery (2023) Vol. 2, Iss. 4, pp. 1070-1077
Open Access | Times Cited: 7
Christopher M. Andolina, Wissam A. Saidi
Digital Discovery (2023) Vol. 2, Iss. 4, pp. 1070-1077
Open Access | Times Cited: 7
Showing 7 citing articles:
Deep-learning neural network potentials for titanate perovskites
Pandu Wisesa, Terumasa Tadano, Wissam A. Saidi
Computational Materials Science (2025) Vol. 250, pp. 113719-113719
Closed Access
Pandu Wisesa, Terumasa Tadano, Wissam A. Saidi
Computational Materials Science (2025) Vol. 250, pp. 113719-113719
Closed Access
Pairwise force field for magnesium oxide atomistic simulations
Luis Gustavo Vieira Gonçalves, José Pedro Rino
Physica B Condensed Matter (2025) Vol. 702, pp. 416983-416983
Closed Access
Luis Gustavo Vieira Gonçalves, José Pedro Rino
Physica B Condensed Matter (2025) Vol. 702, pp. 416983-416983
Closed Access
Deep Learning-Enhanced Titanium Potential Models for Accurate Molecular Dynamics
You Wu, Haoxiang Zhang, Hanqing Li, et al.
The Journal of Physical Chemistry C (2025)
Closed Access
You Wu, Haoxiang Zhang, Hanqing Li, et al.
The Journal of Physical Chemistry C (2025)
Closed Access
Recent advances in the interface structure prediction for heteromaterial systems
Jili Li, Yefei Li
Journal of Materials Informatics (2023) Vol. 3, Iss. 4
Open Access | Times Cited: 9
Jili Li, Yefei Li
Journal of Materials Informatics (2023) Vol. 3, Iss. 4
Open Access | Times Cited: 9
Active Sparse Bayesian Committee Machine Potential for Isothermal-Isobaric Molecular Dynamics Simulations
Soohaeng Yoo Willow, Dong Geon Kim, R. Sundheep, et al.
Physical Chemistry Chemical Physics (2024) Vol. 26, Iss. 33, pp. 22073-22082
Open Access | Times Cited: 3
Soohaeng Yoo Willow, Dong Geon Kim, R. Sundheep, et al.
Physical Chemistry Chemical Physics (2024) Vol. 26, Iss. 33, pp. 22073-22082
Open Access | Times Cited: 3
ChecMatE: A workflow package to automatically generate machine learning potentials and phase diagrams for semiconductor alloys
Yuxin Guo, Yong‐Bin Zhuang, Jueli Shi, et al.
The Journal of Chemical Physics (2023) Vol. 159, Iss. 9
Closed Access | Times Cited: 6
Yuxin Guo, Yong‐Bin Zhuang, Jueli Shi, et al.
The Journal of Chemical Physics (2023) Vol. 159, Iss. 9
Closed Access | Times Cited: 6
Predicting melting temperatures across the periodic table with machine learning atomistic potentials
Christopher M. Andolina, Wissam A. Saidi
Digital Discovery (2024) Vol. 3, Iss. 7, pp. 1421-1429
Open Access | Times Cited: 1
Christopher M. Andolina, Wissam A. Saidi
Digital Discovery (2024) Vol. 3, Iss. 7, pp. 1421-1429
Open Access | Times Cited: 1
Overcoming Inaccuracies in Machine Learning Interatomic Potential Implementation for Ionic Vacancy Simulations
Pandu Wisesa, Wissam A. Saidi
The Journal of Physical Chemistry Letters (2024), pp. 31-37
Closed Access | Times Cited: 1
Pandu Wisesa, Wissam A. Saidi
The Journal of Physical Chemistry Letters (2024), pp. 31-37
Closed Access | Times Cited: 1