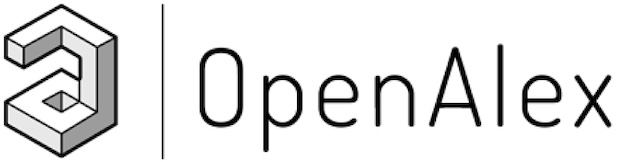
OpenAlex is a bibliographic catalogue of scientific papers, authors and institutions accessible in open access mode, named after the Library of Alexandria. It's citation coverage is excellent and I hope you will find utility in this listing of citing articles!
If you click the article title, you'll navigate to the article, as listed in CrossRef. If you click the Open Access links, you'll navigate to the "best Open Access location". Clicking the citation count will open this listing for that article. Lastly at the bottom of the page, you'll find basic pagination options.
Requested Article:
Quokka: a comprehensive tool for rapid and accurate prediction of kinase family-specific phosphorylation sites in the human proteome
Fuyi Li, Chen Li, Tatiana T. Marquez‐Lago, et al.
Bioinformatics (2018) Vol. 34, Iss. 24, pp. 4223-4231
Open Access | Times Cited: 159
Fuyi Li, Chen Li, Tatiana T. Marquez‐Lago, et al.
Bioinformatics (2018) Vol. 34, Iss. 24, pp. 4223-4231
Open Access | Times Cited: 159
Showing 1-25 of 159 citing articles:
iLearn: an integrated platform and meta-learner for feature engineering, machine-learning analysis and modeling of DNA, RNA and protein sequence data
Zhen Chen, Pei Zhao, Fuyi Li, et al.
Briefings in Bioinformatics (2019) Vol. 21, Iss. 3, pp. 1047-1057
Closed Access | Times Cited: 372
Zhen Chen, Pei Zhao, Fuyi Li, et al.
Briefings in Bioinformatics (2019) Vol. 21, Iss. 3, pp. 1047-1057
Closed Access | Times Cited: 372
Machine intelligence in peptide therapeutics: A next‐generation tool for rapid disease screening
Shaherin Basith, Balachandran Manavalan, Tae Hwan Shin, et al.
Medicinal Research Reviews (2020) Vol. 40, Iss. 4, pp. 1276-1314
Closed Access | Times Cited: 256
Shaherin Basith, Balachandran Manavalan, Tae Hwan Shin, et al.
Medicinal Research Reviews (2020) Vol. 40, Iss. 4, pp. 1276-1314
Closed Access | Times Cited: 256
iTerm-PseKNC: a sequence-based tool for predicting bacterial transcriptional terminators
Chao-Qin Feng, Zhao‐Yue Zhang, Xiaojuan Zhu, et al.
Bioinformatics (2018) Vol. 35, Iss. 9, pp. 1469-1477
Closed Access | Times Cited: 206
Chao-Qin Feng, Zhao‐Yue Zhang, Xiaojuan Zhu, et al.
Bioinformatics (2018) Vol. 35, Iss. 9, pp. 1469-1477
Closed Access | Times Cited: 206
i6mA-Pred: identifying DNA N6-methyladenine sites in the rice genome
Wei Chen, Hao Lv, Fulei Nie, et al.
Bioinformatics (2019) Vol. 35, Iss. 16, pp. 2796-2800
Closed Access | Times Cited: 196
Wei Chen, Hao Lv, Fulei Nie, et al.
Bioinformatics (2019) Vol. 35, Iss. 16, pp. 2796-2800
Closed Access | Times Cited: 196
iRNA(m6A)-PseDNC: Identifying N6-methyladenosine sites using pseudo dinucleotide composition
Wei Chen, Hui Ding, Xu Zhou, et al.
Analytical Biochemistry (2018) Vol. 561-562, pp. 59-65
Closed Access | Times Cited: 187
Wei Chen, Hui Ding, Xu Zhou, et al.
Analytical Biochemistry (2018) Vol. 561-562, pp. 59-65
Closed Access | Times Cited: 187
Identify origin of replication inSaccharomyces cerevisiaeusing two-step feature selection technique
Fanny Dao, Hao Lv, Fang Wang, et al.
Bioinformatics (2018) Vol. 35, Iss. 12, pp. 2075-2083
Closed Access | Times Cited: 180
Fanny Dao, Hao Lv, Fang Wang, et al.
Bioinformatics (2018) Vol. 35, Iss. 12, pp. 2075-2083
Closed Access | Times Cited: 180
Exploring sequence-based features for the improved prediction of DNA N4-methylcytosine sites in multiple species
Leyi Wei, Shasha Luan, Luís Augusto Eijy Nagai, et al.
Bioinformatics (2018) Vol. 35, Iss. 8, pp. 1326-1333
Closed Access | Times Cited: 169
Leyi Wei, Shasha Luan, Luís Augusto Eijy Nagai, et al.
Bioinformatics (2018) Vol. 35, Iss. 8, pp. 1326-1333
Closed Access | Times Cited: 169
DeepPhos: prediction of protein phosphorylation sites with deep learning
Fenglin Luo, Minghui Wang, Yu Liu, et al.
Bioinformatics (2018) Vol. 35, Iss. 16, pp. 2766-2773
Open Access | Times Cited: 168
Fenglin Luo, Minghui Wang, Yu Liu, et al.
Bioinformatics (2018) Vol. 35, Iss. 16, pp. 2766-2773
Open Access | Times Cited: 168
A comprehensive review and performance evaluation of bioinformatics tools for HLA class I peptide-binding prediction
Shutao Mei, Fuyi Li, André Leier, et al.
Briefings in Bioinformatics (2019) Vol. 21, Iss. 4, pp. 1119-1135
Open Access | Times Cited: 155
Shutao Mei, Fuyi Li, André Leier, et al.
Briefings in Bioinformatics (2019) Vol. 21, Iss. 4, pp. 1119-1135
Open Access | Times Cited: 155
An Interpretable Prediction Model for Identifying N7-Methylguanosine Sites Based on XGBoost and SHAP
Yue Bi, Dongxu Xiang, Zongyuan Ge, et al.
Molecular Therapy — Nucleic Acids (2020) Vol. 22, pp. 362-372
Open Access | Times Cited: 141
Yue Bi, Dongxu Xiang, Zongyuan Ge, et al.
Molecular Therapy — Nucleic Acids (2020) Vol. 22, pp. 362-372
Open Access | Times Cited: 141
Identification of protein subcellular localization via integrating evolutionary and physicochemical information into Chou’s general PseAAC
Yinan Shen, Jijun Tang, Fei Guo
Journal of Theoretical Biology (2018) Vol. 462, pp. 230-239
Closed Access | Times Cited: 153
Yinan Shen, Jijun Tang, Fei Guo
Journal of Theoretical Biology (2018) Vol. 462, pp. 230-239
Closed Access | Times Cited: 153
iEnhancer-5Step: Identifying enhancers using hidden information of DNA sequences via Chou's 5-step rule and word embedding
Nguyen Quoc Khanh Le, Edward Kien Yee Yapp, Quang‐Thai Ho, et al.
Analytical Biochemistry (2019) Vol. 571, pp. 53-61
Closed Access | Times Cited: 141
Nguyen Quoc Khanh Le, Edward Kien Yee Yapp, Quang‐Thai Ho, et al.
Analytical Biochemistry (2019) Vol. 571, pp. 53-61
Closed Access | Times Cited: 141
SCRIBER: accurate and partner type-specific prediction of protein-binding residues from proteins sequences
Jian Zhang, Lukasz Kurgan
Bioinformatics (2019) Vol. 35, Iss. 14, pp. i343-i353
Open Access | Times Cited: 129
Jian Zhang, Lukasz Kurgan
Bioinformatics (2019) Vol. 35, Iss. 14, pp. i343-i353
Open Access | Times Cited: 129
Large-scale comparative assessment of computational predictors for lysine post-translational modification sites
Zhen Chen, Xuhan Liu, Fuyi Li, et al.
Briefings in Bioinformatics (2018) Vol. 20, Iss. 6, pp. 2267-2290
Open Access | Times Cited: 126
Zhen Chen, Xuhan Liu, Fuyi Li, et al.
Briefings in Bioinformatics (2018) Vol. 20, Iss. 6, pp. 2267-2290
Open Access | Times Cited: 126
SPalmitoylC-PseAAC: A sequence-based model developed via Chou's 5-steps rule and general PseAAC for identifying S-palmitoylation sites in proteins
Waqar Hussain, Yaser Daanial Khan, Nouman Rasool, et al.
Analytical Biochemistry (2018) Vol. 568, pp. 14-23
Closed Access | Times Cited: 122
Waqar Hussain, Yaser Daanial Khan, Nouman Rasool, et al.
Analytical Biochemistry (2018) Vol. 568, pp. 14-23
Closed Access | Times Cited: 122
MULTiPly: a novel multi-layer predictor for discovering general and specific types of promoters
Meng Zhang, Fuyi Li, Tatiana T. Marquez‐Lago, et al.
Bioinformatics (2019) Vol. 35, Iss. 17, pp. 2957-2965
Open Access | Times Cited: 120
Meng Zhang, Fuyi Li, Tatiana T. Marquez‐Lago, et al.
Bioinformatics (2019) Vol. 35, Iss. 17, pp. 2957-2965
Open Access | Times Cited: 120
DeepTorrent: a deep learning-based approach for predicting DNA N4-methylcytosine sites
Quanzhong Liu, Jin-Xiang Chen, Yanze Wang, et al.
Briefings in Bioinformatics (2020) Vol. 22, Iss. 3
Open Access | Times Cited: 114
Quanzhong Liu, Jin-Xiang Chen, Yanze Wang, et al.
Briefings in Bioinformatics (2020) Vol. 22, Iss. 3
Open Access | Times Cited: 114
DeepCleave: a deep learning predictor for caspase and matrix metalloprotease substrates and cleavage sites
Fuyi Li, Jin-Xiang Chen, André Leier, et al.
Bioinformatics (2019) Vol. 36, Iss. 4, pp. 1057-1065
Open Access | Times Cited: 107
Fuyi Li, Jin-Xiang Chen, André Leier, et al.
Bioinformatics (2019) Vol. 36, Iss. 4, pp. 1057-1065
Open Access | Times Cited: 107
iPPI-PseAAC(CGR): Identify protein-protein interactions by incorporating chaos game representation into PseAAC
Jianhua Jia, Xiaoyan Li, Wang‐Ren Qiu, et al.
Journal of Theoretical Biology (2018) Vol. 460, pp. 195-203
Closed Access | Times Cited: 105
Jianhua Jia, Xiaoyan Li, Wang‐Ren Qiu, et al.
Journal of Theoretical Biology (2018) Vol. 460, pp. 195-203
Closed Access | Times Cited: 105
iRNA-PseKNC(2methyl): Identify RNA 2'-O-methylation sites by convolution neural network and Chou's pseudo components
Muhammad Tahir, Hilal Tayara, Kil To Chong
Journal of Theoretical Biology (2018) Vol. 465, pp. 1-6
Open Access | Times Cited: 101
Muhammad Tahir, Hilal Tayara, Kil To Chong
Journal of Theoretical Biology (2018) Vol. 465, pp. 1-6
Open Access | Times Cited: 101
Procleave: Predicting Protease-Specific Substrate Cleavage Sites by Combining Sequence and Structural Information
Fuyi Li, André Leier, Quanzhong Liu, et al.
Genomics Proteomics & Bioinformatics (2020) Vol. 18, Iss. 1, pp. 52-64
Open Access | Times Cited: 99
Fuyi Li, André Leier, Quanzhong Liu, et al.
Genomics Proteomics & Bioinformatics (2020) Vol. 18, Iss. 1, pp. 52-64
Open Access | Times Cited: 99
iN6-Methyl (5-step): Identifying RNA N6-methyladenosine sites using deep learning mode via Chou's 5-step rules and Chou's general PseKNC
Iman Nazari, Muhammad Tahir, Hilal Tayara, et al.
Chemometrics and Intelligent Laboratory Systems (2019) Vol. 193, pp. 103811-103811
Closed Access | Times Cited: 97
Iman Nazari, Muhammad Tahir, Hilal Tayara, et al.
Chemometrics and Intelligent Laboratory Systems (2019) Vol. 193, pp. 103811-103811
Closed Access | Times Cited: 97
iPhosH-PseAAC: Identify Phosphohistidine Sites in Proteins by Blending Statistical Moments and Position Relative Features According to the Chou's 5-Step Rule and General Pseudo Amino Acid Composition
Muhammad Awais, Waqar Hussain, Yaser Daanial Khan, et al.
IEEE/ACM Transactions on Computational Biology and Bioinformatics (2019) Vol. 18, Iss. 2, pp. 596-610
Closed Access | Times Cited: 95
Muhammad Awais, Waqar Hussain, Yaser Daanial Khan, et al.
IEEE/ACM Transactions on Computational Biology and Bioinformatics (2019) Vol. 18, Iss. 2, pp. 596-610
Closed Access | Times Cited: 95
pLoc_bal-mHum: Predict subcellular localization of human proteins by PseAAC and quasi-balancing training dataset
Kuo‐Chen Chou, Xiang Cheng, Xuan Xiao
Genomics (2018) Vol. 111, Iss. 6, pp. 1274-1282
Open Access | Times Cited: 92
Kuo‐Chen Chou, Xiang Cheng, Xuan Xiao
Genomics (2018) Vol. 111, Iss. 6, pp. 1274-1282
Open Access | Times Cited: 92
Twenty years of bioinformatics research for protease-specific substrate and cleavage site prediction: a comprehensive revisit and benchmarking of existing methods
Fuyi Li, Yanan Wang, Chen Li, et al.
Briefings in Bioinformatics (2018) Vol. 20, Iss. 6, pp. 2150-2166
Open Access | Times Cited: 92
Fuyi Li, Yanan Wang, Chen Li, et al.
Briefings in Bioinformatics (2018) Vol. 20, Iss. 6, pp. 2150-2166
Open Access | Times Cited: 92