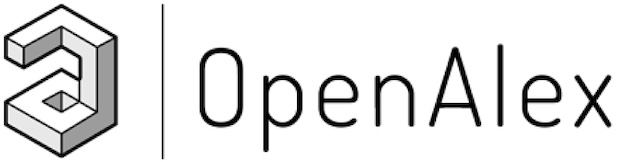
OpenAlex is a bibliographic catalogue of scientific papers, authors and institutions accessible in open access mode, named after the Library of Alexandria. It's citation coverage is excellent and I hope you will find utility in this listing of citing articles!
If you click the article title, you'll navigate to the article, as listed in CrossRef. If you click the Open Access links, you'll navigate to the "best Open Access location". Clicking the citation count will open this listing for that article. Lastly at the bottom of the page, you'll find basic pagination options.
Requested Article:
An Evaluation of Eight Machine Learning Regression Algorithms for Forest Aboveground Biomass Estimation from Multiple Satellite Data Products
Yuzhen Zhang, Jun Ma, Shunlin Liang, et al.
Remote Sensing (2020) Vol. 12, Iss. 24, pp. 4015-4015
Open Access | Times Cited: 107
Yuzhen Zhang, Jun Ma, Shunlin Liang, et al.
Remote Sensing (2020) Vol. 12, Iss. 24, pp. 4015-4015
Open Access | Times Cited: 107
Showing 1-25 of 107 citing articles:
A Review of Ensemble Learning Algorithms Used in Remote Sensing Applications
Yuzhen Zhang, Jingjing Liu, Wenjuan Shen
Applied Sciences (2022) Vol. 12, Iss. 17, pp. 8654-8654
Open Access | Times Cited: 183
Yuzhen Zhang, Jingjing Liu, Wenjuan Shen
Applied Sciences (2022) Vol. 12, Iss. 17, pp. 8654-8654
Open Access | Times Cited: 183
Combination of Feature Selection and CatBoost for Prediction: The First Application to the Estimation of Aboveground Biomass
Mi Luo, Yifu Wang, Yunhong Xie, et al.
Forests (2021) Vol. 12, Iss. 2, pp. 216-216
Open Access | Times Cited: 155
Mi Luo, Yifu Wang, Yunhong Xie, et al.
Forests (2021) Vol. 12, Iss. 2, pp. 216-216
Open Access | Times Cited: 155
Integration of machine learning and remote sensing for above ground biomass estimation through Landsat-9 and field data in temperate forests of the Himalayan region
Shoaib Ahmad Anees, Kaleem Mehmood, Waseem Razzaq Khan, et al.
Ecological Informatics (2024) Vol. 82, pp. 102732-102732
Open Access | Times Cited: 39
Shoaib Ahmad Anees, Kaleem Mehmood, Waseem Razzaq Khan, et al.
Ecological Informatics (2024) Vol. 82, pp. 102732-102732
Open Access | Times Cited: 39
Improving Forest Above-Ground Biomass Estimation by Integrating Individual Machine Learning Models
Mi Luo, Shoaib Ahmad Anees, Qiuyan Huang, et al.
Forests (2024) Vol. 15, Iss. 6, pp. 975-975
Open Access | Times Cited: 28
Mi Luo, Shoaib Ahmad Anees, Qiuyan Huang, et al.
Forests (2024) Vol. 15, Iss. 6, pp. 975-975
Open Access | Times Cited: 28
Estimation of Aboveground Biomass for Different Forest Types Using Data from Sentinel-1, Sentinel-2, ALOS PALSAR-2, and GEDI
Chu Wang, Wangfei Zhang, Yongjie Ji, et al.
Forests (2024) Vol. 15, Iss. 1, pp. 215-215
Open Access | Times Cited: 19
Chu Wang, Wangfei Zhang, Yongjie Ji, et al.
Forests (2024) Vol. 15, Iss. 1, pp. 215-215
Open Access | Times Cited: 19
Improved Estimation of Aboveground Biomass in Rubber Plantations Using Deep Learning on UAV Multispectral Imagery
Hongjian Tan, Weili Kou, Weiheng Xu, et al.
Drones (2025) Vol. 9, Iss. 1, pp. 32-32
Open Access | Times Cited: 2
Hongjian Tan, Weili Kou, Weiheng Xu, et al.
Drones (2025) Vol. 9, Iss. 1, pp. 32-32
Open Access | Times Cited: 2
A stacking ensemble algorithm for improving the biases of forest aboveground biomass estimations from multiple remotely sensed datasets
Yuzhen Zhang, Jun Ma, Shunlin Liang, et al.
GIScience & Remote Sensing (2022) Vol. 59, Iss. 1, pp. 234-249
Open Access | Times Cited: 54
Yuzhen Zhang, Jun Ma, Shunlin Liang, et al.
GIScience & Remote Sensing (2022) Vol. 59, Iss. 1, pp. 234-249
Open Access | Times Cited: 54
Forest Height Mapping Using Feature Selection and Machine Learning by Integrating Multi-Source Satellite Data in Baoding City, North China
Nan Zhang, Mingjie Chen, Fan Yang, et al.
Remote Sensing (2022) Vol. 14, Iss. 18, pp. 4434-4434
Open Access | Times Cited: 40
Nan Zhang, Mingjie Chen, Fan Yang, et al.
Remote Sensing (2022) Vol. 14, Iss. 18, pp. 4434-4434
Open Access | Times Cited: 40
Estimating Aboveground Carbon Dynamic of China Using Optical and Microwave Remote-Sensing Datasets from 2013 to 2019
Zhongbing Chang, Lei Fan, Jean‐Pierre Wigneron, et al.
Journal of Remote Sensing (2023) Vol. 3
Open Access | Times Cited: 26
Zhongbing Chang, Lei Fan, Jean‐Pierre Wigneron, et al.
Journal of Remote Sensing (2023) Vol. 3
Open Access | Times Cited: 26
Evaluation of machine learning methods and multi-source remote sensing data combinations to construct forest above-ground biomass models
Xingguang Yan, Jing Li, Andrew R. Smith, et al.
International Journal of Digital Earth (2023) Vol. 16, Iss. 2, pp. 4471-4491
Open Access | Times Cited: 26
Xingguang Yan, Jing Li, Andrew R. Smith, et al.
International Journal of Digital Earth (2023) Vol. 16, Iss. 2, pp. 4471-4491
Open Access | Times Cited: 26
A comparative analysis of machine learning techniques for aboveground biomass estimation: A case study of the Western Ghats, India
Kurian Ayushi, Kanda Naveen Babu, Narayanan Ayyappan, et al.
Ecological Informatics (2024) Vol. 80, pp. 102479-102479
Open Access | Times Cited: 15
Kurian Ayushi, Kanda Naveen Babu, Narayanan Ayyappan, et al.
Ecological Informatics (2024) Vol. 80, pp. 102479-102479
Open Access | Times Cited: 15
Evaluating machine learning approaches for aboveground biomass prediction in fragmented high-elevated forests using multi-sensor satellite data
Asim Qadeer, Muhammad Shakir, Li Wang, et al.
Remote Sensing Applications Society and Environment (2024) Vol. 36, pp. 101291-101291
Closed Access | Times Cited: 9
Asim Qadeer, Muhammad Shakir, Li Wang, et al.
Remote Sensing Applications Society and Environment (2024) Vol. 36, pp. 101291-101291
Closed Access | Times Cited: 9
Estimating Forest Growing Stock Volume Using Feature Selection and Advanced Remote Sensing Algorithm
Yabing Zhao, Famiao Guo, Yin Wang, et al.
Remote Sensing Applications Society and Environment (2025), pp. 101458-101458
Closed Access | Times Cited: 1
Yabing Zhao, Famiao Guo, Yin Wang, et al.
Remote Sensing Applications Society and Environment (2025), pp. 101458-101458
Closed Access | Times Cited: 1
Combination of Hyperspectral and Machine Learning to Invert Soil Electrical Conductivity
Pingping Jia, Junhua Zhang, Wei He, et al.
Remote Sensing (2022) Vol. 14, Iss. 11, pp. 2602-2602
Open Access | Times Cited: 35
Pingping Jia, Junhua Zhang, Wei He, et al.
Remote Sensing (2022) Vol. 14, Iss. 11, pp. 2602-2602
Open Access | Times Cited: 35
Estimating Aboveground Biomass of Two Different Forest Types in Myanmar from Sentinel-2 Data with Machine Learning and Geostatistical Algorithms
Phyo Wai, Huiyi Su, Mingshi Li
Remote Sensing (2022) Vol. 14, Iss. 9, pp. 2146-2146
Open Access | Times Cited: 34
Phyo Wai, Huiyi Su, Mingshi Li
Remote Sensing (2022) Vol. 14, Iss. 9, pp. 2146-2146
Open Access | Times Cited: 34
Prediction of Urban Forest Aboveground Carbon Using Machine Learning Based on Landsat 8 and Sentinel-2: A Case Study of Shanghai, China
Huimian Li, Guilian Zhang, Qicheng Zhong, et al.
Remote Sensing (2023) Vol. 15, Iss. 1, pp. 284-284
Open Access | Times Cited: 22
Huimian Li, Guilian Zhang, Qicheng Zhong, et al.
Remote Sensing (2023) Vol. 15, Iss. 1, pp. 284-284
Open Access | Times Cited: 22
Estimating the Aboveground Biomass of Various Forest Types with High Heterogeneity at the Provincial Scale Based on Multi-Source Data
Tian‐Bao Huang, Guanglong Ou, Yong Wu, et al.
Remote Sensing (2023) Vol. 15, Iss. 14, pp. 3550-3550
Open Access | Times Cited: 22
Tian‐Bao Huang, Guanglong Ou, Yong Wu, et al.
Remote Sensing (2023) Vol. 15, Iss. 14, pp. 3550-3550
Open Access | Times Cited: 22
Modelling aboveground biomass of a multistage managed forest through synergistic use of Landsat-OLI, ALOS-2 L-band SAR and GEDI metrics
Hitendra Padalia, Ankit Prakash, Taibanganba Watham
Ecological Informatics (2023) Vol. 77, pp. 102234-102234
Closed Access | Times Cited: 22
Hitendra Padalia, Ankit Prakash, Taibanganba Watham
Ecological Informatics (2023) Vol. 77, pp. 102234-102234
Closed Access | Times Cited: 22
Remote sensing and machine learning applications for aboveground biomass estimation in agroforestry systems: a review
Bhuwan Thapa, Sarah Taylor Lovell, Jeffrey J. Wilson
Agroforestry Systems (2023) Vol. 97, Iss. 6, pp. 1097-1111
Closed Access | Times Cited: 20
Bhuwan Thapa, Sarah Taylor Lovell, Jeffrey J. Wilson
Agroforestry Systems (2023) Vol. 97, Iss. 6, pp. 1097-1111
Closed Access | Times Cited: 20
Performance of machine learning algorithms for multi-step ahead prediction of reference evapotranspiration across various agro-climatic zones and cropping seasons
Nehar Mandal, Kironmala Chanda
Journal of Hydrology (2023) Vol. 620, pp. 129418-129418
Closed Access | Times Cited: 19
Nehar Mandal, Kironmala Chanda
Journal of Hydrology (2023) Vol. 620, pp. 129418-129418
Closed Access | Times Cited: 19
Exploiting Digitalization of Solar PV Plants Using Machine Learning: Digital Twin Concept for Operation
Tolga Yalçin, Pol Paradell, Paschalia Stefanidou-Voziki, et al.
Energies (2023) Vol. 16, Iss. 13, pp. 5044-5044
Open Access | Times Cited: 17
Tolga Yalçin, Pol Paradell, Paschalia Stefanidou-Voziki, et al.
Energies (2023) Vol. 16, Iss. 13, pp. 5044-5044
Open Access | Times Cited: 17
Applying Bayesian Optimization for Machine Learning Models in Predicting the Surface Roughness in Single-Point Diamond Turning Polycarbonate
Van-Hai Nguyen, Tien-Thinh Le, Hoanh-Son Truong, et al.
Mathematical Problems in Engineering (2021) Vol. 2021, pp. 1-16
Open Access | Times Cited: 36
Van-Hai Nguyen, Tien-Thinh Le, Hoanh-Son Truong, et al.
Mathematical Problems in Engineering (2021) Vol. 2021, pp. 1-16
Open Access | Times Cited: 36
Delineating the relative contribution of climate related variables to chlorophyll-a and phytoplankton biomass in lakes using the ERA5-Land climate reanalysis data
Konstantinos Stefanidis, George Varlas, Aikaterini Vourka, et al.
Water Research (2021) Vol. 196, pp. 117053-117053
Closed Access | Times Cited: 35
Konstantinos Stefanidis, George Varlas, Aikaterini Vourka, et al.
Water Research (2021) Vol. 196, pp. 117053-117053
Closed Access | Times Cited: 35
A Proposed Ensemble Feature Selection Method for Estimating Forest Aboveground Biomass from Multiple Satellite Data
Yuzhen Zhang, Jingjing Liu, Wenhao Li, et al.
Remote Sensing (2023) Vol. 15, Iss. 4, pp. 1096-1096
Open Access | Times Cited: 16
Yuzhen Zhang, Jingjing Liu, Wenhao Li, et al.
Remote Sensing (2023) Vol. 15, Iss. 4, pp. 1096-1096
Open Access | Times Cited: 16
Estimating fractional vegetation cover and aboveground biomass for land degradation assessment in eastern Mongolia steppe: combining ground vegetation data and remote sensing
Batnyambuu Dashpurev, Munkhtsetseg Dorj, Thanh Noi Phan, et al.
International Journal of Remote Sensing (2023) Vol. 44, Iss. 2, pp. 452-468
Closed Access | Times Cited: 14
Batnyambuu Dashpurev, Munkhtsetseg Dorj, Thanh Noi Phan, et al.
International Journal of Remote Sensing (2023) Vol. 44, Iss. 2, pp. 452-468
Closed Access | Times Cited: 14