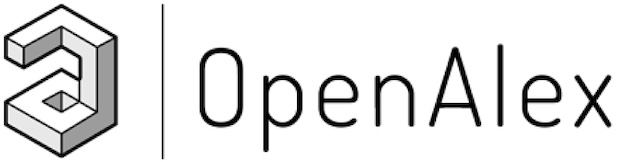
OpenAlex is a bibliographic catalogue of scientific papers, authors and institutions accessible in open access mode, named after the Library of Alexandria. It's citation coverage is excellent and I hope you will find utility in this listing of citing articles!
If you click the article title, you'll navigate to the article, as listed in CrossRef. If you click the Open Access links, you'll navigate to the "best Open Access location". Clicking the citation count will open this listing for that article. Lastly at the bottom of the page, you'll find basic pagination options.
Requested Article:
Application of Machine Learning Approaches to Predict the Strength Property of Geopolymer Concrete
Rongchuan Cao, Zheng Fang, Man Jin, et al.
Materials (2022) Vol. 15, Iss. 7, pp. 2400-2400
Open Access | Times Cited: 43
Rongchuan Cao, Zheng Fang, Man Jin, et al.
Materials (2022) Vol. 15, Iss. 7, pp. 2400-2400
Open Access | Times Cited: 43
Showing 1-25 of 43 citing articles:
A critical review on modeling and prediction on properties of fresh and hardened geopolymer composites
Peng Zhang, Yifan Mao, Weisuo Yuan, et al.
Journal of Building Engineering (2024) Vol. 88, pp. 109184-109184
Closed Access | Times Cited: 23
Peng Zhang, Yifan Mao, Weisuo Yuan, et al.
Journal of Building Engineering (2024) Vol. 88, pp. 109184-109184
Closed Access | Times Cited: 23
Eco-friendly mix design of slag-ash-based geopolymer concrete using explainable deep learning
R.S.S. Ranasinghe, W.K.V.J.B. Kulasooriya, Udara Sachinthana Perera, et al.
Results in Engineering (2024) Vol. 23, pp. 102503-102503
Open Access | Times Cited: 22
R.S.S. Ranasinghe, W.K.V.J.B. Kulasooriya, Udara Sachinthana Perera, et al.
Results in Engineering (2024) Vol. 23, pp. 102503-102503
Open Access | Times Cited: 22
Prediction of compressive and flexural strength of coal gangue-based geopolymer using machine learning method
Yuxuan Zeng, Yanbing Chen, Yizhou Liu, et al.
Materials Today Communications (2025) Vol. 44, pp. 112076-112076
Closed Access | Times Cited: 5
Yuxuan Zeng, Yanbing Chen, Yizhou Liu, et al.
Materials Today Communications (2025) Vol. 44, pp. 112076-112076
Closed Access | Times Cited: 5
Geopolymer: A Systematic Review of Methodologies
Jabulani Matsimbe, Megersa Olumana Dinka, David O. Olukanni, et al.
Materials (2022) Vol. 15, Iss. 19, pp. 6852-6852
Open Access | Times Cited: 67
Jabulani Matsimbe, Megersa Olumana Dinka, David O. Olukanni, et al.
Materials (2022) Vol. 15, Iss. 19, pp. 6852-6852
Open Access | Times Cited: 67
Compressive strength prediction of one-part alkali activated material enabled by interpretable machine learning
Syed Farasat Ali Shah, Bing Chen, Muhammad Zahid, et al.
Construction and Building Materials (2022) Vol. 360, pp. 129534-129534
Closed Access | Times Cited: 51
Syed Farasat Ali Shah, Bing Chen, Muhammad Zahid, et al.
Construction and Building Materials (2022) Vol. 360, pp. 129534-129534
Closed Access | Times Cited: 51
Critical review on the application of artificial intelligence techniques in the production of geopolymer-concrete
George Uwadiegwu Alaneme, Kolawole Adisa Olonade, Ebenezer Esenogho
SN Applied Sciences (2023) Vol. 5, Iss. 8
Open Access | Times Cited: 25
George Uwadiegwu Alaneme, Kolawole Adisa Olonade, Ebenezer Esenogho
SN Applied Sciences (2023) Vol. 5, Iss. 8
Open Access | Times Cited: 25
Estimation of compressive strength of waste concrete utilizing fly ash/slag in concrete with interpretable approaches: optimization and graphical user interface (GUI)
Yakubu Aminu Dodo, Kiran Arif, Mana Alyami, et al.
Scientific Reports (2024) Vol. 14, Iss. 1
Open Access | Times Cited: 15
Yakubu Aminu Dodo, Kiran Arif, Mana Alyami, et al.
Scientific Reports (2024) Vol. 14, Iss. 1
Open Access | Times Cited: 15
A comparative analysis of tree-based machine learning algorithms for predicting the mechanical properties of fibre-reinforced GGBS geopolymer concrete
Shimol Philip, M. Nidhi, Hemn Unis Ahmed
Multiscale and Multidisciplinary Modeling Experiments and Design (2024) Vol. 7, Iss. 3, pp. 2555-2583
Closed Access | Times Cited: 13
Shimol Philip, M. Nidhi, Hemn Unis Ahmed
Multiscale and Multidisciplinary Modeling Experiments and Design (2024) Vol. 7, Iss. 3, pp. 2555-2583
Closed Access | Times Cited: 13
A systematic literature review of AI-based prediction methods for self-compacting, geopolymer, and other eco-friendly concrete types: Advancing sustainable concrete
Tariq Ali, Mohamed Hechmi El Ouni, Muhammad Zeeshan Qureshi, et al.
Construction and Building Materials (2024) Vol. 440, pp. 137370-137370
Closed Access | Times Cited: 11
Tariq Ali, Mohamed Hechmi El Ouni, Muhammad Zeeshan Qureshi, et al.
Construction and Building Materials (2024) Vol. 440, pp. 137370-137370
Closed Access | Times Cited: 11
State-of-the-art review of geopolymer concrete carbonation: From impact analysis to model establishment
Cheng Zhao, Ziqing Li, Shuangdi Peng, et al.
Case Studies in Construction Materials (2024) Vol. 20, pp. e03124-e03124
Open Access | Times Cited: 9
Cheng Zhao, Ziqing Li, Shuangdi Peng, et al.
Case Studies in Construction Materials (2024) Vol. 20, pp. e03124-e03124
Open Access | Times Cited: 9
Artificial intelligence prediction of the mechanical properties of banana peel-ash and bagasse blended geopolymer concrete
George Uwadiegwu Alaneme, Kolawole Adisa Olonade, Ebenezer Esenogho, et al.
Scientific Reports (2024) Vol. 14, Iss. 1
Open Access | Times Cited: 9
George Uwadiegwu Alaneme, Kolawole Adisa Olonade, Ebenezer Esenogho, et al.
Scientific Reports (2024) Vol. 14, Iss. 1
Open Access | Times Cited: 9
Exploratory literature review and scientometric analysis of artificial intelligence applied to geopolymeric materials
Aldo Ribeiro de Carvalho, Romário Parreira Pita, Thaís Mayra de Oliveira, et al.
Engineering Applications of Artificial Intelligence (2025) Vol. 145, pp. 110210-110210
Closed Access | Times Cited: 1
Aldo Ribeiro de Carvalho, Romário Parreira Pita, Thaís Mayra de Oliveira, et al.
Engineering Applications of Artificial Intelligence (2025) Vol. 145, pp. 110210-110210
Closed Access | Times Cited: 1
Development and optimization of geopolymer concrete with compressive strength prediction using particle swarm-optimized extreme gradient boosting
Shimol Philip, Nidhi Marakkath
Applied Soft Computing (2025), pp. 113149-113149
Closed Access | Times Cited: 1
Shimol Philip, Nidhi Marakkath
Applied Soft Computing (2025), pp. 113149-113149
Closed Access | Times Cited: 1
Assessment of Artificial Intelligence Strategies to Estimate the Strength of Geopolymer Composites and Influence of Input Parameters
Kaffayatullah Khan, Waqas Ahmad, Muhammad Nasir Amin, et al.
Polymers (2022) Vol. 14, Iss. 12, pp. 2509-2509
Open Access | Times Cited: 34
Kaffayatullah Khan, Waqas Ahmad, Muhammad Nasir Amin, et al.
Polymers (2022) Vol. 14, Iss. 12, pp. 2509-2509
Open Access | Times Cited: 34
Prediction of compressive strength of alkali-activated construction demolition waste geopolymers using ensemble machine learning
Jiale Shen, Yue Li, Hui Lin, et al.
Construction and Building Materials (2022) Vol. 360, pp. 129600-129600
Closed Access | Times Cited: 32
Jiale Shen, Yue Li, Hui Lin, et al.
Construction and Building Materials (2022) Vol. 360, pp. 129600-129600
Closed Access | Times Cited: 32
Artificial intelligence techniques in advanced concrete technology: A comprehensive survey on 10 years research trend
Ramin Kazemi
Engineering Reports (2023) Vol. 5, Iss. 9
Open Access | Times Cited: 22
Ramin Kazemi
Engineering Reports (2023) Vol. 5, Iss. 9
Open Access | Times Cited: 22
Behavior of hematite, magnetite, and reduced iron powder in geopolymers: Effects of mechanical properties and reaction mechanism
Xian Wu, Xian Zhou, Chao Guo, et al.
Journal of Cleaner Production (2024) Vol. 444, pp. 141178-141178
Closed Access | Times Cited: 9
Xian Wu, Xian Zhou, Chao Guo, et al.
Journal of Cleaner Production (2024) Vol. 444, pp. 141178-141178
Closed Access | Times Cited: 9
Machine learning-driven innovations in green eco-environmental rubberized concrete design towards sustainability
Haï-Bang Ly, Thuy‐Anh Nguyen
Materials Today Communications (2024) Vol. 39, pp. 108551-108551
Closed Access | Times Cited: 8
Haï-Bang Ly, Thuy‐Anh Nguyen
Materials Today Communications (2024) Vol. 39, pp. 108551-108551
Closed Access | Times Cited: 8
Machine learning guided iterative mix design of geopolymer concrete
Haodong Ji, Yuhui Lyu, Weichao Ying, et al.
Journal of Building Engineering (2024) Vol. 91, pp. 109710-109710
Closed Access | Times Cited: 7
Haodong Ji, Yuhui Lyu, Weichao Ying, et al.
Journal of Building Engineering (2024) Vol. 91, pp. 109710-109710
Closed Access | Times Cited: 7
A Systematic Review of the Research Development on the Application of Machine Learning for Concrete
Kaffayatullah Khan, Waqas Ahmad, Muhammad Nasir Amin, et al.
Materials (2022) Vol. 15, Iss. 13, pp. 4512-4512
Open Access | Times Cited: 26
Kaffayatullah Khan, Waqas Ahmad, Muhammad Nasir Amin, et al.
Materials (2022) Vol. 15, Iss. 13, pp. 4512-4512
Open Access | Times Cited: 26
Experimental investigation and comparative machine learning prediction of the compressive strength of recycled aggregate concrete incorporated with fly ash, GGBS, and metakaolin
Uma Shankar Biswal, Mayank Mishra, Manav Kumar Singh, et al.
Innovative Infrastructure Solutions (2022) Vol. 7, Iss. 4
Closed Access | Times Cited: 26
Uma Shankar Biswal, Mayank Mishra, Manav Kumar Singh, et al.
Innovative Infrastructure Solutions (2022) Vol. 7, Iss. 4
Closed Access | Times Cited: 26
Development of a Machine Learning (ML)-Based Computational Model to Estimate the Engineering Properties of Portland Cement Concrete (PCC)
Rodrigo Polo-Mendoza, Gilberto Martínez-Arguelles, Rita Peñabaena‐Niebles, et al.
Arabian Journal for Science and Engineering (2024) Vol. 49, Iss. 10, pp. 14351-14365
Open Access | Times Cited: 5
Rodrigo Polo-Mendoza, Gilberto Martínez-Arguelles, Rita Peñabaena‐Niebles, et al.
Arabian Journal for Science and Engineering (2024) Vol. 49, Iss. 10, pp. 14351-14365
Open Access | Times Cited: 5
A novel framework for strength prediction of geopolymer mortar: Renovative precursor effect
Zafer Kurt, Yıldıran Yılmaz, Talip Çakmak, et al.
Journal of Building Engineering (2023) Vol. 76, pp. 107041-107041
Closed Access | Times Cited: 12
Zafer Kurt, Yıldıran Yılmaz, Talip Çakmak, et al.
Journal of Building Engineering (2023) Vol. 76, pp. 107041-107041
Closed Access | Times Cited: 12
Data-driven approaches for strength prediction of alkali-activated composites
Mohammed Awad Abuhussain, Ayaz Ahmad, Muhammad Nasir Amin, et al.
Case Studies in Construction Materials (2024) Vol. 20, pp. e02920-e02920
Open Access | Times Cited: 4
Mohammed Awad Abuhussain, Ayaz Ahmad, Muhammad Nasir Amin, et al.
Case Studies in Construction Materials (2024) Vol. 20, pp. e02920-e02920
Open Access | Times Cited: 4
Compressive strength prediction of hydrothermally solidified clay with different machine learning techniques
Minguo Lin, Ruobin Su, Geng Chen, et al.
Journal of Cleaner Production (2023) Vol. 413, pp. 137541-137541
Closed Access | Times Cited: 11
Minguo Lin, Ruobin Su, Geng Chen, et al.
Journal of Cleaner Production (2023) Vol. 413, pp. 137541-137541
Closed Access | Times Cited: 11