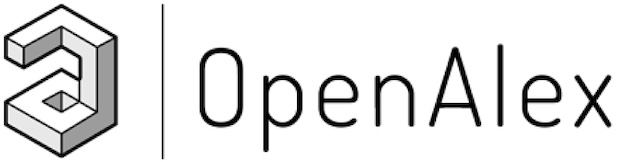
OpenAlex is a bibliographic catalogue of scientific papers, authors and institutions accessible in open access mode, named after the Library of Alexandria. It's citation coverage is excellent and I hope you will find utility in this listing of citing articles!
If you click the article title, you'll navigate to the article, as listed in CrossRef. If you click the Open Access links, you'll navigate to the "best Open Access location". Clicking the citation count will open this listing for that article. Lastly at the bottom of the page, you'll find basic pagination options.
Requested Article:
Intelligent Design of Construction Materials: A Comparative Study of AI Approaches for Predicting the Strength of Concrete with Blast Furnace Slag
Xiangping Wu, Fei Zhu, Mengmeng Zhou, et al.
Materials (2022) Vol. 15, Iss. 13, pp. 4582-4582
Open Access | Times Cited: 19
Xiangping Wu, Fei Zhu, Mengmeng Zhou, et al.
Materials (2022) Vol. 15, Iss. 13, pp. 4582-4582
Open Access | Times Cited: 19
Showing 19 citing articles:
Efficient machine learning method for evaluating compressive strength of cement stabilized soft soil
Chen Zhang, Zhiduo Zhu, Fa Liu, et al.
Construction and Building Materials (2023) Vol. 392, pp. 131887-131887
Closed Access | Times Cited: 47
Chen Zhang, Zhiduo Zhu, Fa Liu, et al.
Construction and Building Materials (2023) Vol. 392, pp. 131887-131887
Closed Access | Times Cited: 47
Artificial Neural Networks for Sustainable Development of the Construction Industry
Mohd. Ahmed, Saeed Alqadhi, Javed Mallick, et al.
Sustainability (2022) Vol. 14, Iss. 22, pp. 14738-14738
Open Access | Times Cited: 35
Mohd. Ahmed, Saeed Alqadhi, Javed Mallick, et al.
Sustainability (2022) Vol. 14, Iss. 22, pp. 14738-14738
Open Access | Times Cited: 35
Comparative analysis of various machine learning algorithms to predict 28-day compressive strength of Self-compacting concrete
Waleed Bin Inqiad, Muhammad Shahid Siddique, Saad S. Alarifi, et al.
Heliyon (2023) Vol. 9, Iss. 11, pp. e22036-e22036
Open Access | Times Cited: 19
Waleed Bin Inqiad, Muhammad Shahid Siddique, Saad S. Alarifi, et al.
Heliyon (2023) Vol. 9, Iss. 11, pp. e22036-e22036
Open Access | Times Cited: 19
Supplementary cementitious materials-based concrete porosity estimation using modeling approaches: A comparative study of GEP and MEP
Qiong Tian, Yijun Lü, Ji Zhou, et al.
REVIEWS ON ADVANCED MATERIALS SCIENCE (2024) Vol. 63, Iss. 1
Closed Access | Times Cited: 8
Qiong Tian, Yijun Lü, Ji Zhou, et al.
REVIEWS ON ADVANCED MATERIALS SCIENCE (2024) Vol. 63, Iss. 1
Closed Access | Times Cited: 8
Efficient machine learning for strength prediction of ready-mix concrete production (prolonged mixing)
Wiput Tuvayanond, Viroon Kamchoom, Lapyote Prasittisopin
Construction Innovation (2024)
Closed Access | Times Cited: 7
Wiput Tuvayanond, Viroon Kamchoom, Lapyote Prasittisopin
Construction Innovation (2024)
Closed Access | Times Cited: 7
Understanding Penetration Attenuation of Permeable Concrete: A Hybrid Artificial Intelligence Technique Based on Particle Swarm Optimization
Fei Zhu, Xiangping Wu, Yijun Lü, et al.
Buildings (2024) Vol. 14, Iss. 4, pp. 1173-1173
Open Access | Times Cited: 6
Fei Zhu, Xiangping Wu, Yijun Lü, et al.
Buildings (2024) Vol. 14, Iss. 4, pp. 1173-1173
Open Access | Times Cited: 6
Compressive strength of waste-derived cementitious composites using machine learning
Qiong Tian, Yijun Lü, Ji Zhou, et al.
REVIEWS ON ADVANCED MATERIALS SCIENCE (2024) Vol. 63, Iss. 1
Open Access | Times Cited: 6
Qiong Tian, Yijun Lü, Ji Zhou, et al.
REVIEWS ON ADVANCED MATERIALS SCIENCE (2024) Vol. 63, Iss. 1
Open Access | Times Cited: 6
Machine Learning in Solid‐State Hydrogen Storage Materials: Challenges and Perspectives
Panpan Zhou, Qianwen Zhou, Xuezhang Xiao, et al.
Advanced Materials (2024)
Closed Access | Times Cited: 6
Panpan Zhou, Qianwen Zhou, Xuezhang Xiao, et al.
Advanced Materials (2024)
Closed Access | Times Cited: 6
An Explainable Machine Learning (XML) approach to determine strength of glass powder concrete
Wali Ullah Khan, Waleed Bin Inqiad, Bilal Ayub, et al.
Materials Today Communications (2025), pp. 112181-112181
Closed Access
Wali Ullah Khan, Waleed Bin Inqiad, Bilal Ayub, et al.
Materials Today Communications (2025), pp. 112181-112181
Closed Access
Intelligent prediction of compressive strength of self-compacting concrete incorporating silica fume using hybrid IWOA-GPR model
Yang Yu, Guangyin Wang, Ghasan Fahim Huseien, et al.
Materials Today Communications (2025), pp. 112282-112282
Closed Access
Yang Yu, Guangyin Wang, Ghasan Fahim Huseien, et al.
Materials Today Communications (2025), pp. 112282-112282
Closed Access
A Machine Learning Approach to Prediction of the Compressive Strength of Segregated Lightweight Aggregate Concretes Using Ultrasonic Pulse Velocity
Violeta Migallón, Héctor Penadés, Jose Penadés, et al.
Applied Sciences (2023) Vol. 13, Iss. 3, pp. 1953-1953
Open Access | Times Cited: 9
Violeta Migallón, Héctor Penadés, Jose Penadés, et al.
Applied Sciences (2023) Vol. 13, Iss. 3, pp. 1953-1953
Open Access | Times Cited: 9
Runtime-based metaheuristic prediction of the compressive strength of net-zero traditional concrete mixed with BFS, FA, SP considering multiple curing regimes
Kennedy C. Onyelowe, Denise‐Penelope N. Kontoni, Sita Rama Murty Pilla, et al.
Asian Journal of Civil Engineering (2023) Vol. 25, Iss. 2, pp. 1241-1253
Closed Access | Times Cited: 9
Kennedy C. Onyelowe, Denise‐Penelope N. Kontoni, Sita Rama Murty Pilla, et al.
Asian Journal of Civil Engineering (2023) Vol. 25, Iss. 2, pp. 1241-1253
Closed Access | Times Cited: 9
Hybrid Machine Learning Model Based on GWO and PSO Optimization for Prediction of Oilwell Cement Compressive Strength under Acidic Corrosion
Li Wang, Sheng Huang, Zaoyuan Li, et al.
SPE Journal (2024) Vol. 29, Iss. 09, pp. 4684-4695
Closed Access | Times Cited: 2
Li Wang, Sheng Huang, Zaoyuan Li, et al.
SPE Journal (2024) Vol. 29, Iss. 09, pp. 4684-4695
Closed Access | Times Cited: 2
A Novel MBAS-RF Approach to Predict Mechanical Properties of Geopolymer-Based Compositions
Shuzhao Chen, Mengmeng Zhou, Xuyang Shi, et al.
Gels (2023) Vol. 9, Iss. 6, pp. 434-434
Open Access | Times Cited: 5
Shuzhao Chen, Mengmeng Zhou, Xuyang Shi, et al.
Gels (2023) Vol. 9, Iss. 6, pp. 434-434
Open Access | Times Cited: 5
A Comparative Study of AI-Based International Roughness Index (IRI) Prediction Models for Jointed Plain Concrete Pavement (JPCP)
Qiang Wang, Mengmeng Zhou, Mohanad Muayad Sabri Sabri, et al.
Materials (2022) Vol. 15, Iss. 16, pp. 5605-5605
Open Access | Times Cited: 7
Qiang Wang, Mengmeng Zhou, Mohanad Muayad Sabri Sabri, et al.
Materials (2022) Vol. 15, Iss. 16, pp. 5605-5605
Open Access | Times Cited: 7
Preparation of Green Sustainable Cement Paste Mixture Based on Inorganic Additives: An Experimental and Modelling Approach
Aamir Mahmood, Miroslava Pechočiaková, Muhammad Tayyab Noman, et al.
Buildings (2024) Vol. 14, Iss. 7, pp. 1922-1922
Open Access | Times Cited: 1
Aamir Mahmood, Miroslava Pechočiaková, Muhammad Tayyab Noman, et al.
Buildings (2024) Vol. 14, Iss. 7, pp. 1922-1922
Open Access | Times Cited: 1
A Study on the Application of Machine Learning for Predicting Concrete Compressive Strength
Wanshun Jiang, Xiao Bing-gang, Yipeng Wang, et al.
(2023)
Open Access | Times Cited: 1
Wanshun Jiang, Xiao Bing-gang, Yipeng Wang, et al.
(2023)
Open Access | Times Cited: 1
Comparative study on machine learning algorithms for predicting compressive strength of high-strength concrete
Jiaxing Zhou, Jiajian Lin, Yang Gao, et al.
(2024), pp. 786-790
Closed Access
Jiaxing Zhou, Jiajian Lin, Yang Gao, et al.
(2024), pp. 786-790
Closed Access
Design and Implementation of Concrete Compressive Strength Data Cleaning Based on PCA-RANSAC Algorithm
Wanshun Jiang, Xiao Bing-gang, Yipeng Wang, et al.
(2023)
Open Access
Wanshun Jiang, Xiao Bing-gang, Yipeng Wang, et al.
(2023)
Open Access