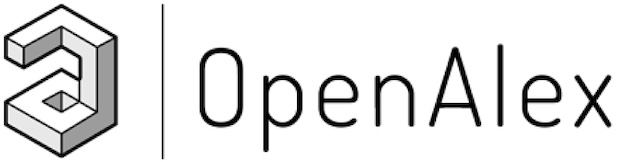
OpenAlex is a bibliographic catalogue of scientific papers, authors and institutions accessible in open access mode, named after the Library of Alexandria. It's citation coverage is excellent and I hope you will find utility in this listing of citing articles!
If you click the article title, you'll navigate to the article, as listed in CrossRef. If you click the Open Access links, you'll navigate to the "best Open Access location". Clicking the citation count will open this listing for that article. Lastly at the bottom of the page, you'll find basic pagination options.
Requested Article:
Comparative Analysis of Gradient-Boosting Ensembles for Estimation of Compressive Strength of Quaternary Blend Concrete
Ismail B. Mustapha, Muyideen Abdulkareem, Taha M. Jassam, et al.
International Journal of Concrete Structures and Materials (2024) Vol. 18, Iss. 1
Open Access | Times Cited: 12
Ismail B. Mustapha, Muyideen Abdulkareem, Taha M. Jassam, et al.
International Journal of Concrete Structures and Materials (2024) Vol. 18, Iss. 1
Open Access | Times Cited: 12
Showing 12 citing articles:
Prediction of wastewater treatment plant performance through machine learning techniques
Hani Mahanna, Nora El-Rashidy, Mosbeh R. Kaloop, et al.
Desalination and Water Treatment (2024) Vol. 319, pp. 100524-100524
Open Access | Times Cited: 11
Hani Mahanna, Nora El-Rashidy, Mosbeh R. Kaloop, et al.
Desalination and Water Treatment (2024) Vol. 319, pp. 100524-100524
Open Access | Times Cited: 11
Machine Learning for predicting strength properties of waste iron slag concrete
Matiur Rahman Raju, Syed Ishtiaq Ahmad, Md Mehedi Hasan, et al.
Heliyon (2025) Vol. 11, Iss. 3, pp. e42133-e42133
Open Access | Times Cited: 1
Matiur Rahman Raju, Syed Ishtiaq Ahmad, Md Mehedi Hasan, et al.
Heliyon (2025) Vol. 11, Iss. 3, pp. e42133-e42133
Open Access | Times Cited: 1
Predicting compressive strength of hollow concrete prisms using machine learning techniques and explainable artificial intelligence (XAI)
Waleed Bin Inqiad, Elena Valentina Dumitrascu, Robert Alexandru Dobre, et al.
Heliyon (2024) Vol. 10, Iss. 17, pp. e36841-e36841
Open Access | Times Cited: 8
Waleed Bin Inqiad, Elena Valentina Dumitrascu, Robert Alexandru Dobre, et al.
Heliyon (2024) Vol. 10, Iss. 17, pp. e36841-e36841
Open Access | Times Cited: 8
Proposing Optimized Random Forest Models for Predicting Compressive Strength of Geopolymer Composites
Bin Feng, Shahab Hosseini, Jie Chen, et al.
Infrastructures (2024) Vol. 9, Iss. 10, pp. 181-181
Open Access | Times Cited: 8
Bin Feng, Shahab Hosseini, Jie Chen, et al.
Infrastructures (2024) Vol. 9, Iss. 10, pp. 181-181
Open Access | Times Cited: 8
Explainable Artificial Intelligence for Predicting the Compressive Strength of Soil and Ground Granulated Blast Furnace Slag Mixtures
Ahmed Mohammed Awad Mohammed, Omayma Husain, Muyideen Abdulkareem, et al.
Results in Engineering (2024) Vol. 25, pp. 103637-103637
Open Access | Times Cited: 4
Ahmed Mohammed Awad Mohammed, Omayma Husain, Muyideen Abdulkareem, et al.
Results in Engineering (2024) Vol. 25, pp. 103637-103637
Open Access | Times Cited: 4
An Explainable Machine Learning (XML) approach to determine strength of glass powder concrete
Wali Ullah Khan, Waleed Bin Inqiad, Bilal Ayub, et al.
Materials Today Communications (2025), pp. 112181-112181
Closed Access
Wali Ullah Khan, Waleed Bin Inqiad, Bilal Ayub, et al.
Materials Today Communications (2025), pp. 112181-112181
Closed Access
PREDICTION OF THE COMPRESSIVE AND TENSILE STRENGTH OF HIGH-PERFORMANCE CONCRETE BASED ON A HYBRID MODEL OF MULTILAYER PERCEPTRON (MLP) AND LIGHTGBM
S. J. Zhao
Ceramics - Silikaty (2025)
Open Access
S. J. Zhao
Ceramics - Silikaty (2025)
Open Access
Interpretable Machine-Learning Models to Predict the Flexural Strength of Fiber-Reinforced SCM-Blended Concrete Composites
Saad Shamim Ansari, Syed Muhammad Ibrahim, Syed Danish Hasan
Journal of structural design and construction practice. (2024) Vol. 30, Iss. 2
Closed Access | Times Cited: 2
Saad Shamim Ansari, Syed Muhammad Ibrahim, Syed Danish Hasan
Journal of structural design and construction practice. (2024) Vol. 30, Iss. 2
Closed Access | Times Cited: 2
Predicting tensile strength of steel fiber-reinforced concrete based on a novel differential evolution-optimized extreme gradient boosting machine
NhatâDuc Hoang
Neural Computing and Applications (2024)
Closed Access | Times Cited: 1
NhatâDuc Hoang
Neural Computing and Applications (2024)
Closed Access | Times Cited: 1
Soft Computing Techniques to Model the Compressive Strength in Geo-Polymer Concrete: Approaches Based on an Adaptive Neuro-Fuzzy Inference System
Zhiguo Chang, Xuyang Shi, Kaidan Zheng, et al.
Buildings (2024) Vol. 14, Iss. 11, pp. 3505-3505
Open Access | Times Cited: 1
Zhiguo Chang, Xuyang Shi, Kaidan Zheng, et al.
Buildings (2024) Vol. 14, Iss. 11, pp. 3505-3505
Open Access | Times Cited: 1
Traffic noise prediction model using GIS and ensemble machine learning: a case study at Universiti Teknologi Malaysia (UTM) Campus
Khaled Yousef Almansi, Uznir Ujang, Suhaibah Azri, et al.
Environmental Science and Pollution Research (2024) Vol. 31, Iss. 51, pp. 60905-60926
Closed Access | Times Cited: 1
Khaled Yousef Almansi, Uznir Ujang, Suhaibah Azri, et al.
Environmental Science and Pollution Research (2024) Vol. 31, Iss. 51, pp. 60905-60926
Closed Access | Times Cited: 1
Investigation of nano-basic oxygen furnace slag and nano-banded iron formation on properties of high-performance geopolymer concrete
Ali H. AlAteah
REVIEWS ON ADVANCED MATERIALS SCIENCE (2024) Vol. 63, Iss. 1
Open Access
Ali H. AlAteah
REVIEWS ON ADVANCED MATERIALS SCIENCE (2024) Vol. 63, Iss. 1
Open Access