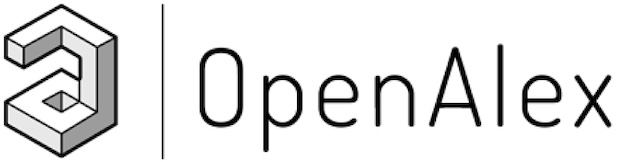
OpenAlex is a bibliographic catalogue of scientific papers, authors and institutions accessible in open access mode, named after the Library of Alexandria. It's citation coverage is excellent and I hope you will find utility in this listing of citing articles!
If you click the article title, you'll navigate to the article, as listed in CrossRef. If you click the Open Access links, you'll navigate to the "best Open Access location". Clicking the citation count will open this listing for that article. Lastly at the bottom of the page, you'll find basic pagination options.
Requested Article:
Neural network-based fuel consumption estimation for container ships in Korea
Luan Thanh Le, Gunwoo Lee, Keun-Sik Park, et al.
Maritime Policy & Management (2020) Vol. 47, Iss. 5, pp. 615-632
Closed Access | Times Cited: 75
Luan Thanh Le, Gunwoo Lee, Keun-Sik Park, et al.
Maritime Policy & Management (2020) Vol. 47, Iss. 5, pp. 615-632
Closed Access | Times Cited: 75
Showing 1-25 of 75 citing articles:
A Review of Further Directions for Artificial Intelligence, Machine Learning, and Deep Learning in Smart Logistics
Manuel Woschank, Erwin Rauch, Helmut Zsifkovits
Sustainability (2020) Vol. 12, Iss. 9, pp. 3760-3760
Open Access | Times Cited: 243
Manuel Woschank, Erwin Rauch, Helmut Zsifkovits
Sustainability (2020) Vol. 12, Iss. 9, pp. 3760-3760
Open Access | Times Cited: 243
Review of current regulations, available technologies, and future trends in the green shipping industry
Mina Tadros, L. Ventura, C. Guedes Soares
Ocean Engineering (2023) Vol. 280, pp. 114670-114670
Open Access | Times Cited: 86
Mina Tadros, L. Ventura, C. Guedes Soares
Ocean Engineering (2023) Vol. 280, pp. 114670-114670
Open Access | Times Cited: 86
Harnessing the power of Machine learning for AIS Data-Driven maritime Research: A comprehensive review
Ying Yang, Yang Liu, Guorong Li, et al.
Transportation Research Part E Logistics and Transportation Review (2024) Vol. 183, pp. 103426-103426
Open Access | Times Cited: 36
Ying Yang, Yang Liu, Guorong Li, et al.
Transportation Research Part E Logistics and Transportation Review (2024) Vol. 183, pp. 103426-103426
Open Access | Times Cited: 36
Data analytics for fuel consumption management in maritime transportation: Status and perspectives
Ran Yan, Shuaian Wang, Harilaos N. Psaraftis
Transportation Research Part E Logistics and Transportation Review (2021) Vol. 155, pp. 102489-102489
Open Access | Times Cited: 99
Ran Yan, Shuaian Wang, Harilaos N. Psaraftis
Transportation Research Part E Logistics and Transportation Review (2021) Vol. 155, pp. 102489-102489
Open Access | Times Cited: 99
Emerging approaches applied to maritime transport research: Past and future
Ran Yan, Shuaian Wang, Lu Zhen, et al.
Communications in Transportation Research (2021) Vol. 1, pp. 100011-100011
Open Access | Times Cited: 84
Ran Yan, Shuaian Wang, Lu Zhen, et al.
Communications in Transportation Research (2021) Vol. 1, pp. 100011-100011
Open Access | Times Cited: 84
Development of condition-based maintenance strategy for fault diagnosis for ship engine systems
Çağlar Karatuğ, Yasin Arslanoğlu
Ocean Engineering (2022) Vol. 256, pp. 111515-111515
Closed Access | Times Cited: 57
Çağlar Karatuğ, Yasin Arslanoğlu
Ocean Engineering (2022) Vol. 256, pp. 111515-111515
Closed Access | Times Cited: 57
A comprehensive review on the prediction of ship energy consumption and pollution gas emissions
Kai Wang, Jianhang Wang, Lianzhong Huang, et al.
Ocean Engineering (2022) Vol. 266, pp. 112826-112826
Open Access | Times Cited: 47
Kai Wang, Jianhang Wang, Lianzhong Huang, et al.
Ocean Engineering (2022) Vol. 266, pp. 112826-112826
Open Access | Times Cited: 47
Prediction of harbour vessel fuel consumption based on machine learning approach
Zhong Shuo Chen, Jasmine Siu Lee Lam, Zengqi Xiao
Ocean Engineering (2023) Vol. 278, pp. 114483-114483
Closed Access | Times Cited: 38
Zhong Shuo Chen, Jasmine Siu Lee Lam, Zengqi Xiao
Ocean Engineering (2023) Vol. 278, pp. 114483-114483
Closed Access | Times Cited: 38
Discrete multi-objective artificial bee colony algorithm for green co-scheduling problem of ship lift and ship lock
Qianqian Zheng, Yu Zhang, Lijun He, et al.
Advanced Engineering Informatics (2023) Vol. 55, pp. 101897-101897
Closed Access | Times Cited: 29
Qianqian Zheng, Yu Zhang, Lijun He, et al.
Advanced Engineering Informatics (2023) Vol. 55, pp. 101897-101897
Closed Access | Times Cited: 29
Using Artificial Neural Networks for Predicting Ship Fuel Consumption
Van Giao Nguyen, R. Sakthivel, Krzysztof Rudzki, et al.
Polish Maritime Research (2023) Vol. 30, Iss. 2, pp. 39-60
Open Access | Times Cited: 28
Van Giao Nguyen, R. Sakthivel, Krzysztof Rudzki, et al.
Polish Maritime Research (2023) Vol. 30, Iss. 2, pp. 39-60
Open Access | Times Cited: 28
Comparative study of machine learning techniques to predict fuel consumption of a marine diesel engine
Onur Yüksel, Murat Bayraktar, Mustafa Sokukcu
Ocean Engineering (2023) Vol. 286, pp. 115505-115505
Closed Access | Times Cited: 25
Onur Yüksel, Murat Bayraktar, Mustafa Sokukcu
Ocean Engineering (2023) Vol. 286, pp. 115505-115505
Closed Access | Times Cited: 25
Improving ship energy efficiency: Models, methods, and applications
Ran Yan, Dong Yang, Tianyu Wang, et al.
Applied Energy (2024) Vol. 368, pp. 123132-123132
Closed Access | Times Cited: 16
Ran Yan, Dong Yang, Tianyu Wang, et al.
Applied Energy (2024) Vol. 368, pp. 123132-123132
Closed Access | Times Cited: 16
Prediction of harbour vessel emissions based on machine learning approach
Zhong Shuo Chen, Jasmine Siu Lee Lam, Zengqi Xiao
Transportation Research Part D Transport and Environment (2024) Vol. 131, pp. 104214-104214
Open Access | Times Cited: 12
Zhong Shuo Chen, Jasmine Siu Lee Lam, Zengqi Xiao
Transportation Research Part D Transport and Environment (2024) Vol. 131, pp. 104214-104214
Open Access | Times Cited: 12
Fuel consumption cost prediction model for ro-ro carriers: a machine learning-based application
Miao Su, Hee-Jeong Lee, Xueqin Wang, et al.
Maritime Policy & Management (2024), pp. 1-21
Closed Access | Times Cited: 11
Miao Su, Hee-Jeong Lee, Xueqin Wang, et al.
Maritime Policy & Management (2024), pp. 1-21
Closed Access | Times Cited: 11
Accuracy and applicability of ship’s fuel consumption prediction models: A comprehensive comparative analysis
Xi Luo, Ran Yan, Lang Xu, et al.
Energy (2024), pp. 133187-133187
Closed Access | Times Cited: 11
Xi Luo, Ran Yan, Lang Xu, et al.
Energy (2024), pp. 133187-133187
Closed Access | Times Cited: 11
The Development of a Machine Learning-Based Carbon Emission Prediction Method for a Multi-Fuel-Propelled Smart Ship by Using Onboard Measurement Data
J. H. Lee, Jeong-On Eom, Jumi Park, et al.
Sustainability (2024) Vol. 16, Iss. 6, pp. 2381-2381
Open Access | Times Cited: 10
J. H. Lee, Jeong-On Eom, Jumi Park, et al.
Sustainability (2024) Vol. 16, Iss. 6, pp. 2381-2381
Open Access | Times Cited: 10
Use of Artificial Neural Networks to Predict Fuel Consumption on the Basis of Technical Parameters of Vehicles
Jarosław Ziółkowski, Mateusz Oszczypała, Jerzy Małąchowski, et al.
Energies (2021) Vol. 14, Iss. 9, pp. 2639-2639
Open Access | Times Cited: 56
Jarosław Ziółkowski, Mateusz Oszczypała, Jerzy Małąchowski, et al.
Energies (2021) Vol. 14, Iss. 9, pp. 2639-2639
Open Access | Times Cited: 56
An adaptive hyper parameter tuning model for ship fuel consumption prediction under complex maritime environments
Tianrui Zhou, Qinyou Hu, Zhihui Hu, et al.
Journal of Ocean Engineering and Science (2021) Vol. 7, Iss. 3, pp. 255-263
Open Access | Times Cited: 51
Tianrui Zhou, Qinyou Hu, Zhihui Hu, et al.
Journal of Ocean Engineering and Science (2021) Vol. 7, Iss. 3, pp. 255-263
Open Access | Times Cited: 51
Strategy for ship energy efficiency based on optimization model and data-driven approach
Çağlar Karatuğ, Mina Tadros, L. Ventura, et al.
Ocean Engineering (2023) Vol. 279, pp. 114397-114397
Open Access | Times Cited: 21
Çağlar Karatuğ, Mina Tadros, L. Ventura, et al.
Ocean Engineering (2023) Vol. 279, pp. 114397-114397
Open Access | Times Cited: 21
Predicting PM10 and PM2.5 concentration in container ports: A deep learning approach
So-Young Park, Su‐Han Woo, Changwon Lim
Transportation Research Part D Transport and Environment (2023) Vol. 115, pp. 103601-103601
Closed Access | Times Cited: 20
So-Young Park, Su‐Han Woo, Changwon Lim
Transportation Research Part D Transport and Environment (2023) Vol. 115, pp. 103601-103601
Closed Access | Times Cited: 20
Measurement-based relationships between container ship operating parameters and fuel consumption
Tomasz Cepowski, Andrzej Drozd
Applied Energy (2023) Vol. 347, pp. 121315-121315
Open Access | Times Cited: 19
Tomasz Cepowski, Andrzej Drozd
Applied Energy (2023) Vol. 347, pp. 121315-121315
Open Access | Times Cited: 19
Innovative approaches to addressing the tradeoff between interpretability and accuracy in ship fuel consumption prediction
Haoqing Wang, Ran Yan, Shuaian Wang, et al.
Transportation Research Part C Emerging Technologies (2023) Vol. 157, pp. 104361-104361
Closed Access | Times Cited: 19
Haoqing Wang, Ran Yan, Shuaian Wang, et al.
Transportation Research Part C Emerging Technologies (2023) Vol. 157, pp. 104361-104361
Closed Access | Times Cited: 19
A novel high-precision and self-adaptive prediction method for ship energy consumption based on the multi-model fusion approach
Kai Wang, Xing Liu, Xin Guo, et al.
Energy (2024), pp. 133265-133265
Closed Access | Times Cited: 6
Kai Wang, Xing Liu, Xin Guo, et al.
Energy (2024), pp. 133265-133265
Closed Access | Times Cited: 6
Ship fuel consumption prediction based on transfer learning: Models and applications
Xi Luo, Mingyang Zhang, Yi Han, et al.
Engineering Applications of Artificial Intelligence (2024) Vol. 141, pp. 109769-109769
Closed Access | Times Cited: 5
Xi Luo, Mingyang Zhang, Yi Han, et al.
Engineering Applications of Artificial Intelligence (2024) Vol. 141, pp. 109769-109769
Closed Access | Times Cited: 5
Predicting VLCC fuel consumption with machine learning using operationally available sensor data
Christos N. Papandreou, Απόστολος Ζιακόπουλος
Ocean Engineering (2021) Vol. 243, pp. 110321-110321
Closed Access | Times Cited: 32
Christos N. Papandreou, Απόστολος Ζιακόπουλος
Ocean Engineering (2021) Vol. 243, pp. 110321-110321
Closed Access | Times Cited: 32