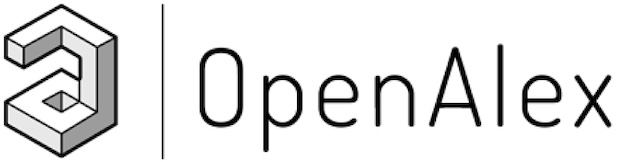
OpenAlex is a bibliographic catalogue of scientific papers, authors and institutions accessible in open access mode, named after the Library of Alexandria. It's citation coverage is excellent and I hope you will find utility in this listing of citing articles!
If you click the article title, you'll navigate to the article, as listed in CrossRef. If you click the Open Access links, you'll navigate to the "best Open Access location". Clicking the citation count will open this listing for that article. Lastly at the bottom of the page, you'll find basic pagination options.
Requested Article:
Predicting oxidation and spin states by high-dimensional neural networks: Applications to lithium manganese oxide spinels
Marco Eckhoff, K. Nikolas Lausch, Peter E. Blöchl, et al.
The Journal of Chemical Physics (2020) Vol. 153, Iss. 16
Open Access | Times Cited: 33
Marco Eckhoff, K. Nikolas Lausch, Peter E. Blöchl, et al.
The Journal of Chemical Physics (2020) Vol. 153, Iss. 16
Open Access | Times Cited: 33
Showing 1-25 of 33 citing articles:
Four Generations of High-Dimensional Neural Network Potentials
Jörg Behler
Chemical Reviews (2021) Vol. 121, Iss. 16, pp. 10037-10072
Closed Access | Times Cited: 604
Jörg Behler
Chemical Reviews (2021) Vol. 121, Iss. 16, pp. 10037-10072
Closed Access | Times Cited: 604
Machine Learning for Electronically Excited States of Molecules
Julia Westermayr, Philipp Marquetand
Chemical Reviews (2020) Vol. 121, Iss. 16, pp. 9873-9926
Open Access | Times Cited: 343
Julia Westermayr, Philipp Marquetand
Chemical Reviews (2020) Vol. 121, Iss. 16, pp. 9873-9926
Open Access | Times Cited: 343
Computational Discovery of Transition-metal Complexes: From High-throughput Screening to Machine Learning
Aditya Nandy, Chenru Duan, Michael G. Taylor, et al.
Chemical Reviews (2021) Vol. 121, Iss. 16, pp. 9927-10000
Closed Access | Times Cited: 218
Aditya Nandy, Chenru Duan, Michael G. Taylor, et al.
Chemical Reviews (2021) Vol. 121, Iss. 16, pp. 9927-10000
Closed Access | Times Cited: 218
How to train a neural network potential
Alea Miako Tokita, Jörg Behler
The Journal of Chemical Physics (2023) Vol. 159, Iss. 12
Open Access | Times Cited: 50
Alea Miako Tokita, Jörg Behler
The Journal of Chemical Physics (2023) Vol. 159, Iss. 12
Open Access | Times Cited: 50
2023 roadmap for potassium-ion batteries
Yang Xu, Maria‐Magdalena Titirici, Jingwei Chen, et al.
Journal of Physics Energy (2023) Vol. 5, Iss. 2, pp. 021502-021502
Open Access | Times Cited: 44
Yang Xu, Maria‐Magdalena Titirici, Jingwei Chen, et al.
Journal of Physics Energy (2023) Vol. 5, Iss. 2, pp. 021502-021502
Open Access | Times Cited: 44
Deep dive into machine learning density functional theory for materials science and chemistry
Lenz Fiedler, Karan Shah, Michael Bußmann, et al.
Physical Review Materials (2022) Vol. 6, Iss. 4
Open Access | Times Cited: 69
Lenz Fiedler, Karan Shah, Michael Bußmann, et al.
Physical Review Materials (2022) Vol. 6, Iss. 4
Open Access | Times Cited: 69
High-dimensional neural network potentials for magnetic systems using spin-dependent atom-centered symmetry functions
Marco Eckhoff, Jörg Behler
npj Computational Materials (2021) Vol. 7, Iss. 1
Open Access | Times Cited: 64
Marco Eckhoff, Jörg Behler
npj Computational Materials (2021) Vol. 7, Iss. 1
Open Access | Times Cited: 64
Lifelong Machine Learning Potentials
Marco Eckhoff, Markus Reiher
Journal of Chemical Theory and Computation (2023) Vol. 19, Iss. 12, pp. 3509-3525
Open Access | Times Cited: 23
Marco Eckhoff, Markus Reiher
Journal of Chemical Theory and Computation (2023) Vol. 19, Iss. 12, pp. 3509-3525
Open Access | Times Cited: 23
Machine Learning Potentials with the Iterative Boltzmann Inversion: Training to Experiment
Sakib Matin, Alice Allen, Justin S. Smith, et al.
Journal of Chemical Theory and Computation (2024) Vol. 20, Iss. 3, pp. 1274-1281
Open Access | Times Cited: 13
Sakib Matin, Alice Allen, Justin S. Smith, et al.
Journal of Chemical Theory and Computation (2024) Vol. 20, Iss. 3, pp. 1274-1281
Open Access | Times Cited: 13
Machine Learning Interatomic Potentials for Catalysis
Deqi Tang, Rangsiman Ketkaew, Sandra Luber
Chemistry - A European Journal (2024) Vol. 30, Iss. 60
Open Access | Times Cited: 9
Deqi Tang, Rangsiman Ketkaew, Sandra Luber
Chemistry - A European Journal (2024) Vol. 30, Iss. 60
Open Access | Times Cited: 9
Accelerated Atomistic Modeling of Solid-State Battery Materials With Machine Learning
Haoyue Guo, Qian Wang, Annika Stuke, et al.
Frontiers in Energy Research (2021) Vol. 9
Open Access | Times Cited: 53
Haoyue Guo, Qian Wang, Annika Stuke, et al.
Frontiers in Energy Research (2021) Vol. 9
Open Access | Times Cited: 53
First-principle-data-integrated machine-learning approach for high-throughput searching of ternary electrocatalyst toward oxygen reduction reaction
Hoje Chun, Eunjik Lee, Kyungju Nam, et al.
Chem Catalysis (2021) Vol. 1, Iss. 4, pp. 855-869
Open Access | Times Cited: 42
Hoje Chun, Eunjik Lee, Kyungju Nam, et al.
Chem Catalysis (2021) Vol. 1, Iss. 4, pp. 855-869
Open Access | Times Cited: 42
Machine Learning for the Discovery, Design, and Engineering of Materials
Chenru Duan, Aditya Nandy, Heather J. Kulik
Annual Review of Chemical and Biomolecular Engineering (2022) Vol. 13, Iss. 1, pp. 405-429
Open Access | Times Cited: 38
Chenru Duan, Aditya Nandy, Heather J. Kulik
Annual Review of Chemical and Biomolecular Engineering (2022) Vol. 13, Iss. 1, pp. 405-429
Open Access | Times Cited: 38
Insights into lithium manganese oxide–water interfaces using machine learning potentials
Marco Eckhoff, Jörg Behler
The Journal of Chemical Physics (2021) Vol. 155, Iss. 24
Open Access | Times Cited: 39
Marco Eckhoff, Jörg Behler
The Journal of Chemical Physics (2021) Vol. 155, Iss. 24
Open Access | Times Cited: 39
Machine-learned interatomic potentials: Recent developments and prospective applications
Volker Eyert, J.L. Wormald, W.A. Curtin, et al.
Journal of materials research/Pratt's guide to venture capital sources (2023) Vol. 38, Iss. 24, pp. 5079-5094
Open Access | Times Cited: 15
Volker Eyert, J.L. Wormald, W.A. Curtin, et al.
Journal of materials research/Pratt's guide to venture capital sources (2023) Vol. 38, Iss. 24, pp. 5079-5094
Open Access | Times Cited: 15
Stability and Equilibrium Structures of Unknown Ternary Metal Oxides Explored by Machine-Learned Potentials
Seungwoo Hwang, Jisu Jung, Chang‐Ho Hong, et al.
Journal of the American Chemical Society (2023) Vol. 145, Iss. 35, pp. 19378-19386
Closed Access | Times Cited: 14
Seungwoo Hwang, Jisu Jung, Chang‐Ho Hong, et al.
Journal of the American Chemical Society (2023) Vol. 145, Iss. 35, pp. 19378-19386
Closed Access | Times Cited: 14
Prognosis and Health Management (PHM) of Solid-State Batteries: Perspectives, Challenges, and Opportunities
Hamed Sadegh Kouhestani, Xiaoping Yi, Guoqing Qi, et al.
Energies (2022) Vol. 15, Iss. 18, pp. 6599-6599
Open Access | Times Cited: 20
Hamed Sadegh Kouhestani, Xiaoping Yi, Guoqing Qi, et al.
Energies (2022) Vol. 15, Iss. 18, pp. 6599-6599
Open Access | Times Cited: 20
Structure and Dynamics of the Magnetite(001)/Water Interface from Molecular Dynamics Simulations Based on a Neural Network Potential
Salvatore Romano, Pablo Montero de Hijes, Matthias Meier, et al.
Journal of Chemical Theory and Computation (2025)
Open Access
Salvatore Romano, Pablo Montero de Hijes, Matthias Meier, et al.
Journal of Chemical Theory and Computation (2025)
Open Access
Phonon dispersion filter: A physics-inspired feature selection for machine learning potentials
Tianyan Xu, Yixuan Xue, Harold S. Park, et al.
Journal of Applied Physics (2025) Vol. 137, Iss. 11
Open Access
Tianyan Xu, Yixuan Xue, Harold S. Park, et al.
Journal of Applied Physics (2025) Vol. 137, Iss. 11
Open Access
Representations and strategies for transferable machine learning improve model performance in chemical discovery
Daniel R. Harper, Aditya Nandy, N. Arunachalam, et al.
The Journal of Chemical Physics (2022) Vol. 156, Iss. 7
Open Access | Times Cited: 12
Daniel R. Harper, Aditya Nandy, N. Arunachalam, et al.
The Journal of Chemical Physics (2022) Vol. 156, Iss. 7
Open Access | Times Cited: 12
Ab initio machine learning of phase space averages
Jan Weinreich, Dominik Lemm, Guido Falk von Rudorff, et al.
The Journal of Chemical Physics (2022) Vol. 157, Iss. 2
Open Access | Times Cited: 12
Jan Weinreich, Dominik Lemm, Guido Falk von Rudorff, et al.
The Journal of Chemical Physics (2022) Vol. 157, Iss. 2
Open Access | Times Cited: 12
CoRe Optimizer: An All-in-One Solution for Machine Learning
Marco Eckhoff, Markus Reiher
Machine Learning Science and Technology (2024)
Open Access | Times Cited: 2
Marco Eckhoff, Markus Reiher
Machine Learning Science and Technology (2024)
Open Access | Times Cited: 2
Machine learning for exploring small polaron configurational space
Viktor C. Birschitzky, Florian Ellinger, Ulrike Diebold, et al.
npj Computational Materials (2022) Vol. 8, Iss. 1
Open Access | Times Cited: 11
Viktor C. Birschitzky, Florian Ellinger, Ulrike Diebold, et al.
npj Computational Materials (2022) Vol. 8, Iss. 1
Open Access | Times Cited: 11
Nonclassical Nucleation of Zinc Oxide from a Physically Motivated Machine-Learning Approach
Jacek Goniakowski, Sarath Menon, Gaétan Laurens, et al.
The Journal of Physical Chemistry C (2022) Vol. 126, Iss. 40, pp. 17456-17469
Open Access | Times Cited: 7
Jacek Goniakowski, Sarath Menon, Gaétan Laurens, et al.
The Journal of Physical Chemistry C (2022) Vol. 126, Iss. 40, pp. 17456-17469
Open Access | Times Cited: 7
First-principles materials simulation and design for alkali and alkaline metal ion batteries accelerated by machine learning
Lujie Jin, Yujin Ji, Hongshuai Wang, et al.
Physical Chemistry Chemical Physics (2021) Vol. 23, Iss. 38, pp. 21470-21483
Closed Access | Times Cited: 7
Lujie Jin, Yujin Ji, Hongshuai Wang, et al.
Physical Chemistry Chemical Physics (2021) Vol. 23, Iss. 38, pp. 21470-21483
Closed Access | Times Cited: 7