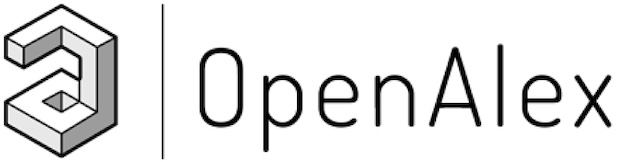
OpenAlex is a bibliographic catalogue of scientific papers, authors and institutions accessible in open access mode, named after the Library of Alexandria. It's citation coverage is excellent and I hope you will find utility in this listing of citing articles!
If you click the article title, you'll navigate to the article, as listed in CrossRef. If you click the Open Access links, you'll navigate to the "best Open Access location". Clicking the citation count will open this listing for that article. Lastly at the bottom of the page, you'll find basic pagination options.
Requested Article:
Uncertainty quantification by direct propagation of shallow ensembles
Matthias Kellner, Michele Ceriotti
Machine Learning Science and Technology (2024) Vol. 5, Iss. 3, pp. 035006-035006
Open Access | Times Cited: 9
Matthias Kellner, Michele Ceriotti
Machine Learning Science and Technology (2024) Vol. 5, Iss. 3, pp. 035006-035006
Open Access | Times Cited: 9
Showing 9 citing articles:
i-PI 3.0: A flexible and efficient framework for advanced atomistic simulations
Yair Litman, Venkat Kapil, Yotam M. Y. Feldman, et al.
The Journal of Chemical Physics (2024) Vol. 161, Iss. 6
Open Access | Times Cited: 8
Yair Litman, Venkat Kapil, Yotam M. Y. Feldman, et al.
The Journal of Chemical Physics (2024) Vol. 161, Iss. 6
Open Access | Times Cited: 8
Exploring parameter dependence of atomic minima with implicit differentiation
Ivan Maliyov, Petr Grigorev, Thomas D. Swinburne
npj Computational Materials (2025) Vol. 11, Iss. 1
Open Access | Times Cited: 1
Ivan Maliyov, Petr Grigorev, Thomas D. Swinburne
npj Computational Materials (2025) Vol. 11, Iss. 1
Open Access | Times Cited: 1
FeNNol: An efficient and flexible library for building force-field-enhanced neural network potentials
Thomas Plé, Olivier Adjoua, Louis Lagardère, et al.
The Journal of Chemical Physics (2024) Vol. 161, Iss. 4
Open Access | Times Cited: 4
Thomas Plé, Olivier Adjoua, Louis Lagardère, et al.
The Journal of Chemical Physics (2024) Vol. 161, Iss. 4
Open Access | Times Cited: 4
Enhanced sampling of robust molecular datasets with uncertainty-based collective variables
Aik Rui Tan, Johannes C. B. Dietschreit, Rafael Gómez‐Bombarelli
The Journal of Chemical Physics (2025) Vol. 162, Iss. 3
Open Access
Aik Rui Tan, Johannes C. B. Dietschreit, Rafael Gómez‐Bombarelli
The Journal of Chemical Physics (2025) Vol. 162, Iss. 3
Open Access
A review of deep learning for super-resolution in fluid flows
Filippos Sofos, Dimitris Drikakis
Physics of Fluids (2025) Vol. 37, Iss. 4
Closed Access
Filippos Sofos, Dimitris Drikakis
Physics of Fluids (2025) Vol. 37, Iss. 4
Closed Access
Spatially Resolved Uncertainties for Machine Learning Potentials
Esther Heid, Johannes Schörghuber, Ralf Wanzenböck, et al.
Journal of Chemical Information and Modeling (2024) Vol. 64, Iss. 16, pp. 6377-6387
Open Access | Times Cited: 3
Esther Heid, Johannes Schörghuber, Ralf Wanzenböck, et al.
Journal of Chemical Information and Modeling (2024) Vol. 64, Iss. 16, pp. 6377-6387
Open Access | Times Cited: 3
Parameter uncertainties for imperfect surrogate models in the low-noise regime
Thomas D. Swinburne, Danny Pérez
Machine Learning Science and Technology (2024) Vol. 6, Iss. 1, pp. 015008-015008
Open Access | Times Cited: 2
Thomas D. Swinburne, Danny Pérez
Machine Learning Science and Technology (2024) Vol. 6, Iss. 1, pp. 015008-015008
Open Access | Times Cited: 2
Spatially resolved uncertainties for machine learning potentials
Esther Heid, Johannes Schörghuber, Ralf Wanzenböck, et al.
(2024)
Open Access | Times Cited: 1
Esther Heid, Johannes Schörghuber, Ralf Wanzenböck, et al.
(2024)
Open Access | Times Cited: 1
Spatially resolved uncertainties for machine learning potentials
Esther Heid, Johannes Schörghuber, Ralf Wanzenböck, et al.
(2024)
Open Access | Times Cited: 1
Esther Heid, Johannes Schörghuber, Ralf Wanzenböck, et al.
(2024)
Open Access | Times Cited: 1