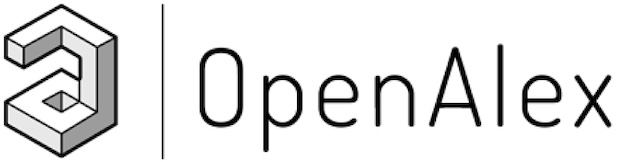
OpenAlex is a bibliographic catalogue of scientific papers, authors and institutions accessible in open access mode, named after the Library of Alexandria. It's citation coverage is excellent and I hope you will find utility in this listing of citing articles!
If you click the article title, you'll navigate to the article, as listed in CrossRef. If you click the Open Access links, you'll navigate to the "best Open Access location". Clicking the citation count will open this listing for that article. Lastly at the bottom of the page, you'll find basic pagination options.
Requested Article:
Can Abnormality be Detected by Graph Neural Networks?
Ziwei Chai, Siqi You, Yang Yang, et al.
Proceedings of the Thirty-First International Joint Conference on Artificial Intelligence (2022), pp. 1945-1951
Open Access | Times Cited: 40
Ziwei Chai, Siqi You, Yang Yang, et al.
Proceedings of the Thirty-First International Joint Conference on Artificial Intelligence (2022), pp. 1945-1951
Open Access | Times Cited: 40
Showing 1-25 of 40 citing articles:
Alleviating Structural Distribution Shift in Graph Anomaly Detection
Yuan Gao, Xiang Wang, Xiangnan He, et al.
(2023)
Open Access | Times Cited: 30
Yuan Gao, Xiang Wang, Xiangnan He, et al.
(2023)
Open Access | Times Cited: 30
DGA-GNN: Dynamic Grouping Aggregation GNN for Fraud Detection
Mingjiang Duan, Tongya Zheng, Yang Gao, et al.
Proceedings of the AAAI Conference on Artificial Intelligence (2024) Vol. 38, Iss. 10, pp. 11820-11828
Open Access | Times Cited: 5
Mingjiang Duan, Tongya Zheng, Yang Gao, et al.
Proceedings of the AAAI Conference on Artificial Intelligence (2024) Vol. 38, Iss. 10, pp. 11820-11828
Open Access | Times Cited: 5
Barely Supervised Learning for Graph-Based Fraud Detection
Hang Yu, Zhengyang Liu, Xiangfeng Luo
Proceedings of the AAAI Conference on Artificial Intelligence (2024) Vol. 38, Iss. 15, pp. 16548-16557
Open Access | Times Cited: 4
Hang Yu, Zhengyang Liu, Xiangfeng Luo
Proceedings of the AAAI Conference on Artificial Intelligence (2024) Vol. 38, Iss. 15, pp. 16548-16557
Open Access | Times Cited: 4
Few-Shot Graph Anomaly Detection via Dual-Level Knowledge Distillation
Xuan Li, Defu Cheng, L H Zhang, et al.
Entropy (2025) Vol. 27, Iss. 1, pp. 28-28
Open Access
Xuan Li, Defu Cheng, L H Zhang, et al.
Entropy (2025) Vol. 27, Iss. 1, pp. 28-28
Open Access
Graph anomaly detection based on hybrid node representation learning
Xiang Wang, Hao Dou, Dibo Dong, et al.
Neural Networks (2025) Vol. 185, pp. 107169-107169
Closed Access
Xiang Wang, Hao Dou, Dibo Dong, et al.
Neural Networks (2025) Vol. 185, pp. 107169-107169
Closed Access
A comprehensive survey on GNN-based anomaly detection: taxonomy, methods, and the role of large language models
Ziqi Yuan, Qingyun Sun, Haoyi Zhou, et al.
International Journal of Machine Learning and Cybernetics (2025)
Closed Access
Ziqi Yuan, Qingyun Sun, Haoyi Zhou, et al.
International Journal of Machine Learning and Cybernetics (2025)
Closed Access
Spectrum-Constrained and Skip-Enhanced Graph Fraud Detection: Addressing Heterophily in Fraud Detection with Spectral and Spatial Modeling
Ijeoma Amuche Chikwendu, Xiaoling Zhang, Chiagoziem C. Ukwuoma, et al.
Symmetry (2025) Vol. 17, Iss. 4, pp. 476-476
Open Access
Ijeoma Amuche Chikwendu, Xiaoling Zhang, Chiagoziem C. Ukwuoma, et al.
Symmetry (2025) Vol. 17, Iss. 4, pp. 476-476
Open Access
How to use Graph Data in the Wild to Help Graph Anomaly Detection?
Yuxuan Cao, Jiarong Xu, Chen Zhao, et al.
(2025), pp. 61-72
Closed Access
Yuxuan Cao, Jiarong Xu, Chen Zhao, et al.
(2025), pp. 61-72
Closed Access
Multi-Granularity Augmented Graph Learning for Spoofing Transaction Detection
Xin Liu, Haojun Rui, Dawei Cheng, et al.
(2025), pp. 5151-5160
Closed Access
Xin Liu, Haojun Rui, Dawei Cheng, et al.
(2025), pp. 5151-5160
Closed Access
Grad: Guided Relation Diffusion Generation for Graph Augmentation in Graph Fraud Detection
Jie Yang, Rui Zhang, Ziyang Cheng, et al.
(2025), pp. 5308-5319
Closed Access
Jie Yang, Rui Zhang, Ziyang Cheng, et al.
(2025), pp. 5308-5319
Closed Access
Mining Mobile Network Fraudsters with Augmented Graph Neural Networks
Xinxin Hu, Haotian Chen, Hongchang Chen, et al.
Entropy (2023) Vol. 25, Iss. 1, pp. 150-150
Open Access | Times Cited: 10
Xinxin Hu, Haotian Chen, Hongchang Chen, et al.
Entropy (2023) Vol. 25, Iss. 1, pp. 150-150
Open Access | Times Cited: 10
Beyond Homophily: Robust Graph Anomaly Detection via Neural Sparsification
Zheng Gong, Guifeng Wang, Ying Sun, et al.
(2023), pp. 2104-2113
Open Access | Times Cited: 7
Zheng Gong, Guifeng Wang, Ying Sun, et al.
(2023), pp. 2104-2113
Open Access | Times Cited: 7
SplitGNN: Spectral Graph Neural Network for Fraud Detection against Heterophily
Bin Wu, Xinyu Yao, Boyan Zhang, et al.
(2023), pp. 2737-2746
Closed Access | Times Cited: 7
Bin Wu, Xinyu Yao, Boyan Zhang, et al.
(2023), pp. 2737-2746
Closed Access | Times Cited: 7
Decoupling Anomaly Discrimination and Representation Learning: Self-supervised Learning for Anomaly Detection on Attributed Graph
Yanming Hu, Chuan Chen, BoWen Deng, et al.
Data Science and Engineering (2024) Vol. 9, Iss. 3, pp. 264-277
Open Access | Times Cited: 2
Yanming Hu, Chuan Chen, BoWen Deng, et al.
Data Science and Engineering (2024) Vol. 9, Iss. 3, pp. 264-277
Open Access | Times Cited: 2
Graph Anomaly Detection with Bi-level Optimization
Yuan Gao, Junfeng Fang, Yongduo Sui, et al.
Proceedings of the ACM Web Conference 2022 (2024), pp. 4383-4394
Closed Access | Times Cited: 2
Yuan Gao, Junfeng Fang, Yongduo Sui, et al.
Proceedings of the ACM Web Conference 2022 (2024), pp. 4383-4394
Closed Access | Times Cited: 2
Fighting against Organized Fraudsters Using Risk Diffusion-based Parallel Graph Neural Network
Jiacheng Ma, Fan Li, Rui Zhang, et al.
(2023), pp. 6138-6146
Open Access | Times Cited: 6
Jiacheng Ma, Fan Li, Rui Zhang, et al.
(2023), pp. 6138-6146
Open Access | Times Cited: 6
Collaborative Fraud Detection: How Collaboration Impacts Fraud Detection
Jinzhang Hu, Ruimin Hu, Zheng Wang, et al.
(2023), pp. 8891-8899
Closed Access | Times Cited: 6
Jinzhang Hu, Ruimin Hu, Zheng Wang, et al.
(2023), pp. 8891-8899
Closed Access | Times Cited: 6
Multiview Graph Contrastive Learning for Multivariate Time-Series Anomaly Detection in IoT
Shuxin Qin, Lin Chen, Yongcan Luo, et al.
IEEE Internet of Things Journal (2023) Vol. 10, Iss. 24, pp. 22401-22414
Closed Access | Times Cited: 5
Shuxin Qin, Lin Chen, Yongcan Luo, et al.
IEEE Internet of Things Journal (2023) Vol. 10, Iss. 24, pp. 22401-22414
Closed Access | Times Cited: 5
Real-Time Anomaly Detection in Network Traffic Using Graph Neural Networks and Random Forest
Waseem Hassan, Seyed Ebrahim Hosseini, Shahbaz Pervez
Lecture notes in computer science (2024), pp. 194-207
Closed Access | Times Cited: 1
Waseem Hassan, Seyed Ebrahim Hosseini, Shahbaz Pervez
Lecture notes in computer science (2024), pp. 194-207
Closed Access | Times Cited: 1
Do not ignore heterogeneity and heterophily: Multi-network collaborative telecom fraud detection
Lingfei Ren, Yilong Zang, Ruimin Hu, et al.
Expert Systems with Applications (2024) Vol. 257, pp. 124974-124974
Closed Access | Times Cited: 1
Lingfei Ren, Yilong Zang, Ruimin Hu, et al.
Expert Systems with Applications (2024) Vol. 257, pp. 124974-124974
Closed Access | Times Cited: 1
Graph Anomaly Detection with Few Labels: A Data-Centric Approach
Xiaoxiao Ma, Ruikun Li, Fanzhen Liu, et al.
Proceedings of the 28th ACM SIGKDD Conference on Knowledge Discovery and Data Mining (2024), pp. 2153-2164
Open Access | Times Cited: 1
Xiaoxiao Ma, Ruikun Li, Fanzhen Liu, et al.
Proceedings of the 28th ACM SIGKDD Conference on Knowledge Discovery and Data Mining (2024), pp. 2153-2164
Open Access | Times Cited: 1
Class Label-aware Graph Anomaly Detection
Junghoon Kim, Yeonjun In, Kanghoon Yoon, et al.
(2023)
Open Access | Times Cited: 3
Junghoon Kim, Yeonjun In, Kanghoon Yoon, et al.
(2023)
Open Access | Times Cited: 3
Where to Find Fascinating Inter-Graph Supervision: Imbalanced Graph Classification with Kernel Information Bottleneck
Hui Tang, Xun Liang
(2023), pp. 3240-3249
Closed Access | Times Cited: 3
Hui Tang, Xun Liang
(2023), pp. 3240-3249
Closed Access | Times Cited: 3
Evolve Path Tracer: Early Detection of Malicious Addresses in Cryptocurrency
Ling Cheng, Feida Zhu, Yong Wang, et al.
Proceedings of the 28th ACM SIGKDD Conference on Knowledge Discovery and Data Mining (2023), pp. 3889-3900
Open Access | Times Cited: 2
Ling Cheng, Feida Zhu, Yong Wang, et al.
Proceedings of the 28th ACM SIGKDD Conference on Knowledge Discovery and Data Mining (2023), pp. 3889-3900
Open Access | Times Cited: 2
Dynamic Relation-Attentive Graph Neural Networks for Fraud Detection
Heehyeon Kim, Jinhyeok Choi, Joyce Jiyoung Whang
2022 IEEE International Conference on Data Mining Workshops (ICDMW) (2023)
Open Access | Times Cited: 2
Heehyeon Kim, Jinhyeok Choi, Joyce Jiyoung Whang
2022 IEEE International Conference on Data Mining Workshops (ICDMW) (2023)
Open Access | Times Cited: 2