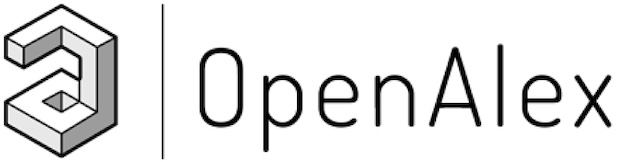
OpenAlex is a bibliographic catalogue of scientific papers, authors and institutions accessible in open access mode, named after the Library of Alexandria. It's citation coverage is excellent and I hope you will find utility in this listing of citing articles!
If you click the article title, you'll navigate to the article, as listed in CrossRef. If you click the Open Access links, you'll navigate to the "best Open Access location". Clicking the citation count will open this listing for that article. Lastly at the bottom of the page, you'll find basic pagination options.
Requested Article:
X-FACTR: Multilingual Factual Knowledge Retrieval from Pretrained Language Models
Zhengbao Jiang, Antonios Anastasopoulos, Jun Araki, et al.
(2020)
Open Access | Times Cited: 83
Zhengbao Jiang, Antonios Anastasopoulos, Jun Araki, et al.
(2020)
Open Access | Times Cited: 83
Showing 1-25 of 83 citing articles:
Pre-train, Prompt, and Predict: A Systematic Survey of Prompting Methods in Natural Language Processing
Pengfei Liu, Weizhe Yuan, Jinlan Fu, et al.
ACM Computing Surveys (2022) Vol. 55, Iss. 9, pp. 1-35
Open Access | Times Cited: 2113
Pengfei Liu, Weizhe Yuan, Jinlan Fu, et al.
ACM Computing Surveys (2022) Vol. 55, Iss. 9, pp. 1-35
Open Access | Times Cited: 2113
Recent Advances in Natural Language Processing via Large Pre-trained Language Models: A Survey
Bonan Min, Hayley Ross, Elior Sulem, et al.
ACM Computing Surveys (2023) Vol. 56, Iss. 2, pp. 1-40
Open Access | Times Cited: 607
Bonan Min, Hayley Ross, Elior Sulem, et al.
ACM Computing Surveys (2023) Vol. 56, Iss. 2, pp. 1-40
Open Access | Times Cited: 607
GPT understands, too
Xiao Liu, Yanan Zheng, Zhengxiao Du, et al.
AI Open (2023) Vol. 5, pp. 208-215
Open Access | Times Cited: 252
Xiao Liu, Yanan Zheng, Zhengxiao Du, et al.
AI Open (2023) Vol. 5, pp. 208-215
Open Access | Times Cited: 252
AMMU: A survey of transformer-based biomedical pretrained language models
Katikapalli Subramanyam Kalyan, Ajit Rajasekharan, S. Sangeetha
Journal of Biomedical Informatics (2021) Vol. 126, pp. 103982-103982
Open Access | Times Cited: 200
Katikapalli Subramanyam Kalyan, Ajit Rajasekharan, S. Sangeetha
Journal of Biomedical Informatics (2021) Vol. 126, pp. 103982-103982
Open Access | Times Cited: 200
Time-Aware Language Models as Temporal Knowledge Bases
Bhuwan Dhingra, Jeremy R. Cole, Julian Martin Eisenschlos, et al.
Transactions of the Association for Computational Linguistics (2022) Vol. 10, pp. 257-273
Open Access | Times Cited: 94
Bhuwan Dhingra, Jeremy R. Cole, Julian Martin Eisenschlos, et al.
Transactions of the Association for Computational Linguistics (2022) Vol. 10, pp. 257-273
Open Access | Times Cited: 94
Knowledge Neurons in Pretrained Transformers
Damai Dai, Li Dong, Yaru Hao, et al.
Proceedings of the 60th Annual Meeting of the Association for Computational Linguistics (Volume 1: Long Papers) (2022), pp. 8493-8502
Open Access | Times Cited: 80
Damai Dai, Li Dong, Yaru Hao, et al.
Proceedings of the 60th Annual Meeting of the Association for Computational Linguistics (Volume 1: Long Papers) (2022), pp. 8493-8502
Open Access | Times Cited: 80
How Can We Know When Language Models Know? On the Calibration of Language Models for Question Answering
Zhengbao Jiang, Jun Araki, Haibo Ding, et al.
Transactions of the Association for Computational Linguistics (2021) Vol. 9, pp. 962-977
Open Access | Times Cited: 103
Zhengbao Jiang, Jun Araki, Haibo Ding, et al.
Transactions of the Association for Computational Linguistics (2021) Vol. 9, pp. 962-977
Open Access | Times Cited: 103
XTREME-R: Towards More Challenging and Nuanced Multilingual Evaluation
Sebastian Ruder, Noah Constant, Jan A. Botha, et al.
Proceedings of the 2021 Conference on Empirical Methods in Natural Language Processing (2021), pp. 10215-10245
Open Access | Times Cited: 98
Sebastian Ruder, Noah Constant, Jan A. Botha, et al.
Proceedings of the 2021 Conference on Empirical Methods in Natural Language Processing (2021), pp. 10215-10245
Open Access | Times Cited: 98
GPT Understands, Too
Xiao Liu, Yanan Zheng, Zhengxiao Du, et al.
arXiv (Cornell University) (2021)
Closed Access | Times Cited: 79
Xiao Liu, Yanan Zheng, Zhengxiao Du, et al.
arXiv (Cornell University) (2021)
Closed Access | Times Cited: 79
Multilingual LAMA: Investigating Knowledge in Multilingual Pretrained Language Models
Nora Kassner, Philipp Dufter, Hinrich Schütze
(2021), pp. 3250-3258
Open Access | Times Cited: 70
Nora Kassner, Philipp Dufter, Hinrich Schütze
(2021), pp. 3250-3258
Open Access | Times Cited: 70
Improving Biomedical Pretrained Language Models with Knowledge
Zheng Yuan, Yijia Liu, Chuanqi Tan, et al.
(2021)
Open Access | Times Cited: 66
Zheng Yuan, Yijia Liu, Chuanqi Tan, et al.
(2021)
Open Access | Times Cited: 66
Knowledgeable or Educated Guess? Revisiting Language Models as Knowledge Bases
Boxi Cao, Hongyu Lin, Xianpei Han, et al.
(2021)
Open Access | Times Cited: 65
Boxi Cao, Hongyu Lin, Xianpei Han, et al.
(2021)
Open Access | Times Cited: 65
Few-shot Learning with Multilingual Generative Language Models
Xi Victoria Lin, Todor Mihaylov, Mikel Artetxe, et al.
Proceedings of the 2021 Conference on Empirical Methods in Natural Language Processing (2022)
Open Access | Times Cited: 63
Xi Victoria Lin, Todor Mihaylov, Mikel Artetxe, et al.
Proceedings of the 2021 Conference on Empirical Methods in Natural Language Processing (2022)
Open Access | Times Cited: 63
Does BERT Pretrained on Clinical Notes Reveal Sensitive Data?
Eric Lehman, Sarthak Jain, Karl Pichotta, et al.
Proceedings of the 2022 Conference of the North American Chapter of the Association for Computational Linguistics: Human Language Technologies (2021)
Open Access | Times Cited: 62
Eric Lehman, Sarthak Jain, Karl Pichotta, et al.
Proceedings of the 2022 Conference of the North American Chapter of the Association for Computational Linguistics: Human Language Technologies (2021)
Open Access | Times Cited: 62
Efficient Large Scale Language Modeling with Mixtures of Experts
Mikel Artetxe, Shruti Bhosale, Naman Goyal, et al.
Proceedings of the 2021 Conference on Empirical Methods in Natural Language Processing (2022)
Open Access | Times Cited: 47
Mikel Artetxe, Shruti Bhosale, Naman Goyal, et al.
Proceedings of the 2021 Conference on Empirical Methods in Natural Language Processing (2022)
Open Access | Times Cited: 47
Are Large Pre-Trained Language Models Leaking Your Personal Information?
Jie Huang, Hanyin Shao, Kevin Chen–Chuan Chang
(2022)
Open Access | Times Cited: 47
Jie Huang, Hanyin Shao, Kevin Chen–Chuan Chang
(2022)
Open Access | Times Cited: 47
Can Language Models be Biomedical Knowledge Bases?
Mujeen Sung, Jinhyuk Lee, Sean S. Yi, et al.
Proceedings of the 2021 Conference on Empirical Methods in Natural Language Processing (2021)
Open Access | Times Cited: 50
Mujeen Sung, Jinhyuk Lee, Sean S. Yi, et al.
Proceedings of the 2021 Conference on Empirical Methods in Natural Language Processing (2021)
Open Access | Times Cited: 50
Probing Pre-Trained Language Models for Cross-Cultural Differences in Values
Arnav Arora, Lucie-Aimée Kaffee, Isabelle Augenstein
(2023)
Open Access | Times Cited: 22
Arnav Arora, Lucie-Aimée Kaffee, Isabelle Augenstein
(2023)
Open Access | Times Cited: 22
Recent Advances in Natural Language Processing via Large Pre-Trained Language Models: A Survey
Bonan Min, Hayley Ross, Elior Sulem, et al.
arXiv (Cornell University) (2021)
Open Access | Times Cited: 39
Bonan Min, Hayley Ross, Elior Sulem, et al.
arXiv (Cornell University) (2021)
Open Access | Times Cited: 39
Unraveling the Inner Workings of Massive Language Models
C. V. Suresh Babu, C. S. Akkash Anniyappa, Dharma Sastha B.
Advances in computational intelligence and robotics book series (2024), pp. 239-279
Closed Access | Times Cited: 6
C. V. Suresh Babu, C. S. Akkash Anniyappa, Dharma Sastha B.
Advances in computational intelligence and robotics book series (2024), pp. 239-279
Closed Access | Times Cited: 6
Relational World Knowledge Representation in Contextual Language Models: A Review
Tara Safavi, Danai Koutra
Proceedings of the 2021 Conference on Empirical Methods in Natural Language Processing (2021)
Open Access | Times Cited: 28
Tara Safavi, Danai Koutra
Proceedings of the 2021 Conference on Empirical Methods in Natural Language Processing (2021)
Open Access | Times Cited: 28
GeoMLAMA: Geo-Diverse Commonsense Probing on Multilingual Pre-Trained Language Models
Da Yin, Hritik Bansal, Masoud Monajatipoor, et al.
Proceedings of the 2021 Conference on Empirical Methods in Natural Language Processing (2022)
Open Access | Times Cited: 20
Da Yin, Hritik Bansal, Masoud Monajatipoor, et al.
Proceedings of the 2021 Conference on Empirical Methods in Natural Language Processing (2022)
Open Access | Times Cited: 20
How Can We Know When Language Models Know? On the Calibration of Language Models for Question Answering
Zhengbao Jiang, Jun Araki, Haibo Ding, et al.
arXiv (Cornell University) (2020)
Open Access | Times Cited: 32
Zhengbao Jiang, Jun Araki, Haibo Ding, et al.
arXiv (Cornell University) (2020)
Open Access | Times Cited: 32
Common Sense Beyond English: Evaluating and Improving Multilingual Language Models for Commonsense Reasoning
Bill Yuchen Lin, Seyeon Lee, Xiaoyang Qiao, et al.
(2021)
Open Access | Times Cited: 27
Bill Yuchen Lin, Seyeon Lee, Xiaoyang Qiao, et al.
(2021)
Open Access | Times Cited: 27
Can Prompt Probe Pretrained Language Models? Understanding the Invisible Risks from a Causal View
Boxi Cao, Hongyu Lin, Xianpei Han, et al.
Proceedings of the 60th Annual Meeting of the Association for Computational Linguistics (Volume 1: Long Papers) (2022), pp. 5796-5808
Open Access | Times Cited: 18
Boxi Cao, Hongyu Lin, Xianpei Han, et al.
Proceedings of the 60th Annual Meeting of the Association for Computational Linguistics (Volume 1: Long Papers) (2022), pp. 5796-5808
Open Access | Times Cited: 18